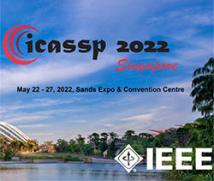
ICASSP 2022 - IEEE International Conference on Acoustics, Speech and Signal Processing is the world’s largest and most comprehensive technical conference focused on signal processing and its applications. The ICASSP 2022 conference will feature world-class presentations by internationally renowned speakers, cutting-edge session topics and provide a fantastic opportunity to network with like-minded professionals from around the world. Visit the website.
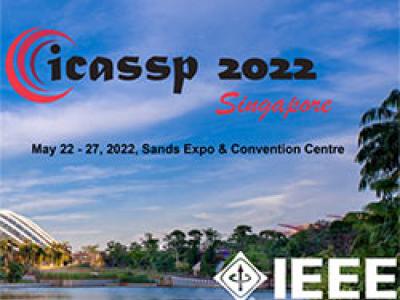
- Read more about [ICASSP 2022] NOT ALL FEATURES ARE EQUAL: SELECTION OF ROBUST FEATURES FOR SPEECH EMOTION RECOGNITION IN NOISY ENVIRONMENTS
- Log in to post comments
Speech emotion recognition (SER) system deployed in real-world applications often encounters noisy speech. While most noise compensation techniques consider all acoustic features to have equal impact on the SER model, some acoustic features may be more sensitive to noisy conditions. This paper investigates the noise robustness of each feature in the acoustic feature set. We focus on low-level descriptors (LLDs) commonly used in SER systems. We firstly train SER models with clean speech by only using a single LLD.
- Categories:
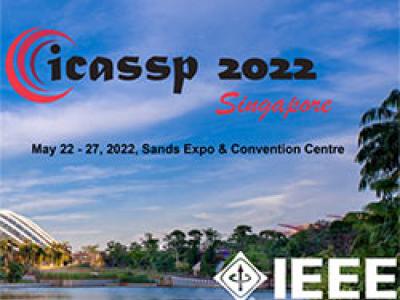
- Read more about Generalized autocorrelation analysis for multi-target detection
- Log in to post comments
We study the multi-target detection problem of recovering a target signal from a noisy measurement that contains multiple copies of the signal at unknown locations. Motivated by the structure reconstruction problem in cryo-electron microscopy, we focus on the high noise regime, where noise hampers accurate detection of signal occurrences. Previous works proposed an autocorrelation analysis framework to estimate the signal directly from the measurement, without detecting signal occurrences.
- Categories:
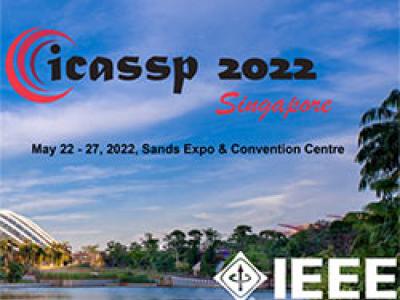
- Read more about Fast and Stable Convergence of Online SGD for CV@R-based Risk-Aware Statistical Learning
- Log in to post comments
- Categories:
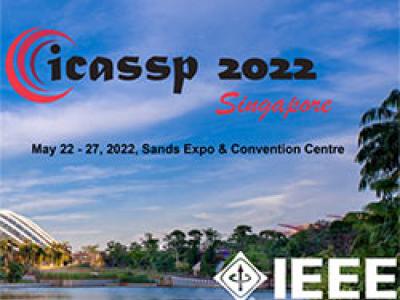
- Read more about Generalized autocorrelation analysis for multi-target detection
- Log in to post comments
We study the multi-target detection problem of recovering a target signal from a noisy measurement that contains multiple copies of the signal at unknown locations. Motivated by the structure reconstruction problem in cryo-electron microscopy, we focus on the high noise regime, where noise hampers accurate detection of signal occurrences. Previous works proposed an autocorrelation analysis framework to estimate the signal directly from the measurement, without detecting signal occurrences.
- Categories:
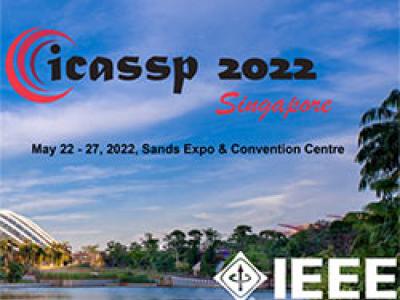
- Read more about WAV2VEC-SWITCH: CONTRASTIVE LEARNING FROM ORIGINAL-NOISY SPEECH PAIRS FOR ROBUST SPEECH RECOGNITION
- Log in to post comments
The goal of self-supervised learning (SSL) for automatic speech recognition (ASR) is to learn good speech representations from a large amount of unlabeled speech for the downstream ASR task. However, most SSL frameworks do not consider noise robustness which is crucial for real-world applications. In this paper we propose wav2vec-Switch, a method to encode noise robustness into contextualized representations of speech via contrastive learning. Specifically, we feed original-noisy speech pairs simultaneously into the wav2vec 2.0 network.
- Categories:
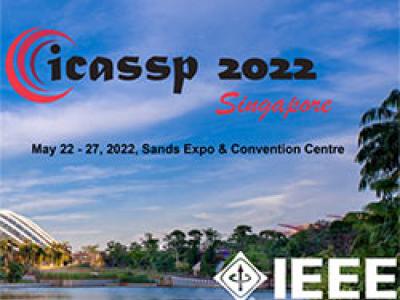
- Read more about Leveraging Local Temporal Information For Multimodal Scene Classification
- Log in to post comments
Robust video scene classification models should capture the spatial (pixel-wise) and temporal (frame-wise) characteristics of a video effectively. Transformer models with self-attention which are designed to get contextualized representations for individual tokens given a sequence of tokens, are becoming increasingly popular in many computer vision tasks. However, the use of Transformer based models for video under-standing is still relatively unexplored.
- Categories:
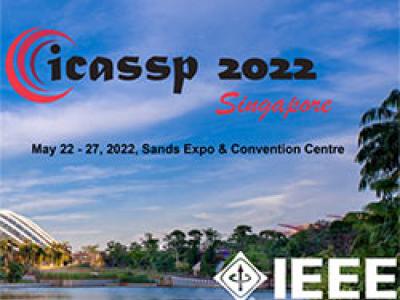
- Read more about DeepFilterNet: A Low Complexity Speech Enhancement Framework for Full-Band Audio based on Deep Filtering
- Log in to post comments
Complex-valued processing has brought deep learning-based speech enhancement and signal extraction to a new level. Typically, the process is based on a time-frequency (TF) mask which is applied to a noisy spectrogram, while complex masks (CM) are usually preferred over real-valued masks due to their ability to modify the phase. Recent work proposed to use a complex filter instead of a point-wise multiplication with a mask.
- Categories:
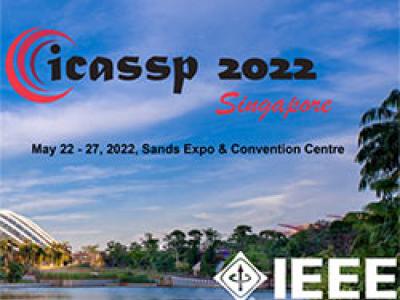
- Read more about SPARSE SUBSPACE TRACKING IN HIGH DIMENSIONS
- Log in to post comments
We studied the problem of sparse subspace tracking in the high-dimensional regime where the dimension is comparable to or much larger than the sample size. Leveraging power iteration and thresholding methods, a new provable algorithm called OPIT was derived for tracking the sparse principal subspace of data streams over time. We also presented a theoretical result on its convergence to verify its consistency in high dimensions. Several experiments were carried out on both synthetic and real data to demonstrate the effectiveness of OPIT.
- Categories: