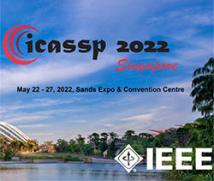
ICASSP 2022 - IEEE International Conference on Acoustics, Speech and Signal Processing is the world’s largest and most comprehensive technical conference focused on signal processing and its applications. The ICASSP 2022 conference will feature world-class presentations by internationally renowned speakers, cutting-edge session topics and provide a fantastic opportunity to network with like-minded professionals from around the world. Visit the website.
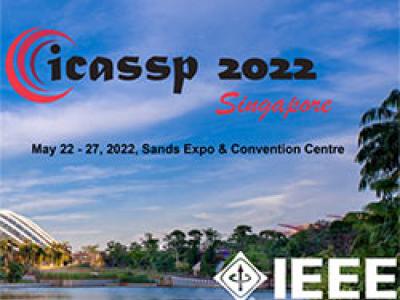
- Read more about THE SLIDE OF WEAKLY SUPERVISED POINT CLOUD UPSAMPLING VIA OPTIMAL TRANSPORT
- Log in to post comments
- Categories:
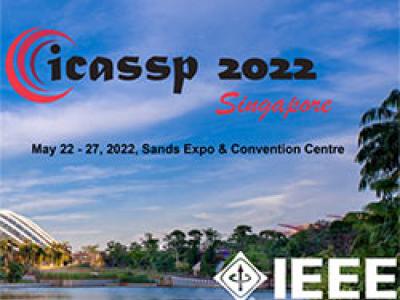
- Read more about TRACKING THE DIMENSIONS OF LATENT SPACES OF GAUSSIAN PROCESS LATENT VARIABLE MODELS
- Log in to post comments
- Categories:
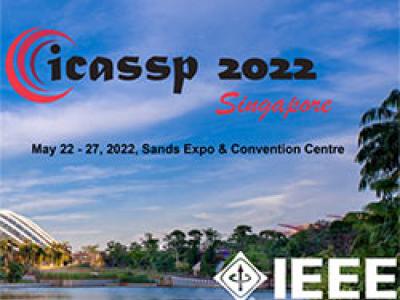
- Read more about Enhancing class understanding via prompt-tuning for zero-shot text classification
- Log in to post comments
- Categories:
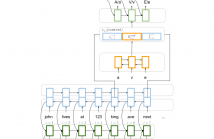
- Read more about CAPITALIZATION NORMALIZATION FOR LANGUAGE MODELING WITH AN ACCURATE AND EFFICIENT HIERARCHICAL RNN MODEL
- Log in to post comments
Capitalization normalization (truecasing) is the task of restoring the correct case (uppercase or lowercase) of noisy text. We propose a fast, accurate and compact two-level hierarchical word-and-character-based recurrent neural network model. We use the truecaser to normalize user-generated text in a Federated Learning framework for language modeling. A case-aware language model trained on this normalized text achieves the same perplexity as a model trained on text with gold capitalization.
- Categories:
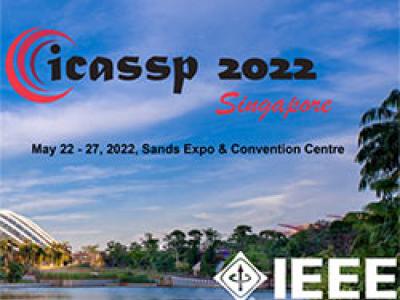
- Read more about Test-Time Detection of Backdoor Triggers for Poisoned Deep Neural Networks
- Log in to post comments
- Categories:
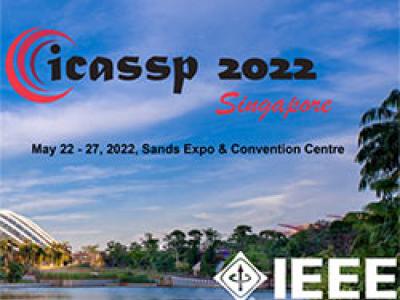
- Read more about Towards End-to-End Integration of Dialog History for Improved Spoken Language Understanding
- Log in to post comments
Dialog history plays an important role in spoken language understanding (SLU) performance in a dialog system. For end-to-end (E2E) SLU, previous work has used dialog history in text form, which makes the model dependent on a cascaded automatic speech recognizer (ASR). This rescinds the benefits of an E2E system which is intended to be compact and robust to ASR errors. In this paper, we propose a hierarchical conversation model that is capable of directly using dialog history in speech form, making it fully E2E.
- Categories:
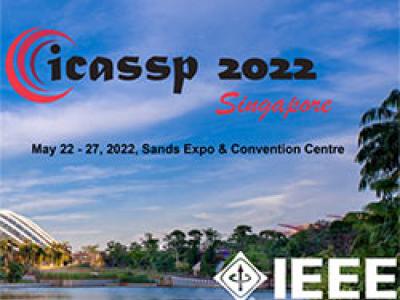
- Read more about Modeling Of Pre-trained Neural Network Embeddings Learned From Raw Waveform For Covid-19 Infection Detection
- Log in to post comments
COVID-19 is a respiratory system disorder that can disrupt the function of lungs. Effects of dysfunctional respiratory mechanism can reflect upon other modalities which function in close coupling. Audio signals result from modulation of respiration through speech production system, and hence acoustic information can be modeled for detection of COVID-19. In that direction, this paper is addressing the second DiCOVA challenge that deals with COVID-19 detection based on speech, cough and breathing.
- Categories:
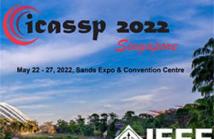
- Read more about Spell my name: keyword boosted speech recognition
- Log in to post comments
Recognition of uncommon words such as names and technical terminology is important to understanding conversations in context. However, the ability to recognise such words remains a challenge in modern automatic speech recognition (ASR) systems. In this paper, we propose a simple but powerful ASR decoding method that can better recognise these uncommon keywords, which in turn enables better readability of the results. The method boosts the probabilities of given keywords in a beam search based on acoustic model predictions. The method does not require any training in advance.
- Categories:
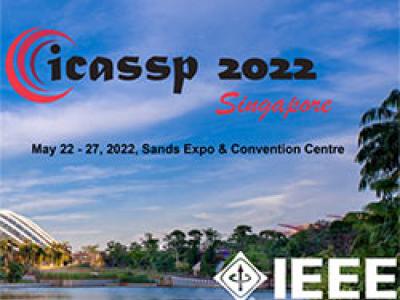
- Read more about Path signatures for non-intrusive load monitoring
- Log in to post comments
- Categories: