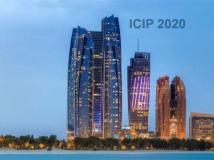
ICIP 2020 is a fully virtual conference. The International Conference on Image Processing (ICIP), sponsored by the IEEE Signal Processing Society, is the premier forum for the presentation of technological advances and research results in the fields of theoretical, experimental, and applied image and video processing. ICIP has been held annually since 1994, brings together leading engineers and scientists in image and video processing from around the world. Visit website.
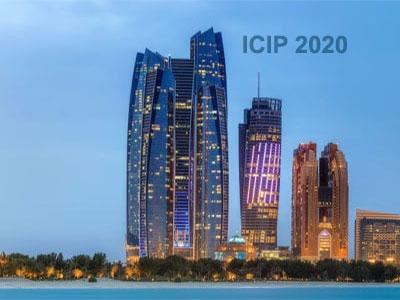
- Read more about An enhanced deep learning architecture for classification of tuberculosis types from CT lung images
- Log in to post comments
- Categories:
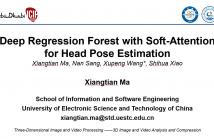
- Read more about Deep Regression Forest with Soft-Attention for Head Pose Estimation
- Log in to post comments
The task of head pose estimation from a single depth image is challenging, due to the presence of large pose variations, occlusions and inhomegeneous facial feature space. To solve the problem, we propose Deep Regression Forest with Soft-Attention (SA-DRF) in a multi-task learning setup. It can be integrated with a general feature learning net and jointly learned in an end-to-end manner. The soft-attention module is facilitated to learn soft masks from the general features and feeds the forest with task-specific features to regress head poses.
- Categories:
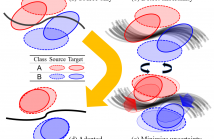
The key principle of unsupervised domain adaptation is to minimize the divergence between source and target domain. Many recent methods follow this principle to learn domain-invariant features. They train task-specific classifiers to maximize the divergence and feature extractors to minimize the divergence in an adversarial way. However, this strategy often limits their performance. In this paper, we present a novel method that learns feature representations that minimize the domain divergence. We show that model uncertainty is a useful surrogate for the domain divergence.
- Categories:
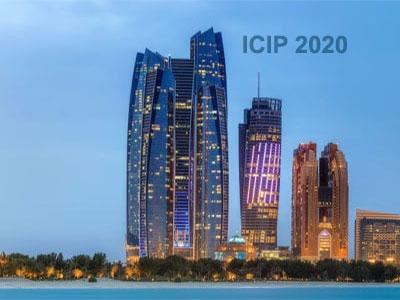
- Read more about RSANET: Deep Recurrent Scale-aware Network for Crowd Counting
- Log in to post comments
- Categories:
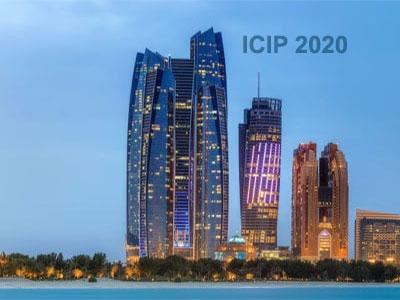
- Read more about Deep Learning Based Cross-Spectral Disparity Estimation for Stereo Imaging
- Log in to post comments
- Categories:
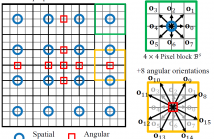
- Read more about A Spatio-Angular Binary Descriptor for Fast Light Field Inter View Matching
- Log in to post comments
Light fields are able to capture light rays from a scene arriving at different angles, effectively creating multiple perspective views of the same scene. Thus, one of the flagship applications of light fields is to estimate the captured scene geometry, which can notably be achieved by establishing correspondences between the perspective views, usually in the form of a disparity map. Such correspondence estimation has been a long standing research topic in computer vision, with application to stereo vision or optical flow.
- Categories:
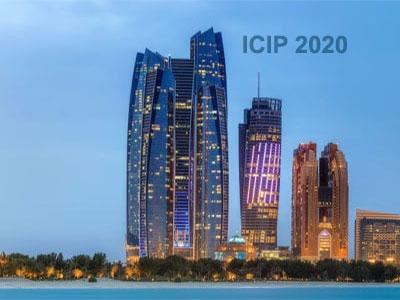
- Read more about MaskPan: mask prior guide pansharpening network
- Log in to post comments
- Categories:
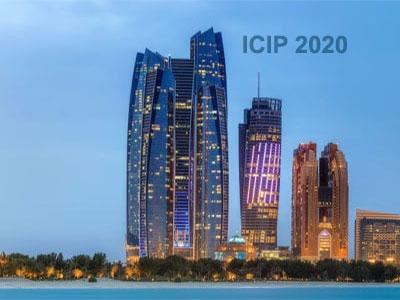
Segmentation of biological images is a challenging task, due to non convex shapes, intensity inhomogeneity and clustered cells. To address these issues, a new algorithm is proposed based on the B-spline level set method. The implicit function of the level set is modelled as a continuous parametric function represented with the B-spline basis. It is different from the discrete formulation associated with conventional level set. In this paper the proposed framework takes into account properties of biological images.
- Categories:
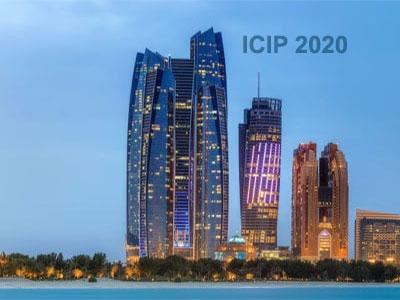
- Read more about MULTI-SCALE EXPLAINABLE FEATURE LEARNING FOR PATHOLOGICAL IMAGE ANALYSIS USING CONVOLUTIONAL NEURAL NETWORKS
- 1 comment
- Log in to post comments
- Categories: