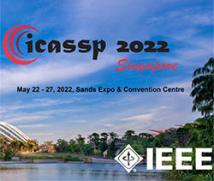
ICASSP 2022 - IEEE International Conference on Acoustics, Speech and Signal Processing is the world’s largest and most comprehensive technical conference focused on signal processing and its applications. The ICASSP 2022 conference will feature world-class presentations by internationally renowned speakers, cutting-edge session topics and provide a fantastic opportunity to network with like-minded professionals from around the world. Visit the website.
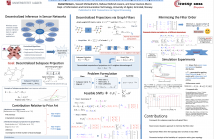
A number of inference problems with sensor networks involve projecting a
measured signal onto a given subspace. In existing decentralized
approaches, sensors communicate with their local neighbors to obtain a
sequence of iterates that asymptotically converges to the desired
projection. In contrast, the present paper develops methods that
produce these projections in a finite and approximately minimal number
of iterations. Building upon tools from graph signal processing, the
problem is cast as the design of a graph filter which, in turn, is
- Categories:
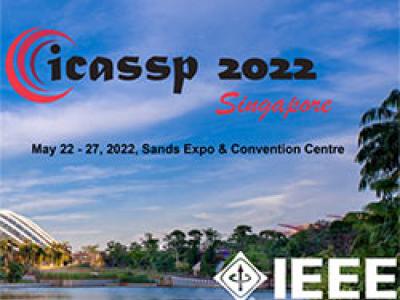
- Read more about HARMONICITY PLAYS A CRITICAL ROLE IN DNN BASED VERSUS IN BIOLOGICALLY-INSPIRED MONAURAL SPEECH SEGREGATION SYSTEMS
- Log in to post comments
Recent advancements in deep learning have led to drastic improvements in speech segregation models. Despite their success and growing applicability, few efforts have been made to analyze the underlying principles that these networks learn to perform segregation. Here we analyze the role of harmonicity on two state-of-the-art Deep Neural Networks (DNN)-based models- Conv-TasNet and DPT-Net. We evaluate their performance with mixtures of natural speech versus slightly manipulated inharmonic speech, where harmonics are slightly frequency jittered.
Parikh_poster.pdf

Parikh_CR.pdf

- Categories:
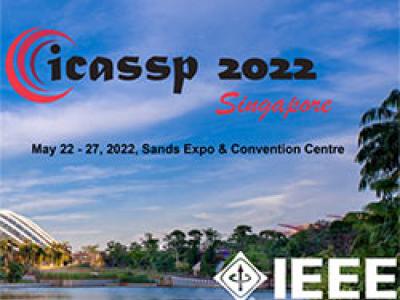
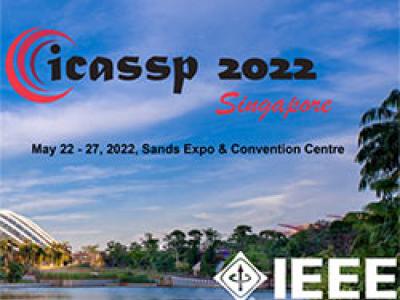
- Read more about Multimodal Depression Classification Using Articulatory Coordination Features and Hierarchical Attention Based Text Embeddings
- Log in to post comments
Multimodal depression classification has gained immense popularity over the recent years. We develop a multimodal depression classification system using articulatory coordination features extracted from vocal tract variables and text transcriptions obtained from an automatic speech recognition tool that yields improvements of area under the receiver operating characteristics curve compared to unimodal classifiers (7.5% and 13.7% for audio and text respectively).
3649_poster.pdf

- Categories:
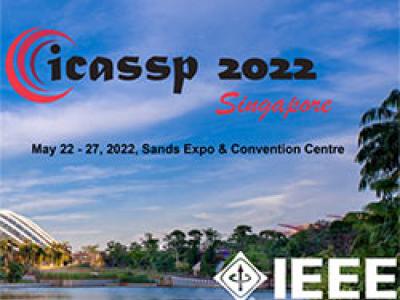
- Read more about Adversarial Learning in Transformer Based Neural Network in Radio signal classification
- Log in to post comments
- Categories:
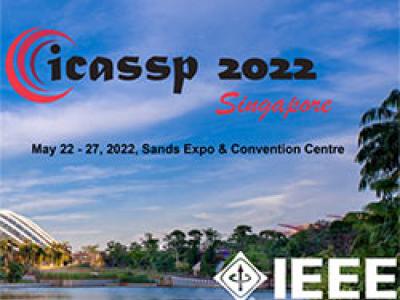
- Read more about AN EFFICIENT METHOD FOR GENERIC DSP IMPLEMENTATION OF DILATED CONVOLUTION
- 1 comment
- Log in to post comments
Dilated convolution is a well-known technique used in neural networks algorithms in AI/ML applications to increase receptive-field under analysis. Dilated convolution layer has an inherent property of capturing wider context in an image and long-term temporal characteristics in an audio signal. In this paper we propose a scheme that allows efficient/generic implementation of 2D dilated convolution and stride on typical DSPs where the instruction sets are well tuned for standard 1D and 2D filtering and convolution operations.
- Categories:
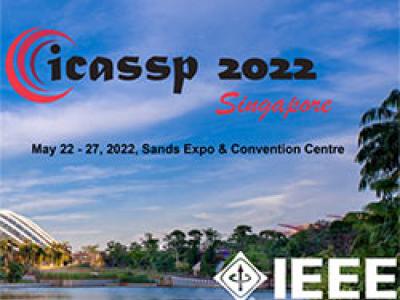
- Read more about Phonotactic Language Recognition using a Universal Phoneme Recognizer and a Transformer Architecture
- 1 comment
- Log in to post comments
In this paper, we describe a phonotactic language recognition model that effectively manages long and short n-gram input sequences to learn contextual phonotacticbased vector embeddings. Our approach uses a transformerbased encoder that integrates a sliding window attention to attempt finding discriminative short and long cooccurrences of language dependent n-gram phonetic units. We then evaluate and compare the use of different phoneme recognizers (Brno and Allosaurus) and sub-unit tokenizers to help select the more discriminative n-grams.
Poster.pdf

- Categories:
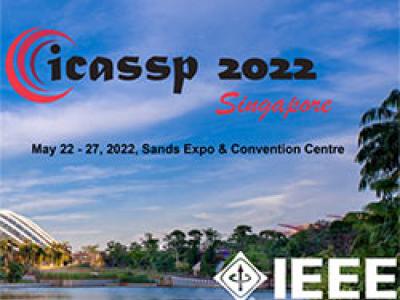
- Read more about On Identifiable Polytope Characterization for Polytopic Matrix Factorization
- Log in to post comments
Polytopic matrix factorization (PMF) is a recently introduced matrix decomposition method in which the data vectors are modeled as linear transformations of samples from a polytope. The successful recovery of the original factors in the generative PMF model is conditioned on the "identifiability" of the chosen polytope. In this article, we investigate the problem of determining the identifiability of a polytope. The identifiability condition requires the polytope to be permutation-and/or-sign-only invariant.
- Categories:
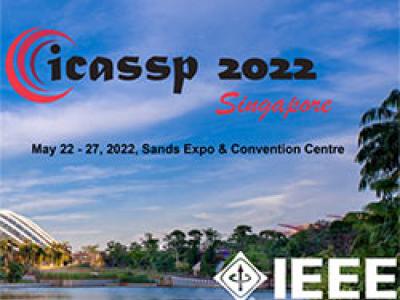
- Read more about Fast learning of fast transforms, with guarantees (ICASSP 2022 poster)
- Log in to post comments
Approximating a matrix by a product of few sparse factors whose supports possess the butterfly structure, which is common to many fast transforms, is key to learn fast transforms and speed up algorithms for inverse problems. We introduce a hierarchical approach that recursively factorizes the considered matrix into two factors. Using recent advances on the well-posedness and tractability of the two-factor fixed-support sparse matrix factorization problem, the proposed algorithm is endowed with exact recovery guarantees.
poster_v2.pdf

- Categories:
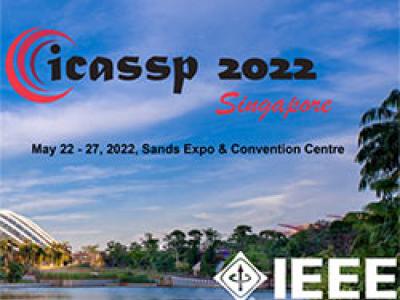
- Read more about THE POSTER OF WEAKLY SUPERVISED POINT CLOUD UPSAMPLING VIA OPTIMAL TRANSPORT
- Log in to post comments
poster.pdf

- Categories: