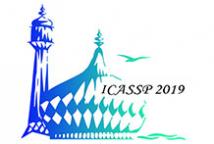
ICASSP is the world’s largest and most comprehensive technical conference focused on signal processing and its applications. The 2019 conference will feature world-class presentations by internationally renowned speakers, cutting-edge session topics and provide a fantastic opportunity to network with like-minded professionals from around the world. Visit website.
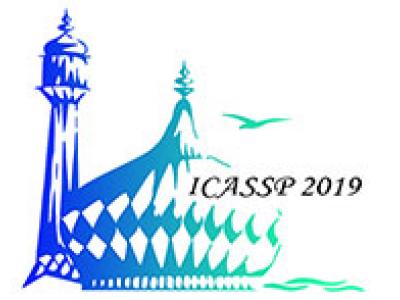
- Read more about Neural Variational Identification and Filtering
- Log in to post comments
- Categories:
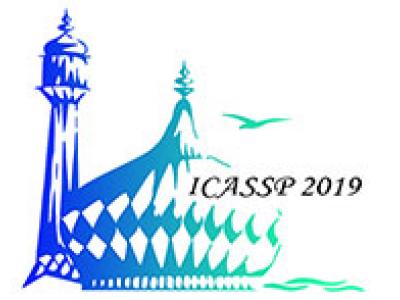
- Read more about [Slides] Accelerating Iterative Hard Thresholding for Low-rank Matrix Completion via Adaptive Restart
- Log in to post comments
This paper introduces the use of adaptive restart to accelerate iterative hard thresholding (IHT) for low-rank matrix completion. First, we analyze the local convergence of accelerated IHT in the non-convex setting of matrix completion problem (MCP). We prove the linear convergence rate of the accelerated algorithm inside the region near the solution. Our analysis poses a major challenge to parameter selection for accelerated IHT when no prior knowledge of the "local Hessian condition number" is given.
- Categories:
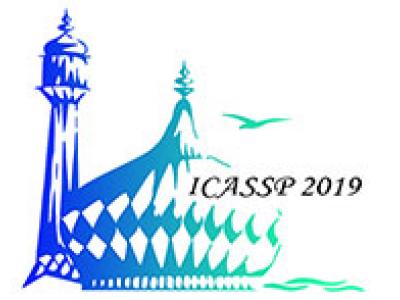
- Read more about PERFORMANCE BOUND FOR BLIND EXTRACTION OF NON-GAUSSIAN COMPLEX-VALUED VECTOR COMPONENT FROM GAUSSIAN BACKGROUND
- Log in to post comments
Independent Vector Extraction aims at the joint blind source extraction of $K$ dependent signals of interest (SOI) from $K$ mixtures (one signal from one mixture). Similarly to Independent Component/Vector Analysis (ICA/IVA), the SOIs are assumed to be independent of the other signals in the mixture. Compared to IVA, the (de-)mixing IVE model is reduced in the number of parameters for the extraction problem. The SOIs are assumed to be non-Gaussian or noncircular Gaussian, while the other signals are modeled as circular Gaussian.
- Categories:
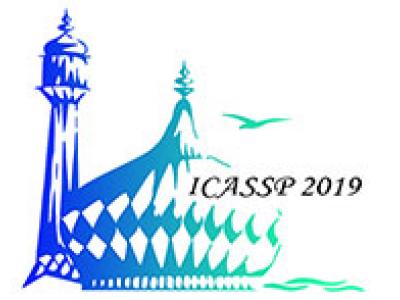
- Read more about Kernel Random Matrices of Large Concentrated Data: The Example of GAN-Generated Images
- Log in to post comments
Based on recent random matrix advances in the analysis of kernel methods for classification and clustering, this paper proposes the study of large kernel methods for a wide class of random inputs, i.e., concentrated data, which are more generic than Gaussian mixtures. The concentration assumption is motivated by the fact that one can use generative models to design complex data structures, through Lipschitz-ally transformed concentrated vectors (e.g., Gaussian) which remain concentrated vectors.
- Categories:
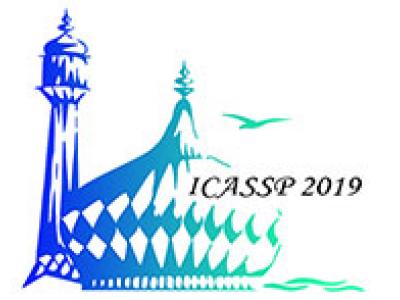
- Read more about [Poster] Local Convergence of the Heavy Ball method in Iterative Hard Thresholding for Low-Rank Matrix Completion
- Log in to post comments
We present a momentum-based accelerated iterative hard thresholding (IHT) for low-rank matrix completion. We analyze the convergence of the proposed Heavy Ball (HB) accelerated IHT near the solution and provide optimal step size parameters that guarantee the fastest rate of convergence. Since the optimal step sizes depend on the unknown structure of the solution matrix, we further propose a heuristic for parameter selection that is inspired by recent results in random matrix theory.
- Categories:
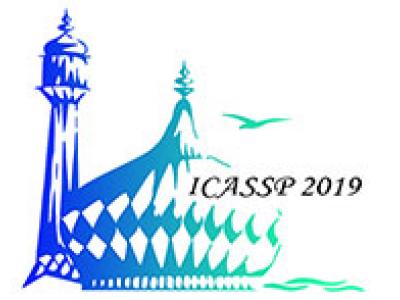
- Read more about Gradient Image Super-Resolution for Low-Resolution Image Recognition
- Log in to post comments
In visual object recognition problems essential to surveillance and navigation problems in a variety of military and civilian use cases,low-resolution and low-quality images present great challenges to this problem. Recent advancements in deep learning based methods like EDSR/VDSR have boosted pixel domain image super-resolution(SR) performances significantly in terms of signal to noise ratio(SNR)/mean square error(MSE) metrics of the super-resolved image.
- Categories:
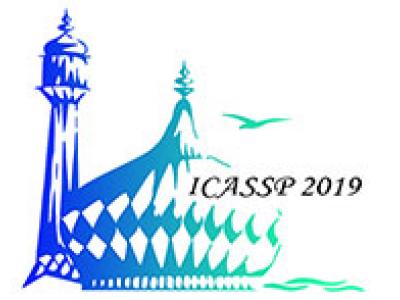
- Read more about Speech Denoising by Parametric Resynthesis
- Log in to post comments
This work proposes the use of clean speech vocoder parameters
as the target for a neural network performing speech enhancement.
These parameters have been designed for text-to-speech
synthesis so that they both produce high-quality resyntheses
and also are straightforward to model with neural networks,
but have not been utilized in speech enhancement until now.
In comparison to a matched text-to-speech system that is given
the ground truth transcripts of the noisy speech, our model is
poster.pdf

- Categories:
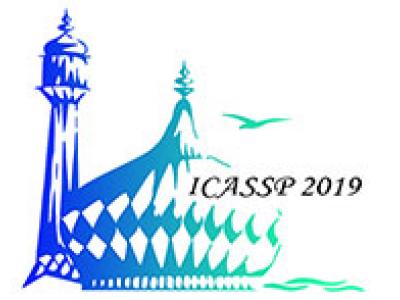
- Read more about Stochatic Adaptive Neural Architecture Search
- Log in to post comments
- Categories:
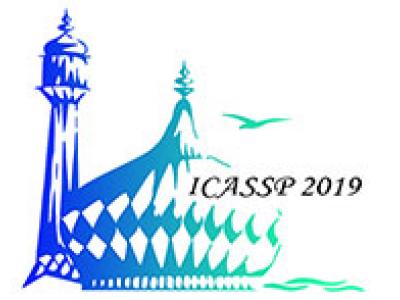
- Read more about Intonation: a Dataset of Quality Vocal Performances Refined by Spectral Clustering on Pitch Congruence
- Log in to post comments
We introduce the "Intonation" dataset of amateur vocal performances with a tendency for good intonation, collected from Smule, Inc. The dataset can be used for music information retrieval tasks such as autotuning, query by humming, and singing style analysis. It is available upon request on the Stanford CCRMA DAMP website. We describe a semi-supervised approach to selecting the audio recordings from a larger collection of performances based on intonation patterns.
- Categories:
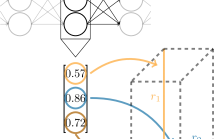
- Read more about Tropical Modeling of Weighted Transducer Algorithms on Graphs
- Log in to post comments
- Categories: