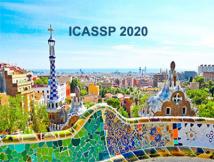
ICASSP is the world’s largest and most comprehensive technical conference focused on signal processing and its applications. The ICASSP 2020 conference will feature world-class presentations by internationally renowned speakers, cutting-edge session topics and provide a fantastic opportunity to network with like-minded professionals from around the world. Visit website.
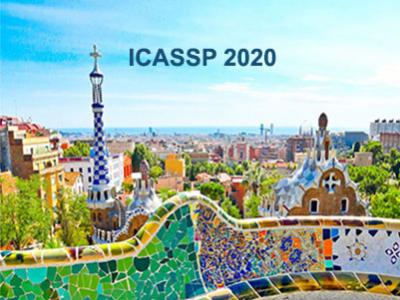
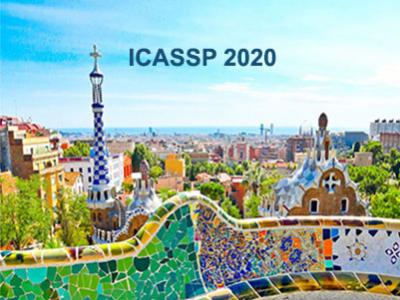
- Read more about IMPROVING PROSODY WITH LINGUISTIC AND BERT DERIVED FEATURES IN MULTI-SPEAKER BASED MANDARIN CHINESE NEURAL TTS
- Log in to post comments
Recent advances of neural TTS have made “human parity” synthesized speech possible when a large amount of studio-quality training data from a voice talent is available. However, with only limited, casual recordings from an ordinary speaker, human-like TTS is still a big challenge, in addition to other artifacts like incomplete sentences, repetition of words, etc.
- Categories:
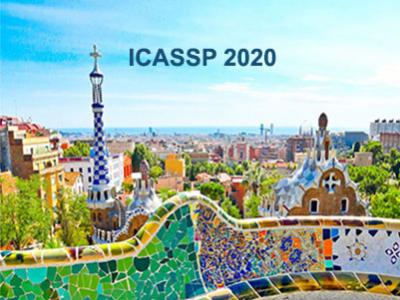
- Read more about Proximal Multitask Learning Over Distributed Networks with Jointly Sparse Structure
- Log in to post comments
- Categories:
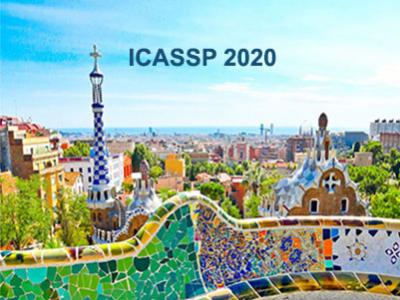
- Read more about ENHANCE FEATURE REPRESENTATION OF ELECTROENCEPHALOGRAM FOR SEIZURE DETECTION
- Log in to post comments
poster.pdf

- Categories:
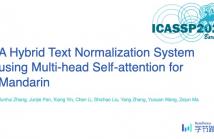
- Read more about A HYBRID TEXT NORMALIZATION SYSTEM USING MULTI-HEAD SELF-ATTENTION FOR MANDARIN
- Log in to post comments
In this paper, we propose a hybrid text normalization system using multi-head self-attention. The system combines the advantages of a rule-based model and a neural model for text preprocessing tasks. Previous studies in Mandarin text normalization usually use a set of hand-written rules, which are hard to improve on general cases. The idea of our proposed system is motivated by the neural models from recent studies and has a better performance on our internal news corpus. This paper also includes different attempts to deal with imbalanced pattern distribution of the dataset.
- Categories:
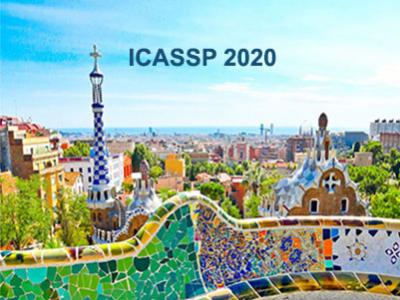
- Read more about Proximal Multitask Learning Over Distributed Networks with Jointly Sparse Structure
- Log in to post comments
- Categories:
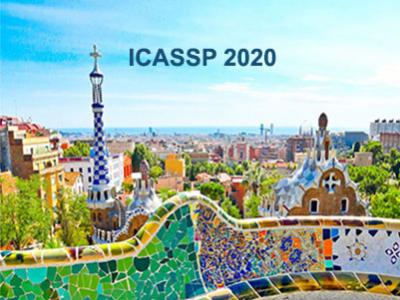
- Read more about A UNIFIED SEQUENCE-TO-SEQUENCE FRONT-END MODEL FOR MANDARIN TEXT-TO-SPEECH SYNTHESIS
- Log in to post comments
- Categories:
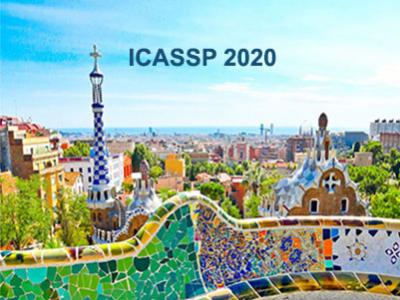
We address the problem of effectively handling overlapping speech in a diarization system. First, we detail a neural Long Short-Term Memory-based architecture for overlap detection. Secondly, detected overlap regions are exploited in conjunction with a frame-level speaker posterior matrix to make two-speaker assignments for overlapped frames in the resegmentation step. The overlap detection module achieves state-of-the-art performance on the AMI, DIHARD, and ETAPE corpora. We apply overlap-aware resegmentation on AMI, resulting in a 20% relative DER reduction over the baseline system.
- Categories:
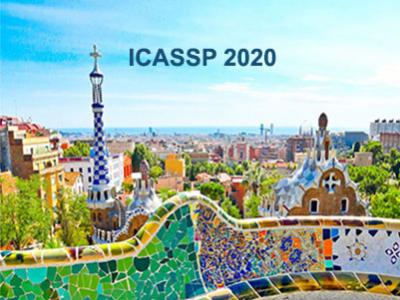
- Read more about COMPARE LEARNING: BI-ATTENTION NETWORK FOR FEW-SHOT LEARNING
- Log in to post comments
Learning with few labeled data is a key challenge for visual recognition, as deep neural networks tend to overfit using a few samples only. One of the Few-shot learning methods called metric learning addresses this challenge by first learning a deep distance metric to determine whether a pair of images belong to the same category, then applying the trained metric to instances from other test set with limited labels. This method makes the most of the few samples and limits the overfitting effectively.
- Categories:
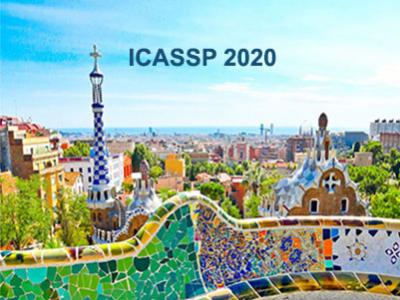
- Read more about Track-before-detect for sub-Nyquist radar
- Log in to post comments
Sub-Nyquist radars require fewer measurements, facilitating low-cost design, flexible resource allocation, etc. By applying compressed sensing (CS) method, such radars achieve close performance to traditional Nyquist radars. However in low signal-to-noise ratio (SNR) scenarios, detecting weak targets is challenging: low probability of detection and many spurious targets could occur in the recovery results of traditional CS method.
- Categories: