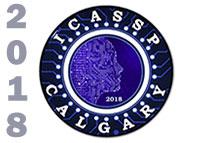
ICASSP is the world’s largest and most comprehensive technical conference focused on signal processing and its applications. The 2019 conference will feature world-class presentations by internationally renowned speakers, cutting-edge session topics and provide a fantastic opportunity to network with like-minded professionals from around the world. Visit website.
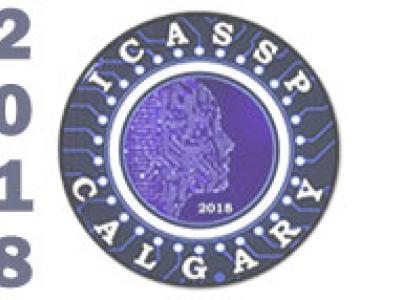
In this paper, we consider the problem of sparse signal detection with compressed measurements in a Bayesian framework. Multiple nodes in the network are assumed to observe sparse signals. Observations at each node are compressed via random projections and sent to a centralized fusion center. Motivated by the fact that reliable detection of the sparse signals does not require complete signal reconstruction, we propose two computationally efficient methods for constructing decision statistics for detection.
ICASSP.pdf

- Categories:
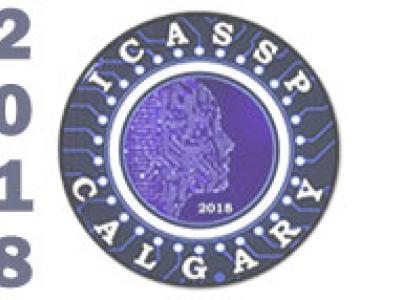
- Read more about Low-Rank Optimization for Data Shuffling in Wireless Distributed Computing
- Log in to post comments
Wireless distributed computing presents new opportunities to execute intelligent tasks on mobile devices for low-latency applications, by wirelessly aggregating the computation and storage resources among mobile devices. However, for low-latency applications, the key bottleneck lies in the exchange of intermediate results among mobile devices for data shuffling. To improve communication efficiency therein, we establish a novel interference alignment condition by exploiting the locally computed intermediate values as side information.
- Categories:
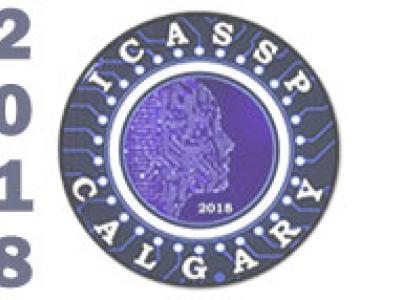
- Read more about On Sequential Random Distortion Testing of Non-Stationary Processes
- Log in to post comments
Random distortion testing (RDT) addresses the problem of testing whether or not a random signal deviates by more than a specified tolerance from a fixed value. The test is non-parametric in the sense that the distribution of the signal under each hypothesis is assumed to be unknown. The signal is observed in independent and identically distributed (i.i.d) additive noise. The need to control the probabilities of false alarm and missed de- tection while reducing the number of samples required to make a decision leads to the SeqRDT approach.
- Categories:
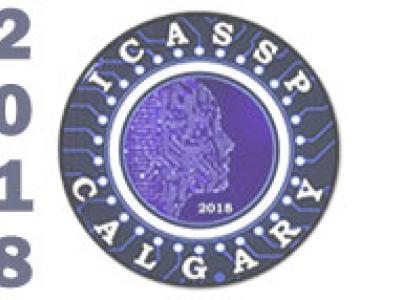
- Read more about On Sequential Random Distortion Testing of Non-Stationary Processes
- Log in to post comments
Random distortion testing (RDT) addresses the problem of testing whether or not a random signal deviates by more than a specified tolerance from a fixed value. The test is non-parametric in the sense that the distribution of the signal under each hypothesis is assumed to be unknown. The signal is observed in independent and identically distributed (i.i.d) additive noise. The need to control the probabilities of false alarm and missed de- tection while reducing the number of samples required to make a decision leads to the SeqRDT approach.
- Categories:
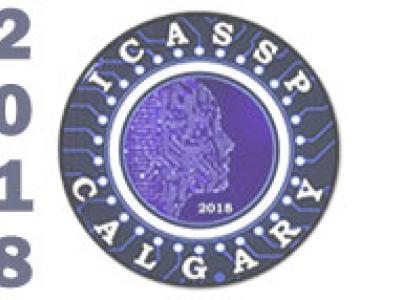
- Read more about Optimal Crowdsourced Classification with a Reject Option in the Presence of Spammers
- Log in to post comments
- Categories:
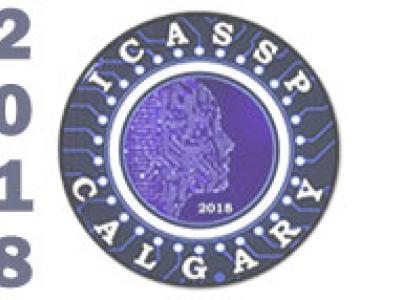
- Read more about Fusion of Multiple Multiband Images with Complementary Spatial and Spectral Resolutions
- Log in to post comments
- Categories:
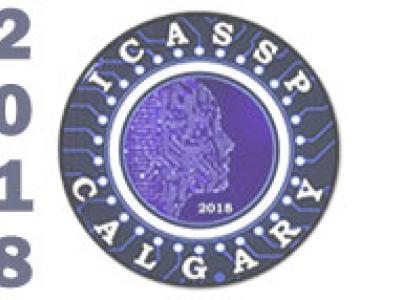
- Read more about Deep Blind Image Quality Assessment by Learning Sensitivity Map
- Log in to post comments
Applying a deep convolutional neural network CNN to no reference image quality assessment (NR-IQA) is a challenging task due to the lack of a training database. In this paper, we propose a CNN-based NR-IQA framework that can effectively solve this problem. The proposed method–the Deep Blind image Quality Assessment predictor (DeepBQA)– adopts two-step training stages to avoid overfitting. In the first stage, a ground-truth objective error map is generated and used as a proxy training target.
- Categories:
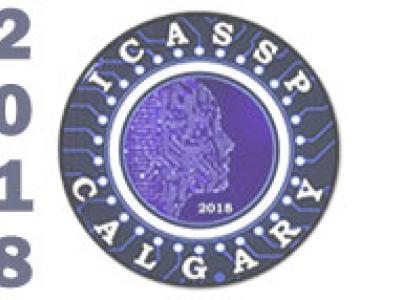
- Read more about PARAMETER ESTIMATION OF HEAVY-TAILED RANDOM WALK MODEL FROM INCOMPLETE DATA
- Log in to post comments
- Categories:
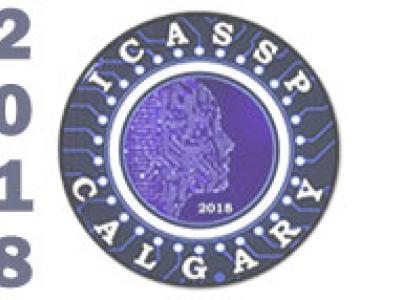
- Read more about AUTOMATIC TEMPORAL SEGMENTATION OF HAND MOVEMENTS FOR HAND POSITIONS RECOGNITION IN FRENCH CUED SPEECH
- Log in to post comments
In the context of Cued Speech (CS) recognition, the recognition
of lips and hand movements is a key task. As we know, a good
temporal segmentation is necessary for the supervised recog-
nition system. However, lips and hand streams cannot share
the same temporal segmentation since they are not synchro-
nized. In this work, we propose a hand preceding model to
predict temporal segmentations of hand movements automati-
cally by exploring the relationship between hand preceding time
- Categories: