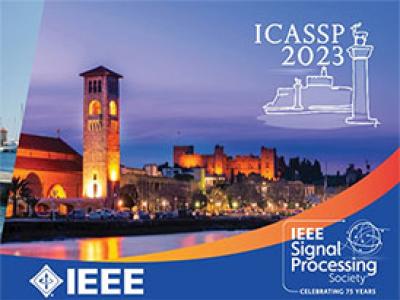
- Read more about Spatial Active Noise Control Method Based on Sound Field Interpolation From Reference Microphone Signals
- Log in to post comments
- Categories:
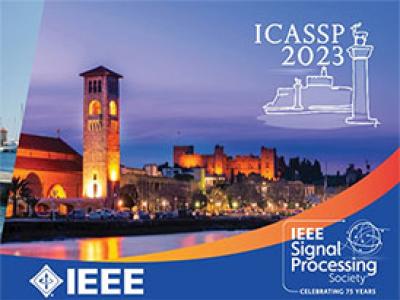
- Categories:
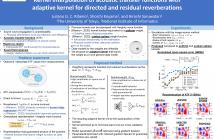
- Read more about Kernel interpolation of acoustic transfer functions with adaptive kernel for directed and residual reverberations
- Log in to post comments
An interpolation method for region-to-region acoustic transfer functions (ATFs) based on kernel ridge regression with an adaptive kernel is proposed. Most current ATF interpolation methods do not incorporate the acoustic properties for which measurements are performed. Our proposed method is based on a separate adaptation of directional weighting functions to directed and residual reverberations, which are used for adapting kernel functions. Thus, the proposed method can not only impose constraints on fundamental acoustic properties, but can also adapt to the acoustic environment.
- Categories:
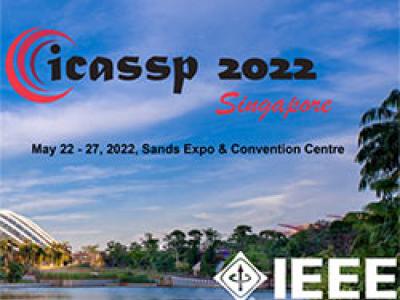
- Read more about Variable span trade-off filter for sound zone control with kernel interpolation weighting
- Log in to post comments
A sound zone control method is proposed, based on the frequency domain variable span trade-off filter (VAST). Existing VAST methods optimizes the sound field at a set of discrete points, while the proposed method uses kernel interpolation to instead optimize the sound field over a continuous region. When the loudspeaker positions are known, the performance can be improved further by applying a directional weighting to the interpolation procedure.
- Categories:
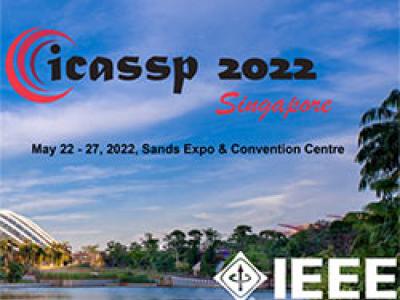
- Read more about Variable span trade-off filter for sound zone control with kernel interpolation weighting
- Log in to post comments
A sound zone control method is proposed, based on the frequency domain variable span trade-off filter (VAST). Existing VAST methods optimizes the sound field at a set of discrete points, while the proposed method uses kernel interpolation to instead optimize the sound field over a continuous region. When the loudspeaker positions are known, the performance can be improved further by applying a directional weighting to the interpolation procedure.
- Categories:
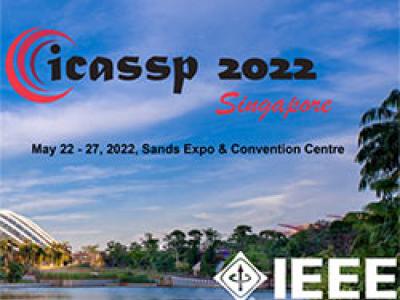
- Read more about Closed-form single source direction-of-arrival estimator using first-order relative harmonic coefficients
- Log in to post comments
- Categories:
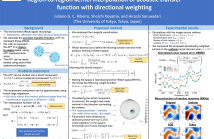
- Read more about Region-to-region kernel interpolation of acoustic transfer function with directional weighting
- Log in to post comments
A method of interpolating the acoustic transfer function (ATF) between regions that takes into account both the physical properties of the ATF and the directionality of region configurations is proposed. Most spatial ATF interpolation methods are limited to estimation in the region of receivers. A kernel method for region-to-region ATF interpolation makes it possible to estimate the ATFs for both source and receiver regions from a discrete set of ATF measurements.
- Categories:
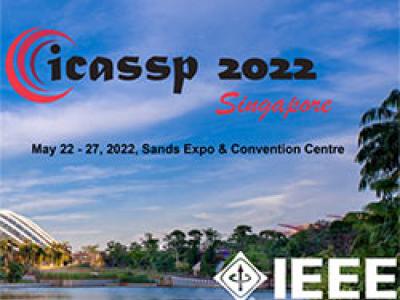
- Read more about ON SYNCHRONIZATION OF WIRELESS ACOUSTIC SENSOR NETWORKS IN THE PRESENCE OF TIME-VARYING SAMPLING RATE OFFSETS AND SPEAKER CHANGES
- Log in to post comments
poster_landscape.pdf

slides.pdf

- Categories:
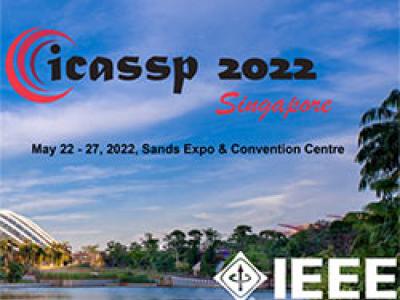
- Read more about SPARSITY-BASED SOUND FIELD SEPARATION IN THE SPHERICAL HARMONICS DOMAIN
- Log in to post comments
Sound field analysis and reconstruction has been a topic of intense research in the last decades for its multiple applications in spatial audio processing tasks. In this context, the identification of the direct and reverberant sound field components is a problem of great interest, where several solutions exploiting spherical harmonics representations have already been proposed.
- Categories: