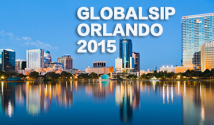
The IEEE Global Conference on Signal and Information Processing (GlobalSIP) is a flagship conference of the IEEE Signal Processing Society. GlobalSIP'15 will be held in Orlando, Florida, USA, December 14-16, 2015. The conference will focus on signal and information processing with an emphasis on up-and-coming signal processing themes. The conference will feature world-class speakers, tutorials, exhibits, and sessions consisting of poster or oral presentations. Outstanding papers will be selected for Best Paper Awards or Best Student Paper Awards; a paper is eligible for a best student paper award if the first author of the paper is a student. IEEE Signal Processing Society and National Science Foundation will provide travel grants to eligible students.
- Read more about Image Transmission over Cognitive Radio Systems with Channel and Sensing Uncertainty
- Log in to post comments
Presentation slides for the 2015 IEEE GlobalSIP paper entitled "Image Transmission over Cognitive Radio Systems with Channel and Sensing Uncertainty" by Chuang Ye, Gozde Ozcan, M. Cenk Gursoy, and Senem Velipasalar.
- Categories:
- Read more about Very-Short Term Forecasting of Electricity Price Signals Using a Pareto Composition of Kernel Machines in Smart Power Systems
- Log in to post comments
Paper presented in "Symposium on Signal and Information Processing for Optimizing Future Energy Systems" that was part of GlobalSip 2015 Conference.
Citation of the paper:
M. Alamaniotis, N. Bourbakis, and L.H. Tsoukalas, “Very-Short Term Forecasting of Electricity Price Signals Using a Pareto Composition of Kernel Machines in Smart Power Systems,” 3rd IEEE Global Conference on Signal and Information Processing, Orlando, FL, December 2015, pp. 1-5.
Authors:
- Categories:
- Categories:

- Read more about Non-Intrusive Load Monitoring: A Power Consumption Based Relaxation
- Log in to post comments
Obtaining per-device energy consumption estimates in Non-Intrusive Load Monitoring (NILM) has proven to be a challenging task. We present Power Consumption Clustered Non-Intrusive Load Monitoring (PCC-NILM), a relaxation of the NILM problem that estimates the energy consumed by devices operating in different power ranges. The Approximate Power Trace Decomposition Algorithm (APTDA) is presented as an unsupervised, data-driven solution to the PCC-NILM problem.
- Categories:
- Read more about Generalized water filling problem
- Log in to post comments
Generalized water filling problem is implemented with the least number of flops (or lease computational complexity) possible.
- Categories:
- Categories:
- Read more about Channel Estimation Using Joint Dictionary Learning in FDD Massive MIMO Systems
- Log in to post comments
In a frequency division duplex (FDD) massive MIMO system, downlink channel estimation poses several challenges with limited training duration being one impediment. Our previous work developed an algorithm to learn a dictionary in which the channel can be sparsely represented, and then leveraged compressed sensing framework to estimate the downlink channel. In this work, we extend the sparse channel representation framework to joint uplink and downlink channel modeling exploiting the similar scattering environment experienced by the signal during uplink and downlink transmission.
- Categories:
- Read more about On Unequal Error Protection Raptor Codes
- Log in to post comments
- Categories:
- Read more about Reconstruction of Graph Signals: Percolation from a Single Seeding Node
- Log in to post comments
Schemes to reconstruct signals defined in the nodes of a graph are proposed. Our focus is on reconstructing bandlimited graph signals, which are signals that admit a sparse representation in a frequency domain related to the structure of the graph. The schemes, which are designed within the framework of linear shift-invariant graph filters, consider that the signal is injected at a single seeding node.
- Categories: