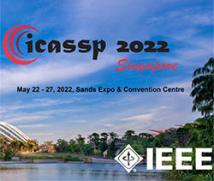
ICASSP 2022 - IEEE International Conference on Acoustics, Speech and Signal Processing is the world’s largest and most comprehensive technical conference focused on signal processing and its applications. The ICASSP 2022 conference will feature world-class presentations by internationally renowned speakers, cutting-edge session topics and provide a fantastic opportunity to network with like-minded professionals from around the world. Visit the website.
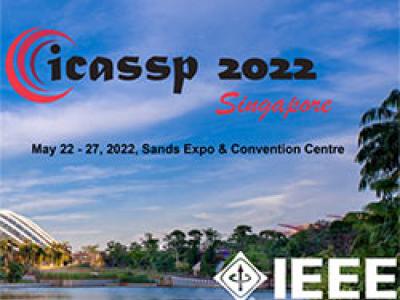
- Read more about Slides of Direct Noisy Speech Modeling for Noisy-to-Noisy Voice Conversion
- Log in to post comments
- Categories:
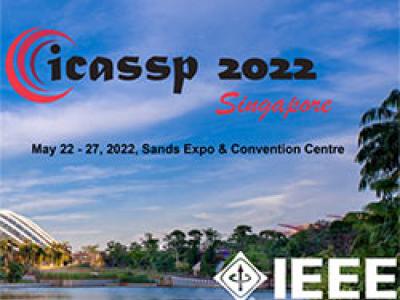
- Read more about Poster of Direct Noisy Speech Modeling for Noisy-to-Noisy Voice Conversion
- Log in to post comments
- Categories:
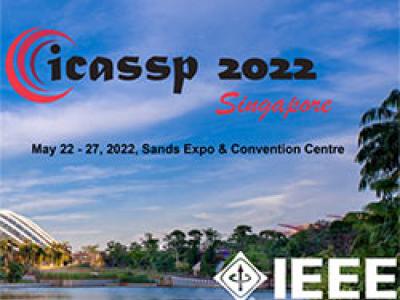
- Read more about A Few-sample Strategy for Guitar Tablature Transcription Based on Inharmonicity Analysis and Playability Constraints
- Log in to post comments
The prominent strategical approaches regarding the problem of guitar tablature transcription rely either on fingering patterns encoding or on the extraction of string-related audio features. The current work combines the two aforementioned strategies in an explicit manner by employing two discrete components for string-fret classification. It extends older few-sample modeling strategies by introducing various adaptation schemes for the first stage of audio processing, taking advantage of the inharmonic characteristics of guitar sound.
- Categories:
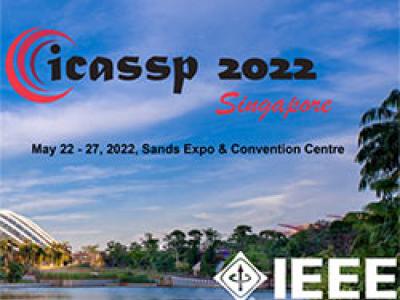
- Read more about MULTI-LINGUAL MULTI-TASK SPEECH EMOTION RECOGNITION USING WAV2VEC 2.0
- Log in to post comments
Speech Emotion Recognition (SER) has several use cases for
Digital Entertainment Content (DEC) in Over-the-top (OTT)
services, emotive Text-to-Speech (TTS) engines and voice
assistants. In this work, we present a Multi-Lingual (MLi) and
Multi-Task Learning (MTL) audio only SER system based on
the multi-lingual pre-trained wav2vec 2.0 model. The model
is fine-tuned on 25 open source datasets in 13 locales across
7 emotion categories. We show that, a) Our wav2vec 2.0
single task based model outperforms Pre-trained Audio Neural
- Categories:
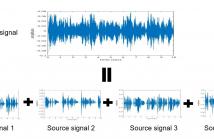
- Read more about HARVESTING PARTIALLY-DISJOINT TIME-FREQUENCY INFORMATION FOR IMPROVING DEGENERATE UNMIXING ESTIMATION TECHNIQUE
- Log in to post comments
The degenerate unmixing estimation technique (DUET) is one of the most efficient blind source separation algorithms tackling the challenging situation when the number of sources exceeds the number of microphones. However, as a time-frequency mask-based method, DUET erroneously results in interference components retention when source signals overlap each other in both frequency and time domains.
slides.pdf

- Categories:
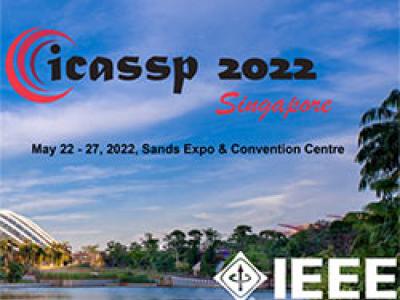
- Read more about Genre-conditioned Acoustic Models for Automatic Lyrics Transcription of Polyphonic Music
- Log in to post comments
Lyrics transcription of polyphonic music is challenging not only because the singing vocals are corrupted by the background music, but also because the background music and the singing style vary across music genres, such as pop, metal, and hip hop, which affects lyrics intelligibility of the song in different ways. In this work, we propose to transcribe the lyrics of polyphonic music using a novel genre-conditioned network.
- Categories:
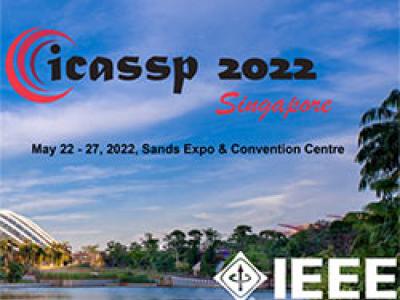
- Read more about TOWARDS JOINT FRAME-LEVEL AND MOS QUALITY PREDICTIONS WITH LOW-COMPLEXITY OBJECTIVE MODELS
- Log in to post comments
The evaluation of the quality of gaming content, with low-complexity and low-delay approaches is a major challenge raised by the emerging gaming video streaming and cloud-gaming services. Considering two existing and a newly created gaming databases this paper confirms that some low-complexity metrics match well with subjective scores when considering usual correlation indicators. It is however argued such a result is insufficient: gaming content suffers from sudden large quality drops that these indicators do not capture.
- Categories:
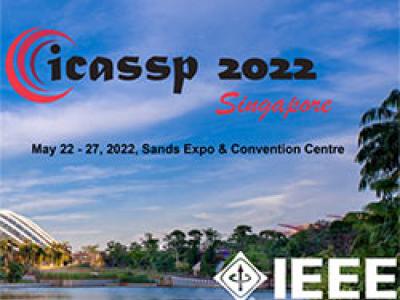
- Read more about SELF SUPERVISED REPRESENTATION LEARNING WITH DEEP CLUSTERING FOR ACOUSTIC UNIT DISCOVERY FROM RAW SPEECH
- Log in to post comments
The automatic discovery of acoustic sub-word units from raw speech, without any text or labels, is a growing field of research. The key challenge is to derive representations of speech that can be categorized into a small number of phoneme-like units which are speaker invariant and can broadly capture the content variability of speech. In this work, we propose a novel neural network paradigm that uses the deep clustering loss along with the autoregressive con- trastive predictive coding (CPC) loss. Both the loss functions, the CPC and the clustering loss, are self-supervised.
- Categories:
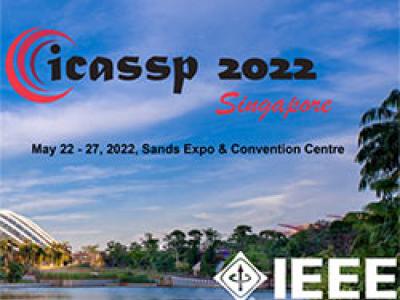
- Read more about Deep Markov Clustering For Panoptic Segmentation
- Log in to post comments
- Categories:
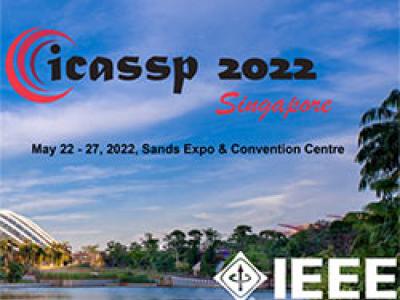
- Read more about LEARNING TO PREDICT SPEECH IN SILENT VIDEOS VIA AUDIOVISUAL ANALOGY
- Log in to post comments
- Categories: