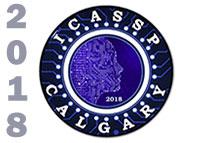
ICASSP is the world’s largest and most comprehensive technical conference focused on signal processing and its applications. The 2019 conference will feature world-class presentations by internationally renowned speakers, cutting-edge session topics and provide a fantastic opportunity to network with like-minded professionals from around the world. Visit website.
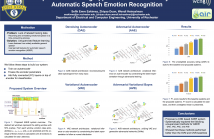
- Read more about UNSUPERVISED LEARNING APPROACH TO FEATURE ANALYSIS FOR AUTOMATIC SPEECH EMOTION RECOGNITION
- Log in to post comments
The scarcity of emotional speech data is a bottleneck of developing automatic speech emotion recognition (ASER) systems. One way to alleviate this issue is to use unsupervised feature learning techniques to learn features from the widely available general speech and use these features to train emotion classifiers. These unsupervised methods, such as denoising autoencoder (DAE), variational autoencoder (VAE), adversarial autoencoder (AAE) and adversarial variational Bayes (AVB), can capture the intrinsic structure of the data distribution in the learned feature representation.
- Categories:
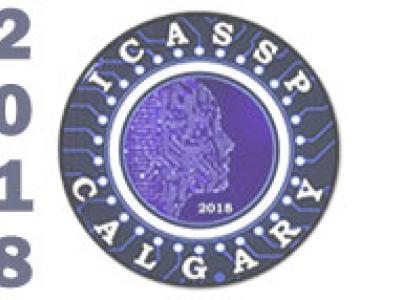
- Read more about Robust Spoken Language Understanding with unsupervised ASR-error adaptation
- Log in to post comments
Robustness to errors produced by automatic speech recognition (ASR) is essential for Spoken Language Understanding (SLU). Traditional robust SLU typically needs ASR hypotheses with semantic annotations for training. However, semantic annotation is very expensive, and the corresponding ASR system may change frequently. Here, we propose a novel unsupervised ASR-error adaptation method, obviating the need of annotated ASR hypotheses.
- Categories:
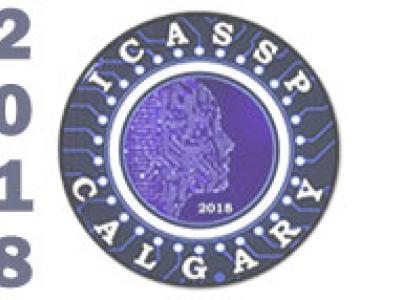
- Read more about MANIFOLD-BASED ANALYSIS OF NATURAL STOCHASTIC TEXTURES WITH APPLICATION IN TEXTURE SYNTHESIS
- Log in to post comments
Embedding textured images in manifolds reveals latent information regarding texture structure and allows useful analysis of these high dimensional images in a low dimensional space. We present a framework for analysis and synthesis of natural stochastic textures (NST) which constitute an important subset of textures that are modelled as realizations of random processes. The randomness of NST differentiates them from other types of images and requires a dedicated method for analysis and synthesis. We demonstrate several applications of this framework.
- Categories:
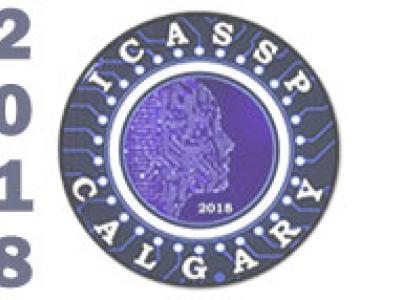
- Read more about EFFICIENT CONVOLUTIONAL DICTIONARY LEARNING USING PARTIAL UPDATE FAST ITERATIVE SHRINKAGE-THRESHOLDING ALGORITHM
- Log in to post comments
Convolutional sparse representations allow modeling an entire image as an alternative to the more common independent patch-based
formulations. Although many approaches have been proposed to efficiently solve the convolutional dictionary learning (CDL) problem,
their computational performance is constrained by the dictionary update stage. In this work, we include two improvements to existing
- Categories:
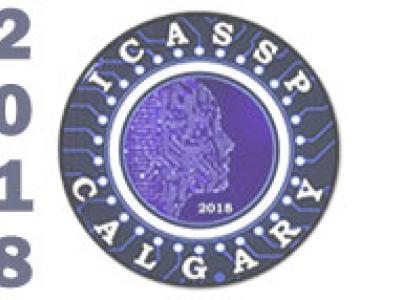
- Read more about Secrecy Capacity Under List Decoding for a Channel With a Passive Eavesdropper and an Active Jammer
- Log in to post comments
- Categories:
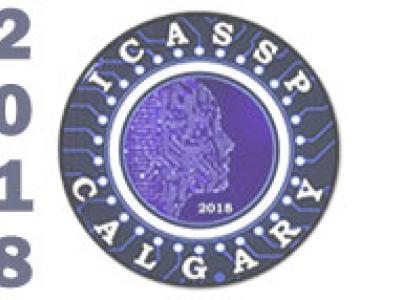
- Read more about Learning-Based Acoustic Source-Microphone Distance Estimation using the Coherent-to-Diffuse Power Ratio
- Log in to post comments
- Categories:
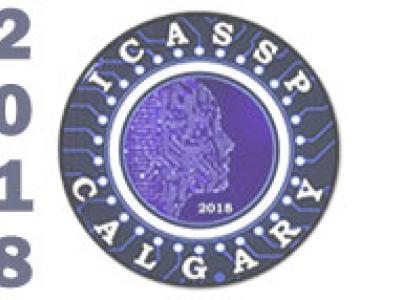
- Read more about ESTIMATION OF THE SOUND FIELD AT ARBITRARY POSITIONS IN DISTRIBUTED MICROPHONE NETWORKS BASED ON DISTRIBUTED RAY SPACE TRANSFORM
- Log in to post comments
- Categories:
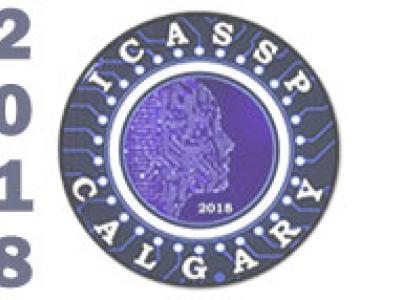
- Read more about Mobile Bayesian Spectrum Learning for Heterogeneous Networks
- Log in to post comments
Spectrum sensing in heterogeneous networks is very challenging as it usually requires a large number of static secondary users (SUs) to obtain the global spectrum states. In this paper, we tackle the spectrum sensing in heterogeneous networks from a new perspective. We exploit the mobility of multiple SUs to simultaneously collect spatial-temporal spectrum sensing data. Then, we propose a novel non-parametric Bayesian learning model, referred to as beta process hidden Markov model to capture the spatio-temporal correlation in the collected spectrum data.
- Categories:
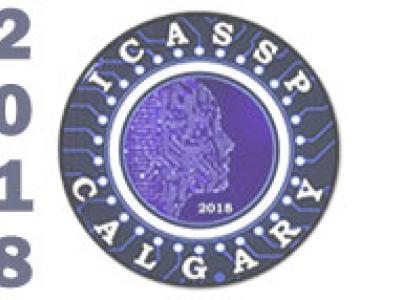
- Read more about Acoustic Scene Classification Using Discrete Random Hashing for Laplacian Kernel Machines
- Log in to post comments
icassp.pptx

- Categories: