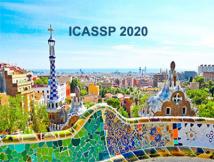
ICASSP is the world’s largest and most comprehensive technical conference focused on signal processing and its applications. The ICASSP 2020 conference will feature world-class presentations by internationally renowned speakers, cutting-edge session topics and provide a fantastic opportunity to network with like-minded professionals from around the world. Visit website.
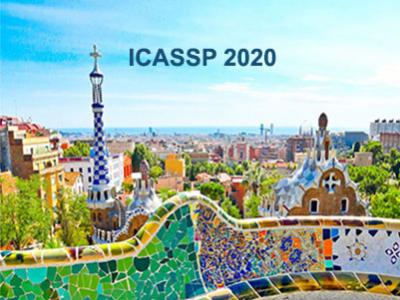
- Read more about MULTI-HEAD ATTENTION FOR SPEECH EMOTION RECOGNITION WITH AUXILIARY LEARNING OF GENDER RECOGNITION
- Log in to post comments
The paper presents a Multi-Head Attention deep learning network for Speech Emotion Recognition (SER) using Log mel-Filter Bank Energies (LFBE) spectral features as the input. The multi-head attention along with the position embedding jointly attends to information from different representations of the same LFBE input sequence. The position embedding helps in attending to the dominant emotion features by identifying positions of the features in the sequence. In addition to Multi-Head Attention and position embedding, we apply multi-task learning with gender recognition as an auxiliary task.
ICASSP.pdf

- Categories:
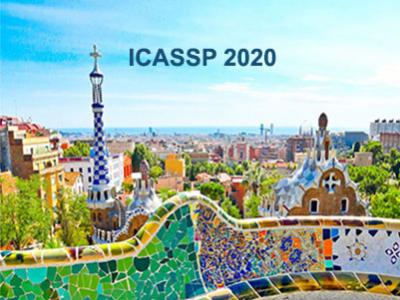
- Read more about Combining cGAN and MIL for Hotspot Segmentation in Bone Scintigraphy
- Log in to post comments
- Categories:
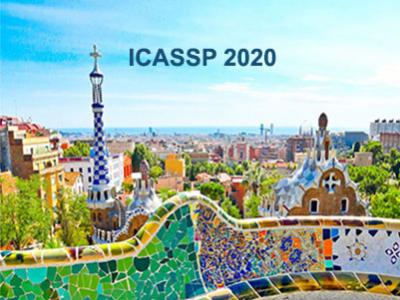
- Read more about Q-GADMM: Quantized Group ADMM for Communication Efficient Decentralized Machine Learning
- Log in to post comments
In this paper, we propose a communication-efficient decentralized machine learning (ML) algorithm, coined quantized group ADMM (Q-GADMM). Every worker in Q-GADMM communicates only with two neighbors, and updates its model via the group alternating direct method of multiplier (GADMM), thereby ensuring fast convergence while reducing the number of communication rounds. Furthermore, each worker quantizes its model updates before transmissions, thereby decreasing the communication payload sizes.
- Categories:
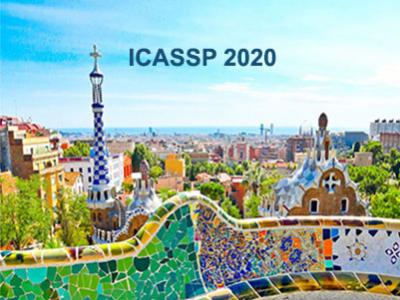
- Read more about Optimizing Bayesian HMM Based x-vector Clustering for theSecond DIHARD Speech Diarization Challenge
- Log in to post comments
- Categories:
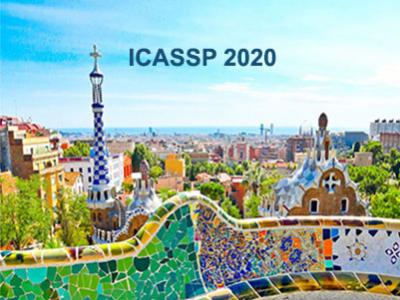
- Read more about Detecting Mismatch between Text Script and Voice-over Using Utterance Verification Based on Phoneme Recognition Ranking
- Log in to post comments
The purpose of this study is to detect the mismatch between text script and voice-over. For this, we present a novel utterance verification (UV) method, which calculates the degree of correspondence between a voice-over and the phoneme sequence of a script. We found that the phoneme recognition probabilities of exaggerated voice-overs decrease compared to ordinary utterances, but their rankings do not demonstrate any significant change.
- Categories:
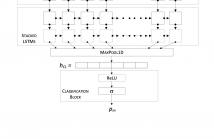
- Read more about Detection of Malicious VBScript Using Static and Dynamic Analysis with Recurrent Deep Learning
- Log in to post comments
- Categories:
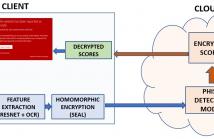
- Read more about Privacy-Preserving Phishing Web Page Classification Via Fully Homomorphic Encryption
- Log in to post comments
- Categories:
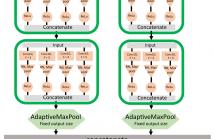
- Read more about 'TEXCEPTION: A Character/Word-Level Deep Learning Model for Phishing URL Detection
- Log in to post comments
- Categories:
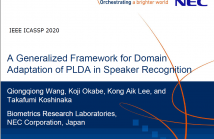
- Read more about A Generalized Framework for Domain Adaptation of PLDA in Speaker Recognition
- Log in to post comments
This paper proposes a generalized framework for domain adaptation of Probabilistic Linear Discriminant Analysis (PLDA) in speaker recognition. It not only includes several existing supervised and unsupervised domain adaptation methods but also makes possible more flexible usage of available data in different domains. In particular, we introduce here the two new techniques described below. (1) Correlation-alignment-based interpolation and (2) covariance regularization.
- Categories:
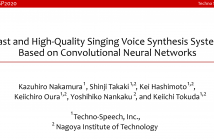
- Read more about Fast and High-Quality Singing Voice Synthesis System based on Convolutional Neural Networks
- Log in to post comments
The present paper describes singing voice synthesis based on convolutional neural networks (CNNs). Singing voice synthesis systems based on deep neural networks (DNNs) are currently being proposed and are improving the naturalness of synthesized singing voices. As singing voices represent a rich form of expression, a powerful technique to model them accurately is required. In the proposed technique, long-term dependencies of singing voices are modeled by CNNs.
- Categories: