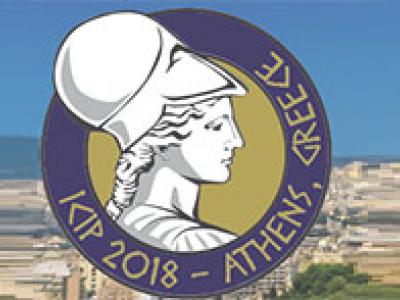
This paper presents an empirical Bayesian method to estimate regularisation parameters in imaging inverse problems. The method calibrates regularisation parameters directly from the observed data by maximum marginal likelihood estimation, and is useful for inverse problems that are convex. A main novelty is that maximum likelihood estimation is performed efficiently by using a stochastic proximal gradient algorithm that is driven by two proximal Markov chain Monte Carlo samplers, intimately combining modern optimisation and sampling techniques.
- Categories:
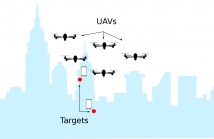
- Read more about Collaborative Target-Localization and Information-based Control in Networks of UAVs
- Log in to post comments
In this paper, we study the capacity of UAV networks for high-accuracy localization of targets. We address the problem of designing a distributed control scheme for UAV navigation and formation based on an information-seeking criterion maximizing the target localization accuracy. Each UAV is assumed to be able to communicate and collaborate with other UAVs that are within a neighboring region, allowing for a feasible distributed solution which takes into account a trade-off between localization accuracy and speed of convergence to a suitable localization of the target.
- Categories:
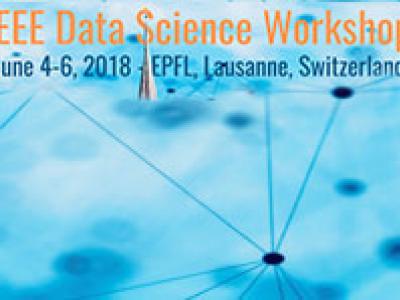
- Read more about Uncertainty Quantification in Sunspot Counts
- Log in to post comments
- Categories:
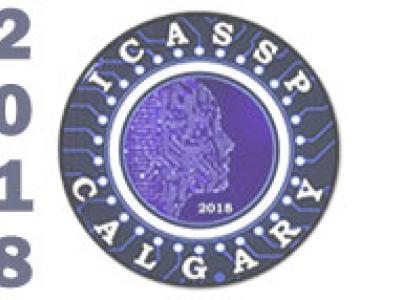
- Read more about PLUG-IN MEASURE-TRANSFORMED QUASI-LIKELIHOOD RATIO TEST FOR RANDOM SIGNAL DETECTION
- Log in to post comments
Recently, we developed a robust generalization of the Gaussian quasi-likelihood ratio test (GQLRT). This generalization, called measure-transformed GQLRT (MT-GQLRT), operates by selecting a Gaussian model that best empirically fits a transformed probability measure of the data. In this letter, a plug-in version of the MT-GQLRT is developed for robust detection of a random signal in nonspherical noise. The proposed detector is derived by plugging an empirical measure-transformed noise covariance, ob- tained from noise-only secondary data, into the MT-GQLRT.
- Categories:
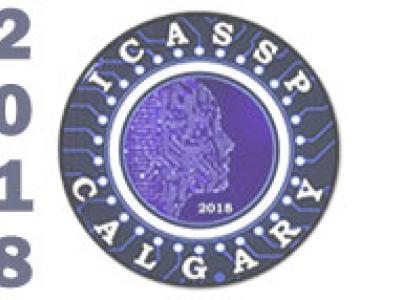
- Read more about SEQUENTIAL INFERENCE METHODS FOR NON-HOMGENEOUS POISSON PROCESSES WITH STATE-SPACE PRIOR
- Log in to post comments
The non-homogeneous Poisson process (NHPP) is a point process with time-varying intensity across its domain, the use of which arises in numerous domains in signal processing, machine learning and many other fields. However, its applications are largely limited by the intractable likelihood and the high computational cost of existing inference schemes. We present an online inference framework that utilises generative Poisson data and sequential Markov Chain Monte Carlo (SMCMC) algorithm, which achieves improved performance in both synthetic and real datasets.
- Categories:
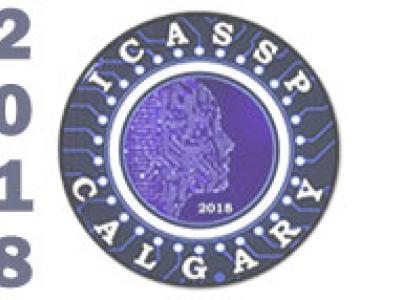
- Read more about DISTRIBUTED APPROXIMATE MESSAGE PASSING WITH SUMMATION PROPAGATION
- Log in to post comments
In this paper, we propose a fully distributed approximate message passing (AMP) algorithm, which reconstructs an unknown vector from its linear measurements obtained at nodes in a network. The proposed algorithm is a distributed implementation of the centralized AMP algorithm, and consists of the local computation at each node and the global computation using communications between nodes. For the global computation, we propose a distributed algorithm named summation propagation to calculate a summation required in the AMP algorithm.
ICASSP2018.pdf

- Categories:
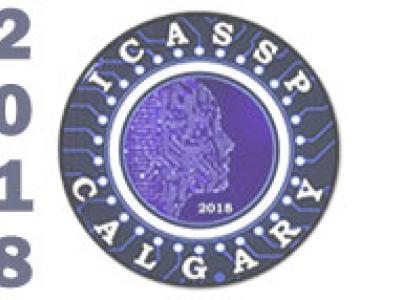
In this paper, we consider the problem of sparse signal detection with compressed measurements in a Bayesian framework. Multiple nodes in the network are assumed to observe sparse signals. Observations at each node are compressed via random projections and sent to a centralized fusion center. Motivated by the fact that reliable detection of the sparse signals does not require complete signal reconstruction, we propose two computationally efficient methods for constructing decision statistics for detection.
ICASSP.pdf

- Categories:
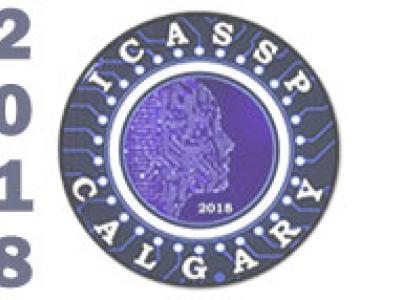
- Read more about On Sequential Random Distortion Testing of Non-Stationary Processes
- Log in to post comments
Random distortion testing (RDT) addresses the problem of testing whether or not a random signal deviates by more than a specified tolerance from a fixed value. The test is non-parametric in the sense that the distribution of the signal under each hypothesis is assumed to be unknown. The signal is observed in independent and identically distributed (i.i.d) additive noise. The need to control the probabilities of false alarm and missed de- tection while reducing the number of samples required to make a decision leads to the SeqRDT approach.
- Categories:
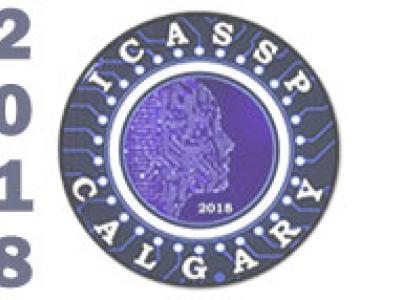
- Read more about Locally Optimal Invariant Detector for Testing Equality of Two Power Spectral Densities
- Log in to post comments
- Categories:
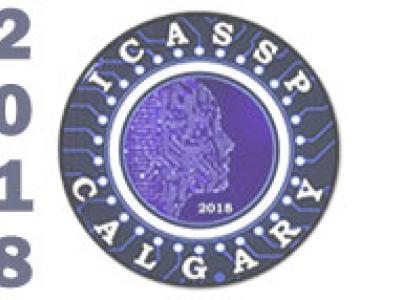
- Read more about HUMAN-MACHINE INFERENCE NETWORKS FOR SMART DECISION MAKING: OPPORTUNITIES AND CHALLENGES
- Log in to post comments
The emerging paradigm of Human-Machine Inference Networks (HuMaINs) combines complementary cognitive strengths of humans and machines in an intelligent manner to tackle various inference tasks and achieves higher performance than either humans or machines by themselves. While inference performance optimization techniques for human-only or sensor-only networks are quite mature, HuMaINs require novel signal processing and machine learning solutions.
- Categories: