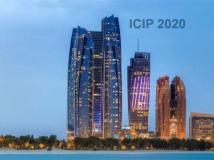
ICIP 2020 is a fully virtual conference. The International Conference on Image Processing (ICIP), sponsored by the IEEE Signal Processing Society, is the premier forum for the presentation of technological advances and research results in the fields of theoretical, experimental, and applied image and video processing. ICIP has been held annually since 1994, brings together leading engineers and scientists in image and video processing from around the world. Visit website.
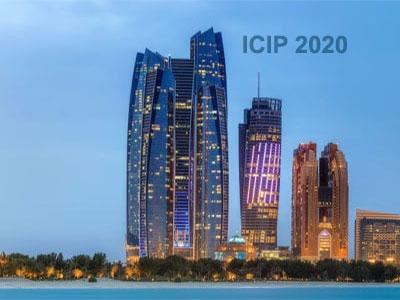
- Read more about GLAUCOMA DETECTION FROM RAW CIRCUMPAPILLARY OCT IMAGES USING FULLY CONVOLUTIONAL NEURAL NETWORKS
- Log in to post comments
- Categories:
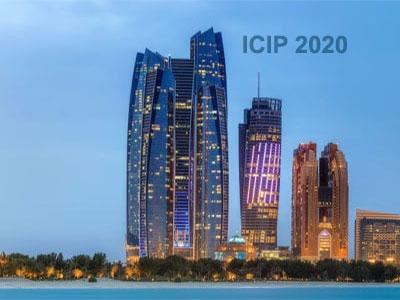
- Read more about RTip : A Fully Automated Root Tip Tracker for Measuring Plant Growth With Intermittent Perturbations
- Log in to post comments
RTip is a tool to quantify plant root growth velocity using high-resolution microscopy image sequences at sub-pixel accuracy. The fully automated RTip tracker is designed for high-throughput analysis of plant phenotyping experiments with episodic perturbations. RTip is able to auto-skip past these manual intervention perturbation activity, i.e. when the root tip is not under the microscope, the image is distorted or blurred. RTip provides the most accurate root growth velocity results with the lowest variance (i.e.
- Categories:
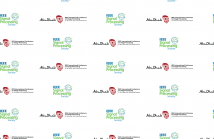
- Read more about ICIP2020-Slides
- Log in to post comments
Motions of facial components convey significant information of facial expressions. Although remarkable advancement has been made, the dynamic of facial topology has not been fully exploited. In this paper, a novel facial expression recognition (FER) algorithm called Spatial Temporal Semantic Graph Network (STSGN) is proposed to automatically learn spatial and temporal patterns through end-to-end feature learning from facial topology structure.
- Categories:
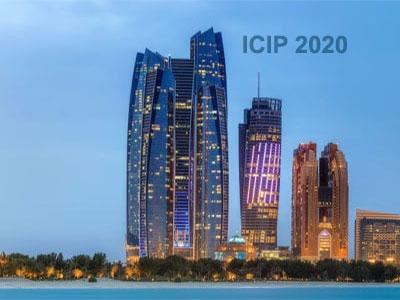
- Read more about Activity Normalization for Activity Detection in Surveillance Videos
- Log in to post comments
- Categories:
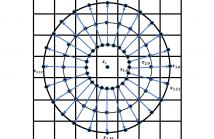
- Read more about AN ENHANCED LOCAL TEXTURE DESCRIPTOR FOR IMAGE SEGMENTATION
- 1 comment
- Log in to post comments
Texture is an indispensable property to develop many vision
based autonomous applications. Compared to colour, feature
dimension in a local texture descriptor is quite large as dense
texture features need to represent the distribution of pixel intensities
in the neighbourhood of each pixel. Large dimensional
features require additional time for further processing
that often restrict real-time applications. In this paper, a robust
local texture descriptor is enhanced by reducing feature
- Categories:
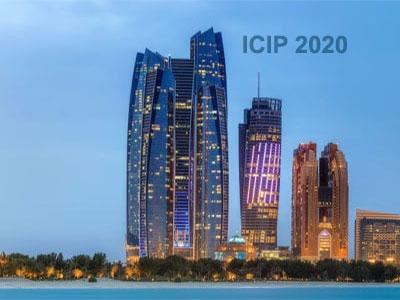
- Read more about ICIP 2020 presentation slides
- Log in to post comments
ICIP-ppt.pptx

- Categories:
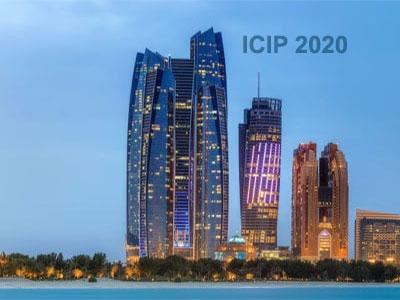
- Read more about Unconstrained Periocular Recognition: Using Generative Deep Learning Frameworks for Attribute Normalization
- Log in to post comments
Ocular biometric systems working in unconstrained environments usually face the problem of small within-class compactness caused by the multiple factors that jointly degrade the quality of the obtained data. In this work, we propose an attribute normalization strategy based on deep learning generative frameworks, that reduces the variability of the samples used in pairwise comparisons, without reducing their discriminability.
- Categories:
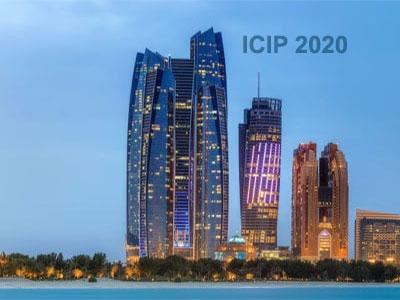
- Read more about Memory Assessment of Versatile Video Coding
- Log in to post comments
This work presents a memory assessment of the next-generation Versatile Video Coding (VVC). The memory analyses are performed adopting as a baseline the state-of-the-art High-Efficiency Video Coding (HEVC). The goal is to offer insights and observations of how critical the memory requirements of VVC are aggravated, compared to HEVC. The adopted methodology consists of two sets of experiments: (1) an overall memory profiling and (2) an inter-prediction specific memory analysis. The results obtained in the memory profiling show that VVC access up to 13.4x more memory than HEVC.
- Categories:
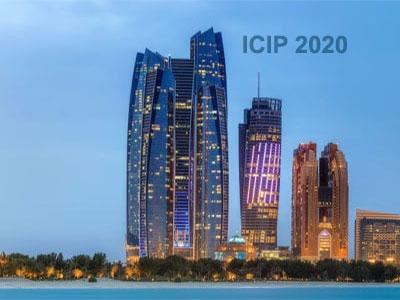
- Read more about Complexity Analysis of VVC Intra Prediction
- 1 comment
- Log in to post comments
- Categories:
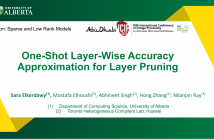
- Read more about One-Shot Layer-Wise Accuracy Approximation for Layer Pruning
- Log in to post comments
Recent advances in neural networks pruning have made it possible to remove a large number of filters without any perceptible drop in accuracy. However, the gain in speed depends on the number of filters per layer. In this paper, we propose a one-shot layer-wise proxy classifier to estimate layer importance that in turn allows us to prune a whole layer. In contrast to existing filter pruning methods which attempt to reduce the layer width of a dense model, our method reduces its depth and can thus guarantee inference speed up.
- Categories: