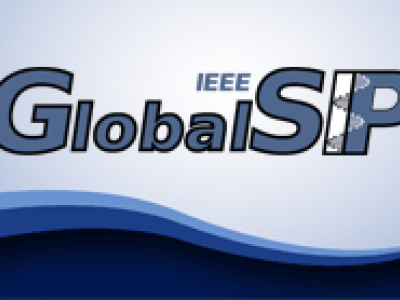
- Read more about FAST METHODS FOR RECOVERING SPARSE PARAMETERS IN LINEAR LOW RANK MODELS
- Log in to post comments
Poster.pdf

- Categories:
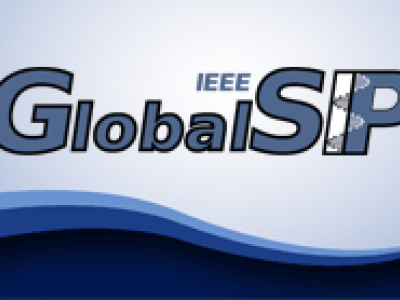
- Read more about FAST METHODS FOR RECOVERING SPARSE PARAMETERS IN LINEAR LOW RANK MODELS
- Log in to post comments
Poster.pdf

- Categories:
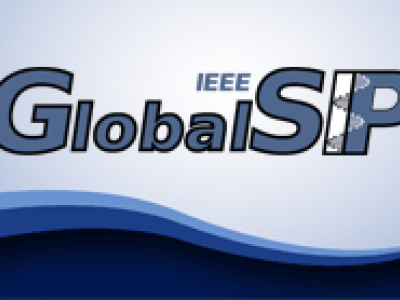
- Read more about DETECTION DIVERSITY OF SPATIO-TEMPORAL DATA USING PITMAN’S EFFICIENCY FOR LOW SNR REGIMES
- Log in to post comments
In this work, we use Pitman’s efficiency to characterize the diversity of a spatio-temporal distributed detection system. Pitman’s efficiency directly measures the detection ability of the data at low signal to noise ratios (SNRs). We study how the
- Categories:
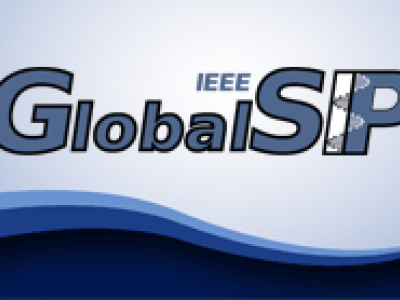
- Read more about Robust Regularized Least-Squares Beamforming Approach to Signal Estimation
- Log in to post comments
In this paper, we address the problem of robust adaptive beamforming of signals received by a linear array. The challenge associated with the beamforming problem is twofold. Firstly, the process requires the inversion of the usually ill-conditioned covariance matrix of the received signals. Secondly, the steering vector pertaining to the direction of arrival of the signal of interest is not known precisely. To tackle these two challenges, the standard capon beamformer is manipulated to a form where the beamformer output is obtained as a scaled version of the inner product of two vectors.
- Categories:
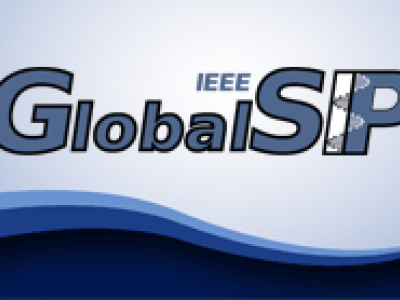
- Read more about Blind Digital Modulation Classification based on M-th Power nonlinear Transformation
- Log in to post comments
Automatic Modulation Classification (AMC) has received a major attention last decades, as a required step between signal detection and demodulation. In the fully-blind scenario, this task turns out to be quite challenging, especially when the computational complexity and the robustness to uncertainty matter. AMC commonly relies on a preprocessor whose function is to estimate unknown parameters, filter the received signal and sample it in a suitable way. Any preprocessing error inherently leads to a performance loss.
- Categories:
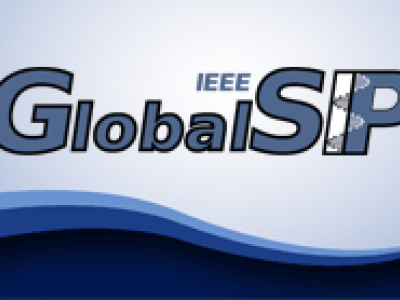
- Read more about D-OAMP: A Denoising-based Signal Recovery Algorithm for Compressed Sensing
- Log in to post comments
- Categories:
- Read more about Technique for Numerical Computation of Cramér-Rao Bound using MATLAB
- Log in to post comments
The purpose of this note is to provide an effective means to compute the Cramér-Rao lower bound numerically for deterministic parameter estimation problems with the use of symbolic computation in MATLAB.
- Categories:
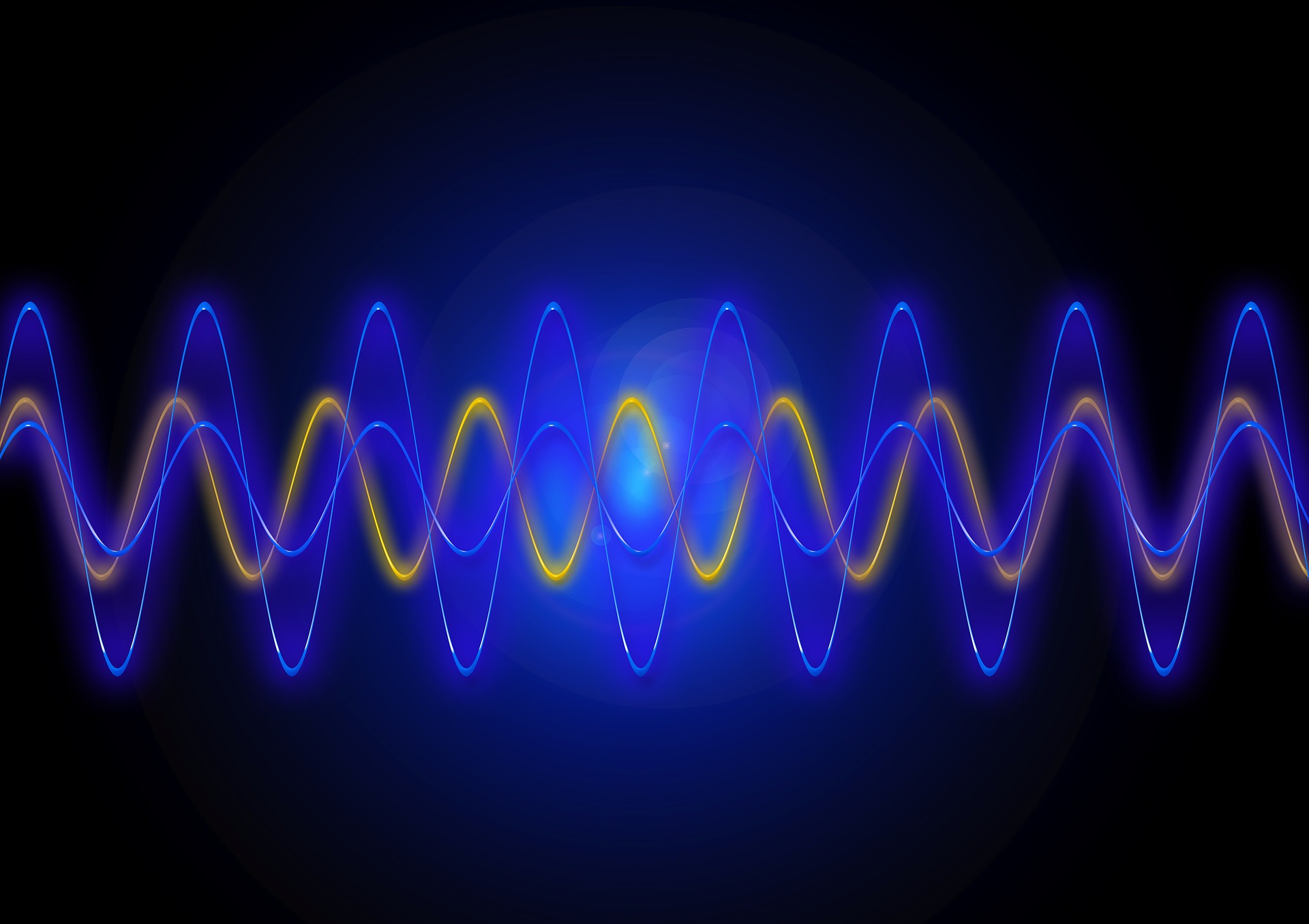
- Read more about Robust Estimation of Self-Exciting Point Process Models with Application to Neuronal Modeling
- Log in to post comments
We consider the problem of estimating discrete self- exciting point process models from limited binary observations, where the history of the process serves as the covariate. We analyze the performance of two classes of estimators: l1-regularized maximum likelihood and greedy estimation for a discrete version of the Hawkes process and characterize the sampling tradeoffs required for stable recovery in the non-asymptotic regime. Our results extend those of compressed sensing for linear and generalized linear models with i.i.d.
- Categories:
- Read more about Particle filters with independent resampling
- Log in to post comments
- Categories:
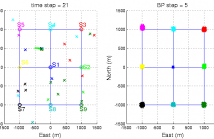
- Read more about Distributed Estimation of Latent Parameters in State Space Models Using Separable Likelihoods
- Log in to post comments
This work is a part of our research on scalable and/or distributed fusion and sensor calibration. We address parameter estimation in multi-sensor state space models which underpins surveillance applications with sensor networks. The parameter likelihood of the problem involves centralised Bayesian filtering of multi-sensor data, which lacks scalability with the number of sensors and induces a large communication load. We propose separable likelihoods which approximate the centralised likelihood with single sensor filtering terms.
- Categories: