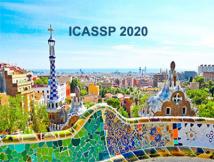
ICASSP is the world’s largest and most comprehensive technical conference focused on signal processing and its applications. The ICASSP 2020 conference will feature world-class presentations by internationally renowned speakers, cutting-edge session topics and provide a fantastic opportunity to network with like-minded professionals from around the world. Visit website.
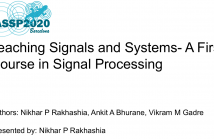
- Read more about Teaching Signals and Systems - A First Course in Signal Processing
- Log in to post comments
Signals and systems is a well known fundamental course in signal processing. How this course is taught to a student can spell the difference between whether s/he pursues a career in this field or not. Giving due consideration to this matter, this paper reflects on the experiences in teaching this course. In addition, the authors share the experiences of creating and conducting a Massive Open Online Course (MOOC) on this subject under edX and subsequently following it up with deliberation among some students who did this course through the platform.
- Categories:
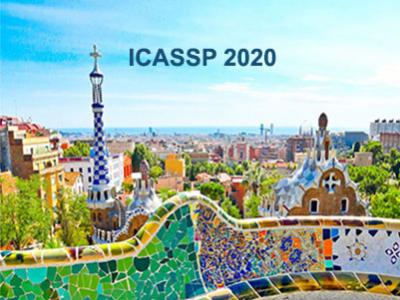
- Read more about JOINT ENHANCEMENT AND DENOISING OF LOW LIGHT IMAGES VIA JND TRANSFORM
- Log in to post comments
Low light images suffer from low dynamic range and severe noise due to low signal-to-noise ratio (SNR). In this paper, we propose joint enhancement and denoising of low light images via justnoticeable-difference (JND) transform. We achieve contrast enhancement and noise reduction simultaneously based on human visual perception. First, we perform contrast enhancement based on perceptual histogram to effectively allocate a dynamic range while preventing over-enhancement. Second, we generate JND map based on an HVS response model from foreground and background luminance, called JND transform.
- Categories:
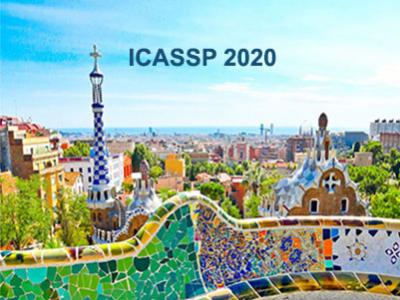
- Read more about Multi-Layer Content Interaction Through Quaternion Product for Visual Question Answering
- Log in to post comments
Multi-modality fusion technologies have greatly improved the performance of neural network-based Video Description/Caption, Visual Question Answering (VQA) and Audio Visual Scene-aware Di-alog (AVSD) over the recent years. Most previous approaches only explore the last layers of multiple layer feature fusion while omit-ting the importance of intermediate layers. To solve the issue for the intermediate layers, we propose an efficient Quaternion Block Net-work (QBN) to learn interaction not only for the last layer but also for all intermediate layers simultaneously.
- Categories:
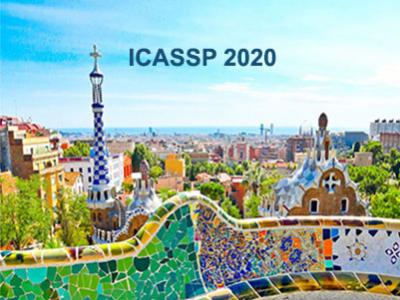
- Read more about WHAT MAKES THE SOUND?: A DUAL-MODALITY INTERACTING NETWORK FOR AUDIO-VISUAL EVENT LOCALIZATION
- Log in to post comments
The presence of auditory and visual senses enables humans to obtain a profound understanding of the real-world scenes. While audio and visual signals are capable of providing scene knowledge individually, the combination of both offers a better insight about the underlying event. In this paper, we address the problem of audio-visual event localization where the goal is to identify the presence of an event that is both audible and visible in a video, using fully or weakly supervised learning.
- Categories:
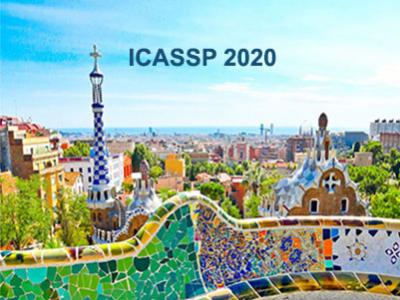
- Read more about Expression Guided EEG Representation Learning for Emotion Recognition
- Log in to post comments
Learning a joint and coordinated representation between different modalities can improve multimodal emotion recognition. In this paper, we propose a deep representation learning approach for emotion recognition from electroencephalogram (EEG) signals guided by facial electromyogram (EMG) and electrooculogram (EOG) signals. We recorded EEG, EMG and EOG signals from 60 participants who watched 40 short videos and self-reported their emotions.
- Categories:
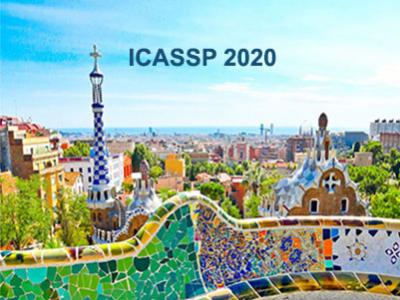
- Read more about Multi-Patch Aggregation Models for Resampling Detection
- 1 comment
- Log in to post comments
Images captured nowadays are of varying dimensions with smartphones and DSLR’s allowing users to choose from a list of available image resolutions. It is therefore imperative for forensic algorithms such as resampling detection to scale well for images of varying dimensions. However, in our experiments we observed that many state-of-the-art forensic algorithms are sensitive to image size and their performance quickly degenerates when operated on images of diverse dimensions despite re-training them using multiple image sizes.
- Categories:
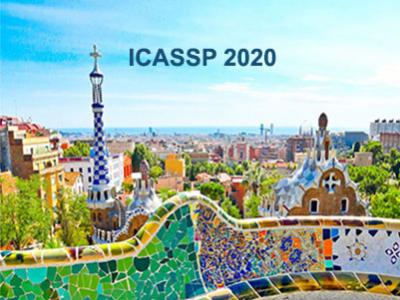
- Read more about COMBINING DEEP EMBEDDINGS OF ACOUSTIC AND ARTICULATORY FEATURES FOR SPEAKER IDENTIFICATION
- Log in to post comments
In this study, deep embedding of acoustic and articulatory features are combined for speaker identification. First, a convolutional neural network (CNN)-based universal background model (UBM) is constructed to generate acoustic feature (AC) embedding. In addition, as the articulatory features (AFs) represent some important phonological properties during speech production, a multilayer perceptron (MLP)-based AF embedding extraction model is also constructed for AF embedding extraction.
- Categories:
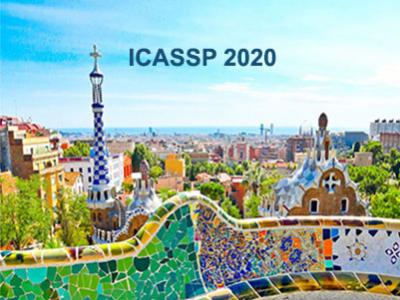
- Read more about Statistics Pooling Time Delay Neural Network Based on X-vector for Speaker Verification
- Log in to post comments
This paper aims to improve speaker embedding representation based on x-vector for extracting more detailed information for speaker verification. We propose a statistics pooling time delay neural network (TDNN), in which the TDNN structure integrates statistics pooling for each layer, to consider the variation of temporal context in frame-level transformation. The proposed feature vector, named as stats-vector, are compared with the baseline x-vector features on the VoxCeleb dataset and the Speakers in the Wild (SITW) dataset for speaker verification.
- Categories:
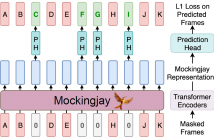
- Read more about Mockingjay: Unsupervised Speech Representation Learning with Deep Bidirectional Transformer Encoders
- Log in to post comments
We present Mockingjay as a new speech representation learning approach, where bidirectional Transformer encoders are pre-trained on a large amount of unlabeled speech. Previous speech representation methods learn through conditioning on past frames and predicting information about future frames. Whereas Mockingjay is designed to predict the current frame through jointly conditioning on both past and future contexts.
- Categories:
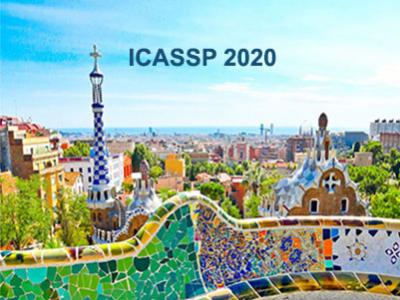
- Read more about A NOVEL RANK SELECTION SCHEME IN TENSOR RING DECOMPOSITION BASED ON REINFORCEMENT LEARNING FOR DEEP NEURAL NETWORKS
- Log in to post comments
Tensor decomposition has been proved to be effective for solving many problems in signal processing and machine learning. Recently, tensor decomposition finds its advantage for compressing deep neural networks. In many applications of deep neural networks, it is critical to reduce the number of parameters and computation workload to accelerate inference speed in deployment of the network. Modern deep neural network consists of multiple layers with multi-array weights where tensor decomposition is a natural way to perform compression.
- Categories: