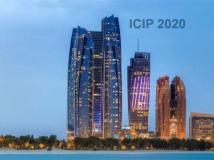
ICIP 2020 is a fully virtual conference. The International Conference on Image Processing (ICIP), sponsored by the IEEE Signal Processing Society, is the premier forum for the presentation of technological advances and research results in the fields of theoretical, experimental, and applied image and video processing. ICIP has been held annually since 1994, brings together leading engineers and scientists in image and video processing from around the world. Visit website.
Hyperspectral (HS) imaging retrieves information from data obtained across a wide spectral range of spectral channels. The object to reconstruct is a 3D cube, where two coordinates are spatial and the third one is spectral. We assume that this cube is complex-valued, i.e. characterized spatially frequency varying amplitude and phase. The observations are squared magnitudes measured as intensities summarized over the spectrum. The HS phase retrieval problem is formulated as a reconstruction of the HS complex-valued object cube from Gaussian noisy intensity observations.
- Categories:
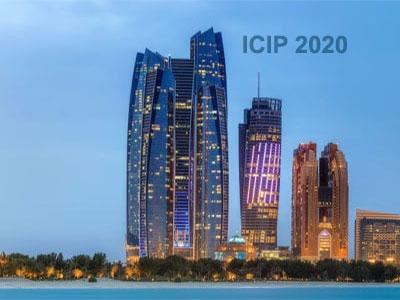
- Read more about RADAR+RGB Fusion for Robust Object Detection in Autonomous Vehicles
- Log in to post comments
This paper presents two variations of architecture referred to as RANet and BIRANet. The proposed architecture aims to use radar signal data along with RGB camera images to form a robust detection network that works efficiently, even in variable lighting and weather conditions such as rain, dust, fog, and others. First, radar information is fused in the feature extractor network. Second, radar points are used to generate guided anchors. Third, a method is proposed to improve region proposal network targets.
- Categories:
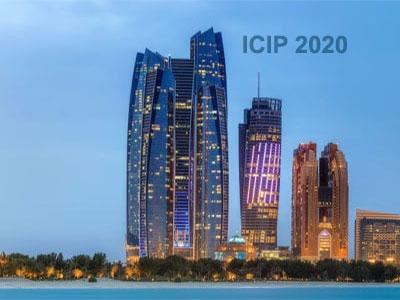
- Read more about Collaborative Learning of Semi-Supervised Clustering and Classification for Labeling Uncurated Data
- 1 comment
- Log in to post comments
Domain-specific image collections present potential value in various areas of science and business but are often not curated nor have any way to readily extract relevant content. To employ contemporary supervised image analysis methods on such image data, they must first be cleaned and organized, and then manually labeled for the nomenclature employed in the specific domain, which is a time consuming and expensive endeavor.
To address this issue, we designed and implemented the Plud system.
- Categories:
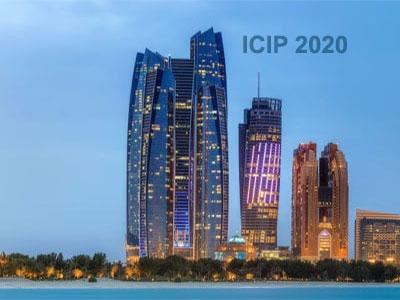
- Read more about Always Look on the Bright Side of the Field: Merging Pose and Contextual Data to Estimate Orientation of Soccer Players [Slides]
- Log in to post comments
SlidesICIP.pdf

- Categories:
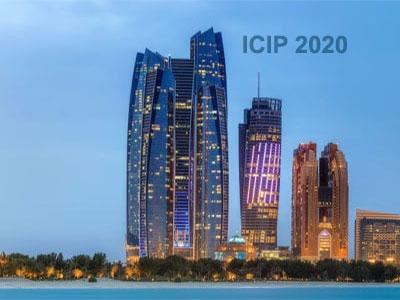
- Read more about 3D Point Cloud Enhancement using Graph-Modelled Multiview Depth Measurements
- Log in to post comments
A 3D point cloud is often synthesized from depth measurements collected by sensors at different viewpoints. The acquired measurements are typically both coarse in precision and corrupted by noise. To improve quality, previous works denoise a synthesized 3D point cloud a posteriori, after projecting the imperfect depth data onto the 3D space. Instead, we enhance depth measurements on the sensed images a priori, exploiting inherent 3D geometric correlation across views, before synthesizing a 3D point cloud from the improved measurements.
- Categories:
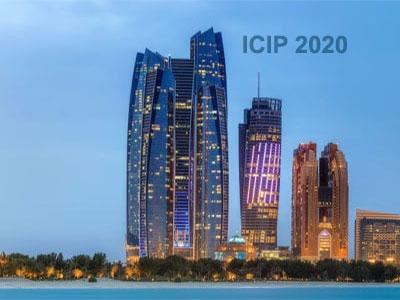
- Read more about Semantic Preserving Image Compression
- Log in to post comments
Video traffic comprises a large majority of the total traffic on the internet today. Uncompressed visual data requires a very large data rate; lossy compression techniques are employed in order to keep the data-rate manageable. Increasingly, a significant amount of visual data being generated is consumed by analytics (such as classification, detection, etc.) residing in the cloud. Image and video compression can produce visual artifacts, especially at lower data-rates, which can result in a significant drop in performance on such analytic tasks.
- Categories:
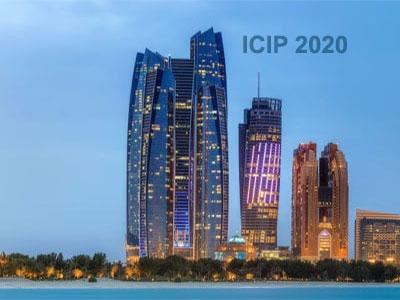
The multi-modality sensor fusion technique is an active research
area in scene understating. In this work, we explore
the RGB image and semantic-map fusion methods for depth
estimation. The LiDARs, Kinect, and TOF depth sensors are
unable to predict the depth-map at illuminate and monotonous
pattern surface. In this paper, we propose a semantic-to-depth
generative adversarial network (S2D-GAN) for depth estimation
from RGB image and its semantic-map. In the first stage,
the proposed S2D-GAN estimates the coarse level depthmap
- Categories:
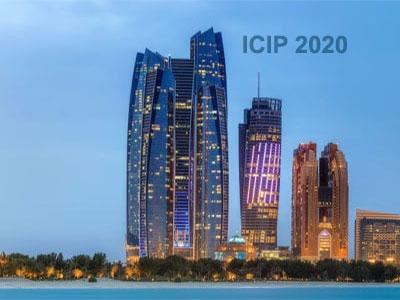
- Read more about Summarizing the performances of a background subtraction algorithm measured on several videos
- Log in to post comments
There exist many background subtraction algorithms to detect motion in videos. To help comparing them, datasets with ground-truth data such as CDNET or LASIESTA have been proposed. These datasets organize videos in categories that represent typical challenges for background subtraction. The evaluation procedure promoted by their authors consists in measuring performance indicators for each video separately and to average them hierarchically, within a category first, then between categories, a procedure which we name “summarization”.
- Categories:
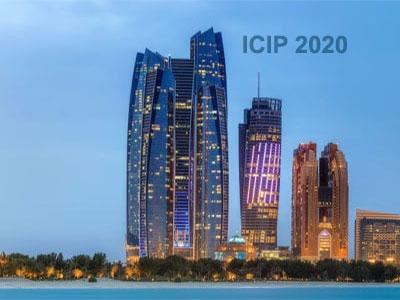
As a fundamental step of document related tasks, document classification has been widely adopted to various document image processing applications. Unlike the general image classification problem in the computer vision field, text document images contain both the visual cues and the corresponding text within the image. However, how to bridge these two different modalities and leverage textual and visual features to classify text document images remains challenging.
- Categories: