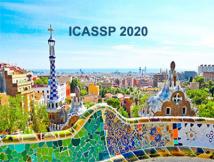
ICASSP is the world’s largest and most comprehensive technical conference focused on signal processing and its applications. The ICASSP 2020 conference will feature world-class presentations by internationally renowned speakers, cutting-edge session topics and provide a fantastic opportunity to network with like-minded professionals from around the world. Visit website.
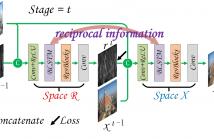
Single image deraining has been widely studied in recent years. Motivated by residual learning, most deep learning based deraining approaches devote research attention to extracting rain streaks, usually yielding visual artifacts in final deraining images. To address this issue, we in this paper propose bilateral recurrent network (BRN) to simultaneously exploit rain streak layer and background image layer. Generally, we employ dual residual networks (ResNet) that are recursively unfolded to sequentially extract rain streaks and predict clean background image.
BRN_slides.pdf

- Categories:
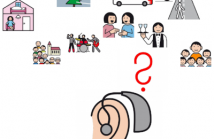
- Read more about Hearing Aid Research Data Set for Acoustic Environment Recognition (HEAR-DS)
- Log in to post comments
State-of-the-art hearing aids (HA) are limited in recognizing acoustic environments. Much effort is spent on research to improve listening experience for HA users in every acoustic situation. There is, however, no dedicated public database to train acoustic environment recognition algorithms with a specific focus on HA applications accounting for their requirements. Existing acoustic scene classification databases are inappropriate for HA signal processing.
- Categories:
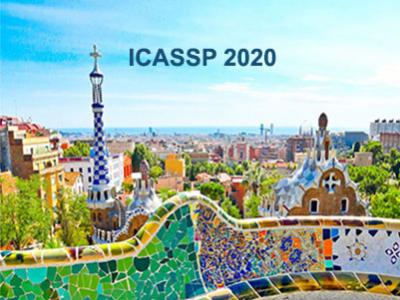
- Read more about A COMPARATIVE STUDY OF ESTIMATING ARTICULATORY MOVEMENTS FROM PHONEME SEQUENCES AND ACOUSTIC FEATURES
- Log in to post comments
Unlike phoneme sequences, movements of speech articulators (lips, tongue, jaw, velum) and the resultant acoustic signal are known to encode not only the linguistic message but also carry para-linguistic information. While several works exist for estimating articulatory movement from acoustic signals, little is known to what extent articulatory movements can be predicted only from linguistic information, i.e., phoneme sequence.
- Categories:
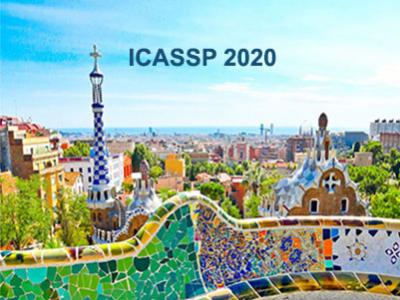
- Read more about SPOKEN LANGUAGE ACQUISITION BASED ON REINFORCEMENT LEARNING AND WORD UNIT SEGMENTATION
- Log in to post comments
The process of spoken language acquisition has been one of the topics which attract the greatest interesting from linguists for decades. By utilizing modern machine learning techniques, we simulated this process on computers, which helps to understand the mystery behind the process, and enable new possibilities of applying this concept on, but not limited to, intelligent robots. This paper proposes a new framework for simulating spoken language acquisition by combining reinforcement learning and unsupervised learning methods.
- Categories:
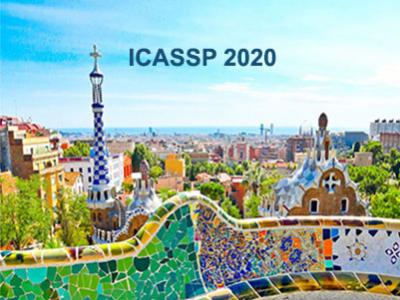
- Read more about Learning to rank music tracks using triplet loss
- Log in to post comments
Most music streaming services rely on automatic recommendation algorithms to exploit their large music catalogs. These algorithms aim at retrieving a ranked list of music tracks based on their similarity with a target music track. In this work, we propose a method for direct recommendation based on the audio content without explicitly tagging the music tracks. To that aim, we propose several strategies to perform triplet mining from ranked lists. We train a Convolutional Neural Network to learn the similarity via triplet loss.
icassp.pdf

- Categories:
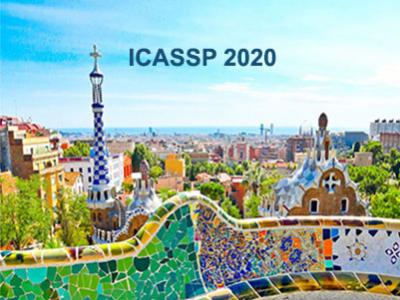
- Read more about Weighted Speech Distortion Losses for Real-time Speech Enhancement
- Log in to post comments
This paper investigates several aspects of training a RNN (recurrent neural network) that impact the objective and subjective quality of enhanced speech for real-time single-channel speech enhancement. Specifically, we focus on a RNN that enhances short-time speech spectra on a single-frame-in, single-frame-out basis, a framework adopted by most classical signal processing methods. We propose two novel mean-squared-error-based learning objectives that enable separate control over the importance of speech distortion versus noise reduction.
- Categories:
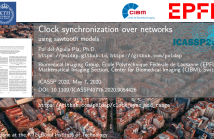
- Read more about Clock synchronization over networks using sawtooth models - Presentation
- Log in to post comments
Clock synchronization and ranging over a wireless network with low communication overhead is a challenging goal with tremendous impact. In this paper, we study the use of time-to-digital converters in wireless sensors, which provides clock synchronization and ranging at negligible communication overhead through a sawtooth signal model for round trip times between two nodes.
- Categories:
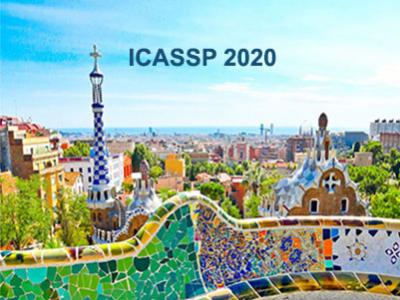
- Read more about Attention based Curiosity-driven Exploration in Deep Reinforcement Learning
- Log in to post comments
Reinforcement Learning enables to train an agent via interaction with the environment. However, in the majority of real-world scenarios, the extrinsic feedback is sparse or not sufficient, thus intrinsic reward formulations are needed to successfully train the agent. This work investigates and extends the paradigm of curiosity-driven exploration. First, a probabilistic approach is taken to exploit the advantages of the attention mechanism, which is successfully applied in other domains of Deep Learning.
- Categories:
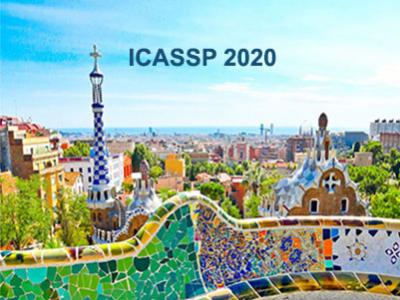
- Read more about Stabilizing Multi agent Deep Reinforcement Learning by Implicitly Estimating Other Agents’ Behaviors
- Log in to post comments
Deep reinforcement learning (DRL) is able to learn control policies for many complicated tasks, but it’s power has not been unleashed to handle multi-agent circumstances. Independent learning, where each agent treats others as part of the environment and learns its own policy without considering others’ policies is a simple way to apply DRL to multi-agent tasks. However, since agents’ policies change as learning proceeds, from the perspective of each agent, the environment is non-stationary, which makes conventional DRL methods inefficient.
- Categories: