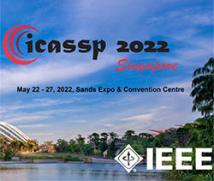
ICASSP 2022 - IEEE International Conference on Acoustics, Speech and Signal Processing is the world’s largest and most comprehensive technical conference focused on signal processing and its applications. The ICASSP 2022 conference will feature world-class presentations by internationally renowned speakers, cutting-edge session topics and provide a fantastic opportunity to network with like-minded professionals from around the world. Visit the website.
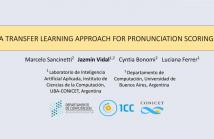
Phone-level pronunciation scoring is a challenging task, with performance far from that of human annotators. Standard systems generate a score for each phone in a phrase using models trained for automatic speech recognition (ASR) with native data only. Better performance has been shown when using systems that are trained specifically for the task using non-native data. Yet, such systems face the challenge that datasets labelled for this task are scarce and usually small.
2022-ICASSP (1).pdf

- Categories:
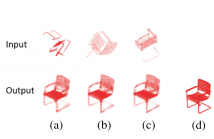
- Read more about CF-NET: COMPLEMENTARY FUSION NETWORK FOR ROTATION INVARIANT POINT CLOUD COMPLETION
- Log in to post comments
Real-world point clouds usually have inconsistent orientations and often suffer from data missing issues. To solve this problem, we design a neural network, CF-Net, to address challenges in rotation invariant completion. In our network, we modify and integrate complementary operators to extract features that are robust against rotation and incompleteness. Our CF-Net can achieve competitive results both geometrically and semantically as demonstrated in this paper.
- Categories:
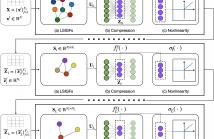
- Read more about Graph Convolutional Networks with Autoencoder-Based Compression and Multi-Layer Graph Learning
- Log in to post comments
The aim of this work is to propose a novel architecture and training strategy for graph convolutional networks (GCN). The proposed architecture, named as Autoencoder-Aided GCN (AA-GCN), compresses the convolutional features in an information-rich embedding at multiple hidden layers, exploiting the presence of autoencoders before the point-wise non-linearities. Then, we propose a novel end-to-end training procedure that learns different graph representations per each layer, jointly with the GCN weights and auto-encoder parameters.
- Categories:
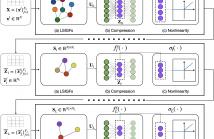
- Read more about Graph Convolutional Networks with Autoencoder-Based Compression and Multi-Layer Graph Learning
- Log in to post comments
The aim of this work is to propose a novel architecture and training strategy for graph convolutional networks (GCN). The proposed architecture, named as Autoencoder-Aided GCN (AA-GCN), compresses the convolutional features in an information-rich embedding at multiple hidden layers, exploiting the presence of autoencoders before the point-wise non-linearities. Then, we propose a novel end-to-end training procedure that learns different graph representations per each layer, jointly with the GCN weights and auto-encoder parameters.
- Categories:
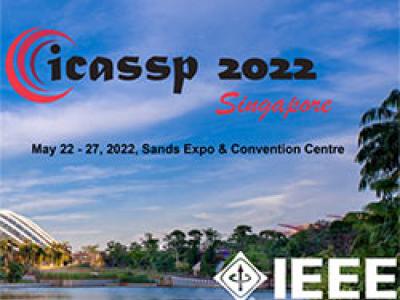
- Read more about SUPERVISED LEARNING BASED SPARSE CHANNEL ESTIMATION FOR RIS AIDED COMMUNICATIONS
- Log in to post comments
An reconfigurable intelligent surface (RIS) can be used to establish line-of-sight (LoS) communication when the direct path is compromised, which is a common occurrence in a millimeter wave (mmWave) network. In this paper, we focus on the uplink channel estimation of a such network. We formulate this as a sparse signal recovery problem, by discretizing the angle of arrivals (AoAs) at the base station (BS). On-grid and off-grid AoAs are considered separately. In the on-grid case, we propose an algorithm to estimate the direct and RIS channels.
- Categories:
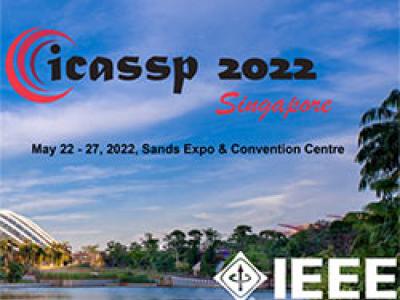
- Read more about Generative adversarial network including referring image segmentation for text-guided image manipulation
- Log in to post comments
This paper proposes a novel generative adversarial network to improve the performance of image manipulation using natural language descriptions that contain desired attributes. Text-guided image manipulation aims to semantically manipulate an image aligned with the text description while preserving text-irrelevant regions. To achieve this, we newly introduce referring image segmentation into the generative adversarial network for image manipulation. The referring image segmentation aims to generate a segmentation mask that extracts the text-relevant region.
- Categories:
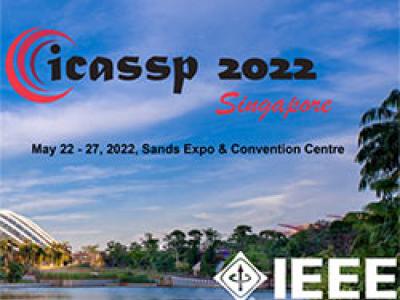
- Read more about ROBUST ADAPTIVE BEAMFORMING BASED ON POWER METHOD PROCESSING AND SPATIAL SPECTRUM MATCHING
- Log in to post comments
Robust adaptive beamforming (RAB) based on interference-plus noise covariance (INC) matrix reconstruction can experience performance degradation when model mismatch errors exist, particularly when the input signal-to-noise ratio (SNR) is large. In this work, we devise an efficient RAB technique for dealing with covariance matrix
- Categories:
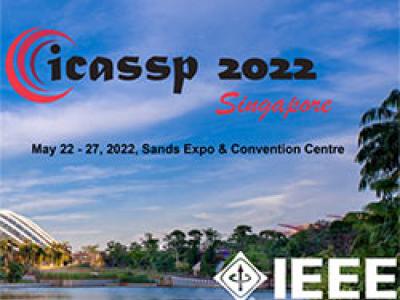
In this paper, a novel multi-head multi-layer perceptron (MLP) structure is presented for implicit neural representation (INR). Since conventional rectified linear unit (ReLU) networks are shown to exhibit spectral bias towards learning low-frequency features of the signal, we aim at mitigating this defect by taking advantage of local structure of the signals. To be more specific, an MLP is used to capture the global features of the underlying generator function of the desired signal.
- Categories:
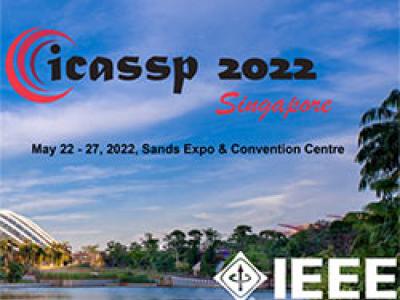
- Read more about Light-SERNet: A Lightweight Fully Convolutional Neural Network for Speech Emotion Recognition
- Log in to post comments
Detecting emotions directly from a speech signal plays an important role in effective human-computer interactions. Existing speech emotion recognition models require massive computational and storage resources, making them hard to implement concurrently with other machine-interactive tasks in embedded systems. In this paper, we propose an efficient and lightweight fully convolutional neural network (FCNN) for speech emotion recognition in systems with limited hardware resources.
- Categories: