ICASSP is the world's largest and most comprehensive technical conference on signal processing and its applications. It provides a fantastic networking opportunity for like-minded professionals from around the world. ICASSP 2016 conference will feature world-class presentations by internationally renowned speakers and cutting-edge session topics.
- Read more about Supervised Subspace Learning based on Deep Randomized Networks
- Log in to post comments
In this paper, we propose a supervised subspace learning method that exploits the rich representation power of deep feedforward networks. In order to derive a fast, yet efficient, learning scheme we employ deep randomized neural networks that have been recently shown to provide good compromise between training speed and performance.
- Categories:
For ICASSP 2016 paper, "Fast Voxel Line Update for Time-Space Image Reconstruction"
- Categories:
- Read more about COUPLED RANK-(Lm, Ln, ∙) BLOCK TERM DECOMPOSITION BY COUPLED BLOCK SIMULTANEOUS GENERALIZED SCHUR DECOMPOSITION
- Log in to post comments
Coupled decompositions of multiple tensors are fundamental tools for multi-set data fusion. In this paper, we introduce a coupled version of the rank-(Lm, Ln, ·) block term decomposition (BTD), applicable to joint independent
subspace analysis. We propose two algorithms for its computation based on a coupled block simultaneous generalized Schur decomposition scheme. Numerical results are given to show the performance of the proposed algorithms.
- Categories:
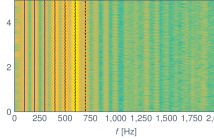
- Read more about Fast and Statistically Efficient Fundamental Frequency Estimation
- Log in to post comments
master.pdf

- Categories:
- Read more about Subspace-based Adaptive Widely Linear Blind Channel Estimation for Constrained Minimum Variance CDMA Receiver
- Log in to post comments
- Categories:
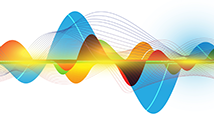
The problem of estimating sparse eigenvectors of a symmetric matrix attracts a lot of attention in many applications, especially those with high dimensional data set. While classical eigenvectors can be obtained as the solution of a maximization
SPCAposter.pdf

- Categories:
- Read more about Dual-microphone voice activity detection based on using optimally weighted maximum a posteriori probability
- Log in to post comments
poster.pdf

- Categories:
- Read more about A NOVEL VIDEO-BASED SMOKE DETECTION METHOD BASED ON COLOR INVARIANTS
- Log in to post comments
In this paper, we address the issue of designing a smoke detector robust to illumination variations. Our contribution consists in resorting to color invariants as salient smoke features. More precisely, the proposed detector employs consecutively of an illumination invariant color representation, a photometric gain based background subtraction, a chrominance detection and a smoke identification based on two invariant color descriptors.
- Categories: