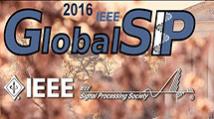
A flagship conference of the IEEE Signal Processing Society, GlobalSIP is structured around coherent symposia that explore new and emerging developments in the field, while maintaining a format that encourages accessibility to interested researchers and fosters interaction and cross-pollination of ideas.
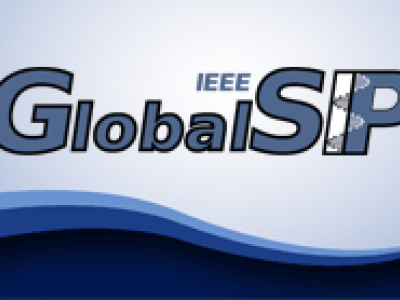
- Read more about Rethinking Sketching as Sampling: Efficient Approximate Solution to Linear Inverse Problems
- Log in to post comments
Sampling and reconstruction of bandlimited graph signals have well-appreciated merits for dimensionality reduction, affordable storage, and online processing of streaming network data. However, these parsimonious signals are oftentimes encountered with high-dimensional linear inverse problems. Hence, interest shifts from reconstructing the signal itself towards instead approximating the input to a prescribed linear operator efficiently.
- Categories:
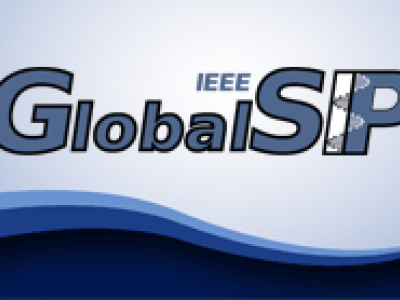
- Read more about Image Compression via Multiple Learned Geometric Dictionaries
- Log in to post comments
- Categories:
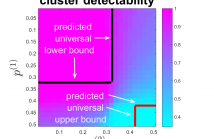
- Read more about Multilayer Spectral Graph Clustering via Convex Layer Aggregation
- Log in to post comments
Multilayer graphs are commonly used for representing different relations between entities and handling heterogeneous data processing tasks. New challenges arise in multilayer graph clustering for assigning clusters to a common multilayer node set and for combining information from each layer. This paper presents a theoretical framework for multilayer spectral graph clustering of the nodes via convex layer aggregation.
- Categories:
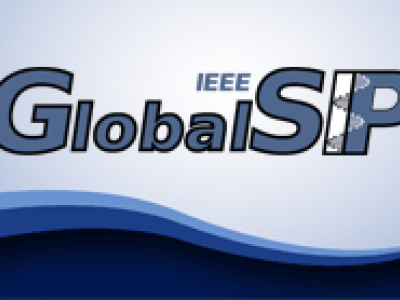
- Read more about Sampling solutions of Schrödinger equations on combinatorial graphs
- Log in to post comments
We consider functions on a weighted combinatorial graph G (finite or countable) whose evolution in time −∞ < t < ∞ is governed by the Schro ̈dinger type equation ∂g(t, v)/∂t = i∆g(t, v), v ∈ V (G), with the combinatorial Laplace operator on the right side.
Global2016.pdf

- Categories:
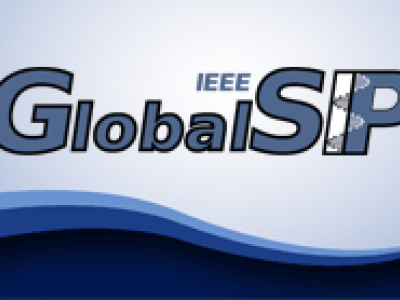
- Read more about Persistent-Homology-based Detection of Power System Low-frequency Oscillations using PMUs
- Log in to post comments
This paper presents a new methodology to detect low-frequency
oscillations in power grids by use of time-synchronized data
from phasor measurement unit (PMU). Principal component analysis
(PCA) is first applied to the massive PMU data to extract the
low-dimensional features, i.e., the principal components (PCs). Then
based on persistent homology, a \emph{cyclicity response function}
is proposed to detect low-frequency oscillations through the use of
PCs. Whenever the cyclicity response exceeds a numerically robust
- Categories:
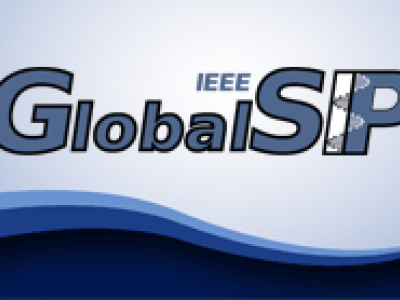
- Read more about Rethinking Sketching as Sampling: Efficient Approximate Solution to Linear Inverse Problems
- Log in to post comments
Sampling and reconstruction of bandlimited graph signals have well-appreciated merits for dimensionality reduction, affordable storage, and online processing of streaming network data. However, these parsimonious signals are oftentimes encountered with high-dimensional linear inverse problems. Hence, interest shifts from reconstructing the signal itself towards instead approximating the input to a prescribed linear operator efficiently.
- Categories:
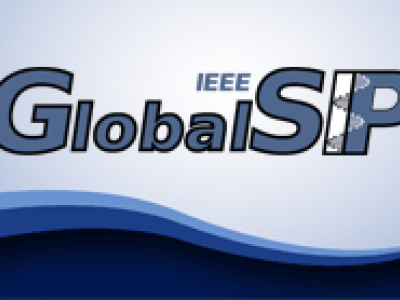
- Read more about Scalable and Robust PCA Approach with Random Column/Row Sampling
- Log in to post comments
- Categories:
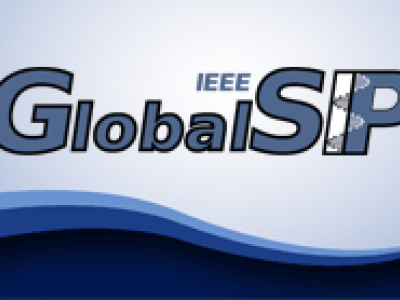
- Read more about Active Regression with Compressive Sensing based Outlier Mitigation for both Small and Large Outliers
- Log in to post comments
In this paper, a new active learning scheme is proposed for linear
regression problems with the objective of resolving the insufficient
training data problem and the unreliable training data labeling prob-
lem. A pool-based active regression technique is applied to select the
optimal training data to label from the overall data pool. Then, com-
pressive sensing is exploited to remove labeling errors if the errors
are sparse and have large enough magnitudes, which are called large
- Categories:
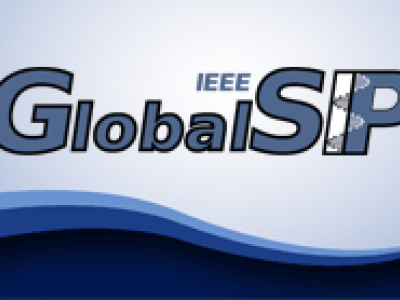
- Read more about COHERENCE FUNCTION ESTIMATION WITH A DERIVATIVE CONSTRAINT FOR POWER GRID OSCILLATION DETECTION
- Log in to post comments
- Categories:
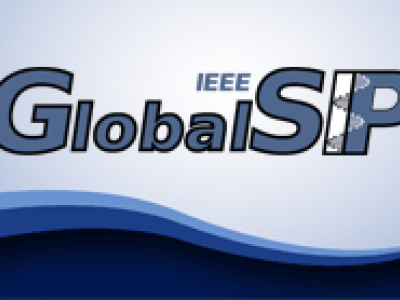
- Read more about COHERENCE FUNCTION ESTIMATION WITH A DERIVATIVE CONSTRAINT FOR POWER GRID OSCILLATION DETECTION
- Log in to post comments
- Categories: