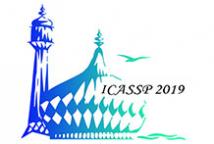
ICASSP is the world’s largest and most comprehensive technical conference focused on signal processing and its applications. The 2019 conference will feature world-class presentations by internationally renowned speakers, cutting-edge session topics and provide a fantastic opportunity to network with like-minded professionals from around the world. Visit website.
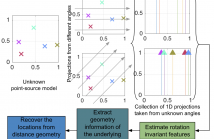
In this paper, we study a 2D tomography problem for point source models with random unknown view angles. Rather than recovering the projection angles, we reconstruct the model through a set of rotation-invariant features that are estimated from the projection data. For a point source model, we show that these features reveal geometric information about the model such as the radial and pairwise distances. This establishes a connection between unknown view tomography and unassigned distance geometry problem (uDGP).
- Categories:
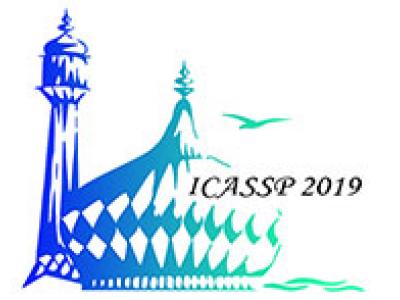
- Read more about SOLVING MEMORY ACCESS CONFLICTS IN LTE-4G STANDARD
- Log in to post comments
- Categories:
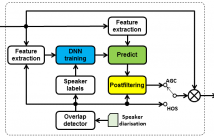
The CHiME-5 speech separation and recognition challenge was recently shown to pose a difficult task for the current automatic speech recognition systems.
Speaker overlap was one of the main difficulties of the challenge. The presence of noise, reverberation and the moving speakers have made the traditional source separation methods ineffective in improving the recognition accuracy.
In this paper we have explored several enhancement strategies aimed to reduce the effect of speaker overlap for CHiME-5 without performing source separation.
- Categories:
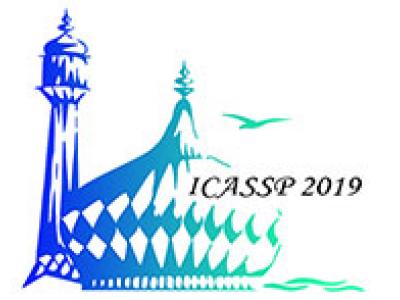
- Read more about Detectability of Denial-of-Service Attacks on Communication Systems
- Log in to post comments
Wireless communication systems are inherently vulnerable to adversarial attacks since malevolent jammers might jam and disrupt the legitimate transmission intentionally. Accordingly it is of crucial interest for the legitimate users to detect such adversarial attacks. This paper develops a detection framework based on Turing machines and studies the detectability of adversarial attacks. Of particular interest are so-called denial-of-service attacks in which the jammer is able to completely prevent any transmission.
- Categories:
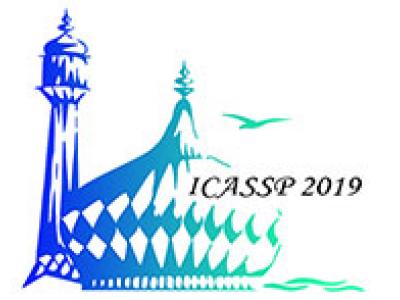
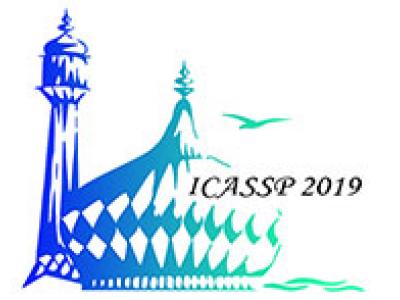
- Read more about Optimal Feature Selection for Blind Super-Resolution Image Quality Evaluation
- Log in to post comments
The visual quality of images resulting from Super Resolution (SR) techniques is predicted with blind image quality assessment (BIQA) models trained on a database(s) of human rated distorted images and associated human subjective opinion scores. Such opinion-aware (OA) methods need a large amount of training samples with associated human subjective scores, which are scarce in the field of SR. By contrast, opinion distortion unaware (ODU) methods do not need human subjective scores for training.
- Categories:
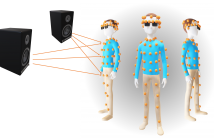
We present an open-access dataset of over 8000 acoustic impulse from 160 microphones spread across the body and affixed to wearable accessories. The data can be used to evaluate audio capture and array processing systems using wearable devices such as hearing aids, headphones, eyeglasses, jewelry, and clothing. We analyze the acoustic transfer functions
- Categories:
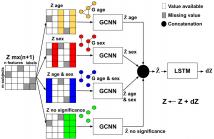
- Read more about Multiple-Graph Recurrent Graph Convolutional Neural Network architectures for predicting disease outcomes
- Log in to post comments
Improving disease outcome prediction can greatly aid in the strategic deployment of secondary prevention approaches. We develop a method to predict the evolution of diseases by taking into account personal attributes of the subjects and their relationships with medical examination results. Our approach builds upon a recent formulation of this problem as a graph-based geometric matrix completion task. The primary innovation is the introduction of multiple graphs, each relying on a different combination of subject attributes.
- Categories:
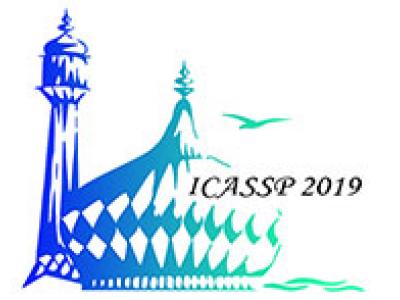
- Read more about ONLINE ESTIMATION AND SMOOTHING OF A TARGET TRAJECTORY IN MIXED STATIONARY/MOVING CONDITIONS
- Log in to post comments
A novel maximum likelihood trajectory estimation algorithm for targets in mixed stationary/moving conditions is presented. The proposed approach is able to estimate position and velocity of the target over arbitrary complex trajectories, while explicitly taking into account the possibility of stop&go motion. Moreover, a novel trajectory reconstruction method based on the theory of Bezier curve is developed for online smoothing of the trajectory, which keeps the advantages of Bayesian smoothing while introducing only a fixed lag in the estimation process.
poster.pdf

- Categories:
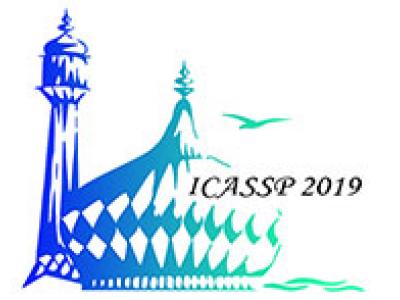
- Read more about Scene Privacy Protection
- Log in to post comments
Uploading pictures to a cloud service may reveal, through automatic inference, scene information that a user might want to keep private. To protect images from automatic scene classification, we present a method that misleads the classifier while introducing only a minimal distortion and limiting the likelihood that the ground-truth class can be inferred from the processed image. The method, based on the Fast Gradient Sign Method (FGSM), generates adversarial images and leverages a multi-class scene classifier trained to select a target scene class.
- Categories: