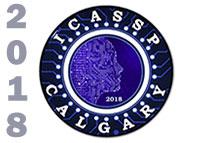
ICASSP is the world’s largest and most comprehensive technical conference focused on signal processing and its applications. The 2019 conference will feature world-class presentations by internationally renowned speakers, cutting-edge session topics and provide a fantastic opportunity to network with like-minded professionals from around the world. Visit website.
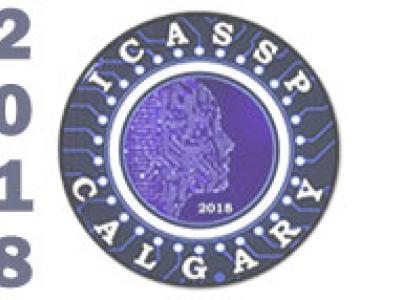
- Read more about IMAGE ALIGNMENT VIA MULTI-MODEL GEOMETRIC FIFTING AND HIERARCHICAL HOMOGRAPHY ESTIMATION
- Log in to post comments
- Categories:
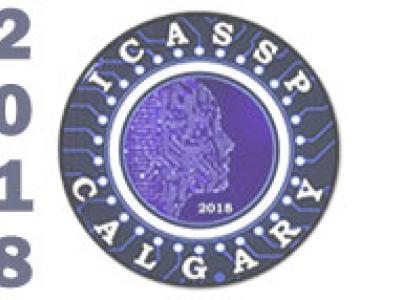
In this work, we introduce subset selection strategies for signal reconstruction based on kernel methods, particularly for the case of kernel-ridge regression. Typically, these methods are employed for exploiting known prior information about the structure of the signal of interest. We use the mean squared error and a scalar function of the covariance matrix of the kernel regressors to establish metrics for the subset selection problem. Despite the NP-hard nature of the problem, we introduce efficient algorithms for finding approximate solutions for the proposed metrics.
- Categories:
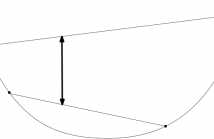
- Read more about THE CHORD GAP DIVERGENCE AND A GENERALIZATION OF THE BHATTACHARYYA DISTANCE
- Log in to post comments
- Categories:
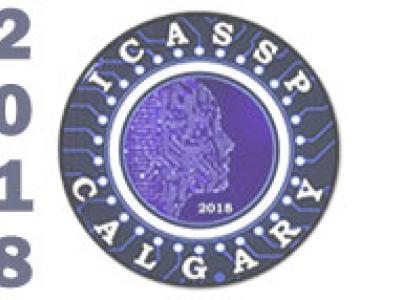
- Categories:
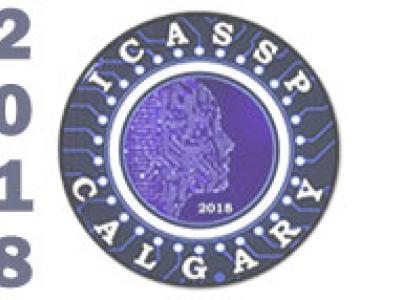
- Read more about Hi, BCD! Hybrid Inexact Block Coordinate Descent for Hyperspectral Super-Resolution
- Log in to post comments
Hyperspectral super-resolution (HSR) is a problem of recovering a high-spectral-spatial-resolution image from a multispectral measurement and a hyperspectral measurement, which have low spectral and spatial resolutions, respectively. We consider a low-rank structured matrix factorization formulation for HSR, which is a non-convex large-scale optimization problem. Our contributions contain both computational and theoretical aspects.
- Categories:
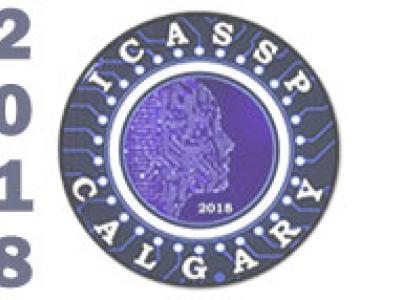
- Read more about MUSIC CHORD RECOGNITION BASED ON MIDI-TRAINED DEEP FEATURE AND BLSTM-CRF HYBIRD DECODING
- Log in to post comments
In this paper, we design a novel deep learning based hybrid system for automatic chord recognition. Currently, there is a bottleneck in the amount of enough annotated data for training robust acoustic models, as hand annotating time-synchronized chord labels requires professional musical skills and considerable labor. As a solution to this problem, we construct a large set of time synchronized MIDI-audio pairs, and use these data to train a Deep Residual Network (DRN) feature extractor, which can then estimate pitch class activations of real-world music audio recordings.
- Categories:
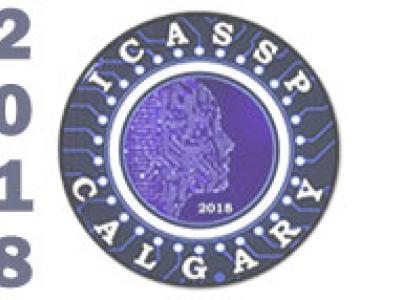
- Read more about ROBUST FEATURE CLUSTERING FOR UNSUPERVISED SPEECH ACTIVITY DETECTION
- Log in to post comments
In certain applications such as zero-resource speech processing
or very-low resource speech-language systems, it might
not be feasible to collect speech activity detection (SAD) annotations.
However, the state-of-the-art supervised SAD techniques
based on neural networks or other machine learning
methods require annotated training data matched to the target
domain. This paper establish a clustering approach for fully
unsupervised SAD useful for cases where SAD annotations
are not available. The proposed approach leverages Hartigan
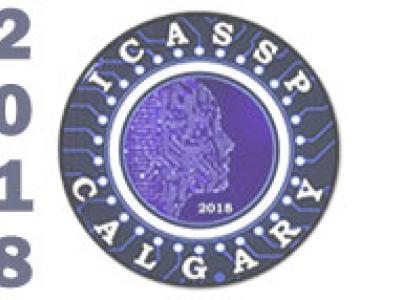
- Read more about HYBRID LSTM-FSMN NETWORKS FOR ACOUSTIC MODELING
- Log in to post comments
- Categories:
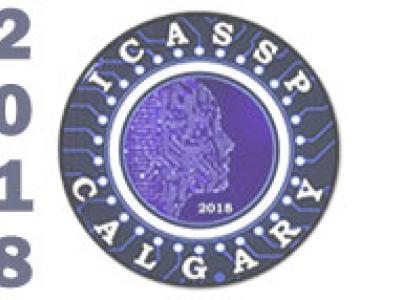
- Read more about COMPARING THE INFLUENCE OF DEPTH AND WIDTH OF DEEP NEURAL NETWORK BASED ON FIXED NUMBER OF PARAMETERS FOR AUDIO EVENT DETECTION
- Log in to post comments
Deep Neural Network (DNN) is a basic method used for the rare Acoustic Event Detection (AED) in synthesised audio. The structure of DNNs including Multi-Layer Perceptron (MLP) and Recurrent Neural Network (RNN) for AED tasks has rather fewer hidden layers compared with computer vision systems. This paper tries to demonstrate that a DNN with more hidden layers does not necessarily guarantee a better performance in AED tasks.
- Categories:
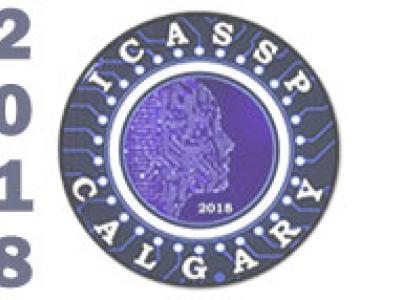
- Read more about L0-REGULARIZED HYBRID GRADIENT SPARSITY PRIORS FOR ROBUST SINGLE-IMAGE BLIND DEBLURRING
- Log in to post comments
Single-image blind deblurring is a challenging ill-posed in- verse problem which aims to estimate both blur kernel and latent sharp image from only one observation. This paper fo- cuses on first estimating the blur kernel alone and then restor- ing the latent image since it has been proven to be more feasi- ble to handle the ill-posed nature during blind deblurring. To estimate an accurate blur kernel, L0-norm of both first- and second-order image gradients is proposed to regularize the final estimation result.
- Categories: