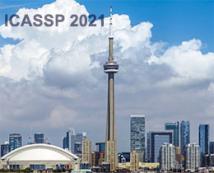
ICASSP 2021 - IEEE International Conference on Acoustics, Speech and Signal Processing is the world’s largest and most comprehensive technical conference focused on signal processing and its applications. The ICASSP 2021 conference will feature world-class presentations by internationally renowned speakers, cutting-edge session topics and provide a fantastic opportunity to network with like-minded professionals from around the world. Visit website.
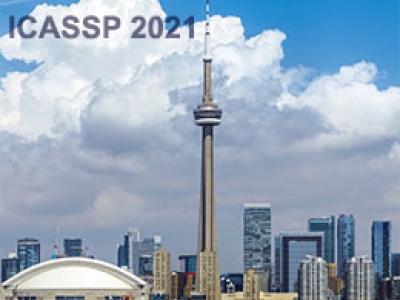
- Read more about A Joint Convolutional and Spatial Quad-Directional LSTM Network for Phase Unwrapping
- Log in to post comments
Phase unwrapping is a classical ill-posed problem which aims to recover the true phase from wrapped phase. In this paper, we introduce a novel Convolutional Neural Network (CNN) that incorporates a Spatial Quad-Directional Long Short Term Memory (SQD-LSTM) for phase unwrapping, by formulating it as a regression problem. Incorporating SQD-LSTM can circumvent the typical CNNs' inherent difficulty of learning global spatial dependencies which are vital when recovering the true phase. Furthermore, we employ a problem specific composite loss function to train this network.
- Categories:
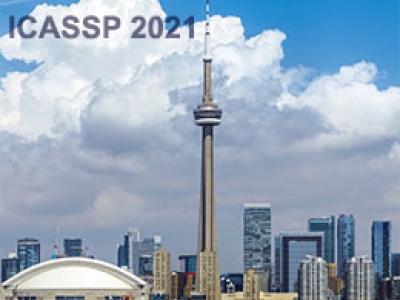
- Read more about SAGA: SPARSE ADVERSARIAL ATTACK ON EEG-BASED BRAIN COMPUTER INTERFACE
- Log in to post comments
With the recent advancement of the Brain-Computer Interface (BCI), Electroencephalogram (EEG) analytics gain a lot of research attention from various domains. Understanding the vulnerabilities of EEG analytics is important for safely applying this emerging technology in our daily life. Recent studies show that EEG analytics are vulnerable to adversarial attacks when adding small perturbations on the EEG data. However, fewer research efforts have been devoted to the robustness of EEG analytics under sparse perturbations that attack only small portions of the data.
- Categories:
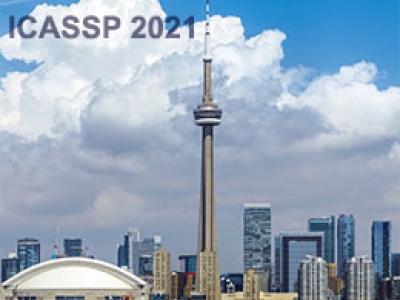
- Read more about ADL-MVDR: All deep learning MVDR beamformer for target speech separation
- Log in to post comments
Speech separation algorithms are often used to separate the target speech from other interfering sources. However, purely neural network based speech separation systems often cause nonlinear distortion that is harmful for automatic speech recognition (ASR) systems. The conventional mask-based minimum variance distortionless response (MVDR) beamformer can be used to minimize the distortion, but comes with high level of residual noise.
ICASSP Poster 1240.pdf

ICASSP slides 1240.pdf

- Categories:
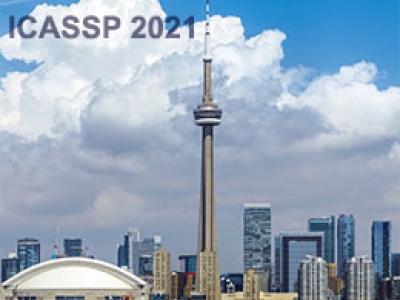
- Read more about Deep Deterministic Information Bottleneck with Matrix-Based Entropy Functional
- Log in to post comments
We introduce the matrix-based Renyi’s α-order entropy functional to parameterize Tishby et al. information bottleneck (IB) principle with a neural network. We term our methodology Deep Deterministic Information Bottleneck (DIB), as it avoids variational inference and distribution assumption. We show that deep neural networks trained with DIB outperform the variational objective counterpart and those that are trained
with other forms of regularization, in terms of generalization performance and robustness to adversarial attack. Code available at
- Categories:
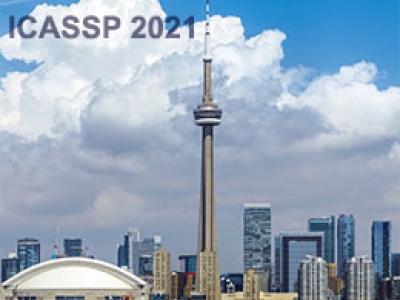
Humans express ideas, beliefs, and statements through language. The manner of expression can carry information indicating the author's degree of confidence in their statement. Understanding the certainty level of a claim is crucial in areas such as medicine, finance, engineering, and many others where errors can lead to disastrous results. In this work, we apply a joint model that leverages words and part-of-speech tags to improve hedge detection in text and achieve a new top score on the CoNLL-2010 Wikipedia corpus.
- Categories:
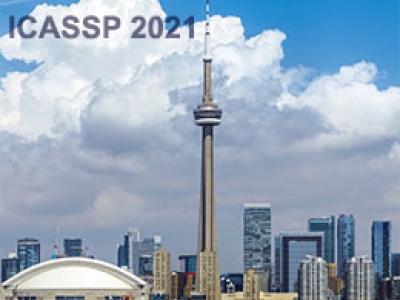
- Read more about AN END-TO-END NON-INTRUSIVE MODEL FOR SUBJECTIVE AND OBJECTIVE REAL-WORLD SPEECH ASSESSMENT USING A MULTI-TASK FRAMEWORK
- Log in to post comments
Speech assessment is crucial for many applications, but current intrusive methods cannot be used in real environments. Data-driven approaches have been proposed, but they use simulated speech materials or only estimate objective scores. In this paper, we propose a novel multi-task non-intrusive approach that is capable of simultaneously estimating both subjective and objective scores of real-world speech, to help facilitate learning. This approach enhances our prior work, which estimated subjective mean-opinion scores, where our
ICASSP Poster 3995.pdf

ICASSP Slides 3995.pdf

- Categories:
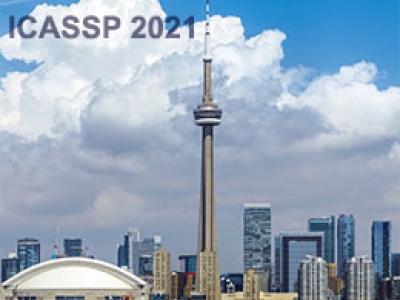
- Read more about CMIM: CROSS-MODAL INFORMATION MAXIMIZATION FOR MEDICAL IMAGING
- Log in to post comments
- Categories:
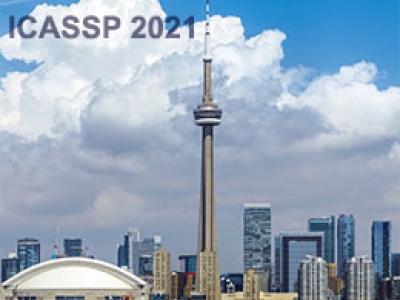
- Read more about A SPARSE CODING APPROACH TO AUTOMATIC DIET MONITORING WITH CONTINUOUS GLUCOSE MONITORS
- Log in to post comments
- Categories:
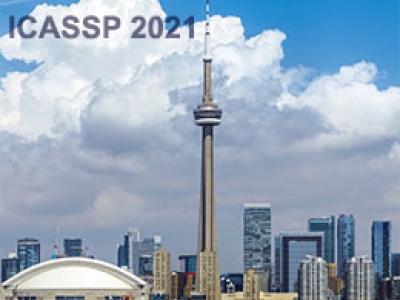
- Read more about PROGRESSIVE MULTI-STAGE FEATURE MIX FOR PERSON RE-IDENTIFICATION
- 1 comment
- Log in to post comments
slides.pdf

- Categories: