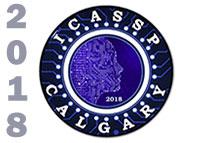
ICASSP is the world’s largest and most comprehensive technical conference focused on signal processing and its applications. The 2019 conference will feature world-class presentations by internationally renowned speakers, cutting-edge session topics and provide a fantastic opportunity to network with like-minded professionals from around the world. Visit website.
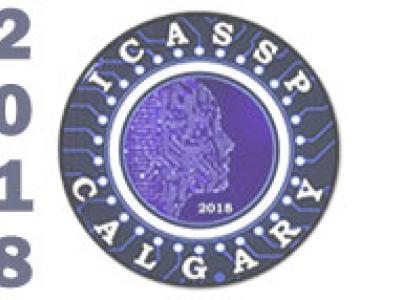
- Read more about CLASSIFICATION OF CORALS IN REFLECTANCE AND FLUORESCENCE IMAGES USING CONVOLUTIONAL NEURAL NETWORK REPRESENTATIONS
- Log in to post comments
Coral species, with complex morphology and ambiguous boundaries, pose a great challenge for automated classification. CNN activations, which are extracted from fully connected layers of deep networks (FC features), have been successfully used as powerful universal representations in many visual tasks. In this paper, we investigate the transferability and combined performance of FC features and CONV features (extracted
- Categories:
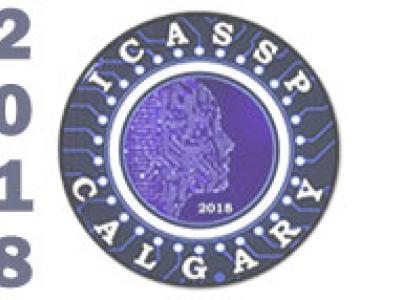
- Read more about VR IQA NET: Deep Virtual Reality Image Quality Assessment using Adversarial Learning
- Log in to post comments
In this paper, we propose a novel virtual reality image quality assessment (VR IQA) with adversarial learning for omnidirectional images. To take into account the characteristics of the omnidirectional image, we devise deep networks including novel quality score predictor and human perception guider. The proposed quality score predictor automatically predicts the quality score of distorted image using the latent spatial and position feature.
- Categories:
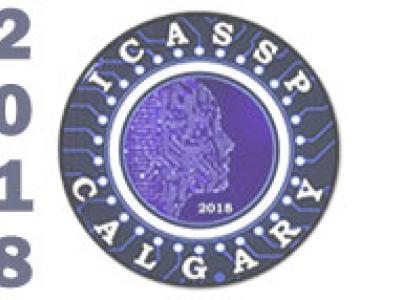
- Read more about DEEP FACTORIZATION FOR SPEECH SIGNAL
- Log in to post comments
Various informative factors mixed in speech signals, leading to great difficulty when decoding any of the factors. An intuitive idea is to factorize each speech frame into individual informative factors, though it turns out to be highly difficult. Recently, we found that speaker traits, which were assumed to be long-term distributional properties, are actually short-time patterns, and can be learned by a carefully designed deep neural network (DNN). This discovery motivated a cascade deep factorization (CDF) framework that will be presented in this paper.
- Categories:
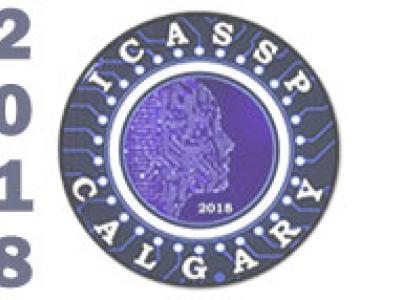
In recent studies, it has shown that speaker patterns can be learned from very short speech segments (e.g., 0.3 seconds) by a carefully designed convolutional & time-delay deep neural network (CT-DNN) model. By enforcing the model to discriminate the speakers in the training data, frame-level speaker features can be derived from the last hidden layer.
- Categories:
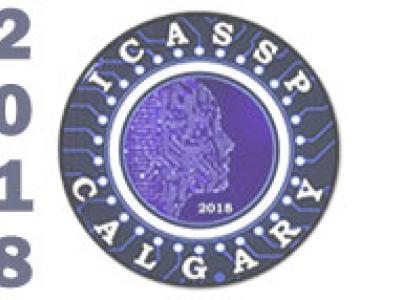
- Read more about High-speed Optical Camera Communication Using an Optimally Modulated Signal
- Log in to post comments
This paper describes a high-speed optical camera communication (OCC) technique using an LED and a rolling-shutter camera. In the proposed technique, the symbols being transmitted are encoded as time delays of optimally modulated signals derived theoretically. A receiver decodes the symbols by using intensities obtained from four consecutive line sensors of a camera.
- Categories:
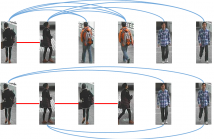
- Read more about Deep Feature Embedding Learning for Person Re-Identification Using Lifted Structured Loss
- Log in to post comments
In this paper, we propose deep feature embedding learning for person re-identification (re-id) using lifted structured loss. Although triplet loss has been commonly used in deep neural networks for person re-id, the triplet loss-based framework is not effective in fully using the batch information. Thus, it needs to choose hard negative samples manually that is very time-consuming. To address this problem, we adopt lifted structured loss for deep neural networks that makes the network learn better feature embedding by minimizing intra-class variation and maximizing inter-class variation.
- Categories:
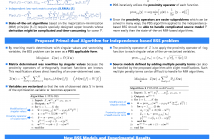
- Read more about Determined Blind Source Separation via Proximal Splitting Algorithm
- Log in to post comments
The state-of-the-art algorithms of determined blind source separation (BSS) methods based on the independent component analysis
- Categories:
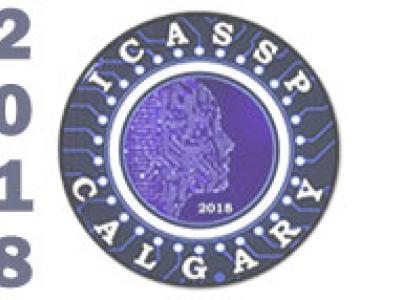
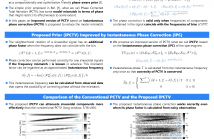
- Read more about Phase Corrected Total Variation for Audio Signals
- Log in to post comments
In optimization-based signal processing, the so-called prior term models the desired signal, and therefore its design is the key factor to achieve a good performance. For audio signals, the time-directional total variation applied to a spectrogram in combination with phase correction has been proposed recently to model sinusoidal components of the signal. Although it is a promising prior, its applicability might be restricted to some extent because of the mismatch of the assumption to the signal.
- Categories:
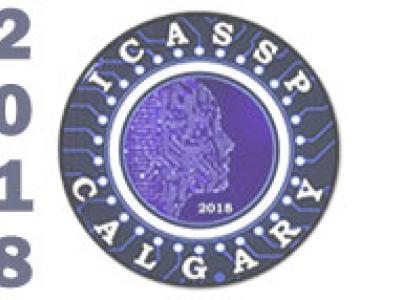
- Read more about SEQUENTIAL INFERENCE METHODS FOR NON-HOMGENEOUS POISSON PROCESSES WITH STATE-SPACE PRIOR
- Log in to post comments
The non-homogeneous Poisson process (NHPP) is a point process with time-varying intensity across its domain, the use of which arises in numerous domains in signal processing, machine learning and many other fields. However, its applications are largely limited by the intractable likelihood and the high computational cost of existing inference schemes. We present an online inference framework that utilises generative Poisson data and sequential Markov Chain Monte Carlo (SMCMC) algorithm, which achieves improved performance in both synthetic and real datasets.
- Categories: