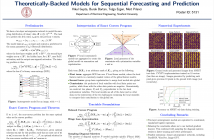
- Read more about Convex Neural Autoregressive Models: Towards Tractable, Expressive, and Theoretically-Backed Models for Sequential Forecasting and Prediction
- Log in to post comments
Three features are crucial for sequential forecasting and generation models: tractability, expressiveness, and theoretical backing. While neural autoregressive models are relatively tractable and offer powerful predictive and generative capabilities, they often have complex optimization landscapes, and their theoretical properties are not well understood. To address these issues, we present convex formulations of autoregressive models with one hidden layer.
- Categories:
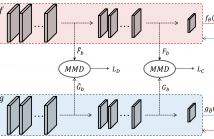
- Read more about A Consensual Collaborative Learning Method for Remote Sensing Image Classification under Noisy Multi-Labels
- Log in to post comments
Collecting a large number of reliable training images annotated by multiple land-cover class labels in the framework of multi-label classification is time-consuming and costly in remote sensing (RS). To address this problem, publicly available thematic products are often used for annotating RS images with zero-labeling-cost. However, such an approach may result in constructing a training set with noisy multi-labels, distorting the learning process. To address this problem, we propose a Consensual Collaborative Multi-Label Learning (CCML) method.
- Categories:
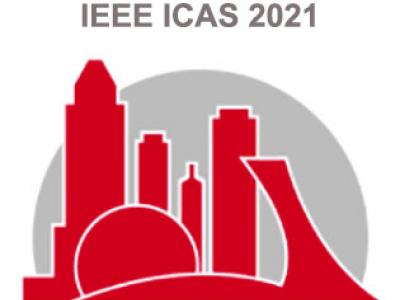
- Read more about An Off-Road Terrain Dataset Including Images Labeled With Measures of Terrain Roughness (Presentation Slides)
- Log in to post comments
- Categories:
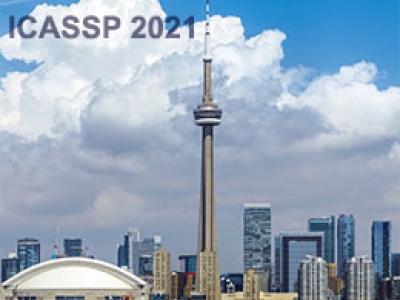
We summarise previous work showing that the basic sigmoid activation function arises as an instance of Bayes’s theorem, and that recurrence follows from the prior. We derive a layer- wise recurrence without the assumptions of previous work, and show that it leads to a standard recurrence with modest modifications to reflect use of log-probabilities. The resulting architecture closely resembles the Li-GRU which is the current state of the art for ASR. Although the contribution is mainly theoretical, we show that it is able to outperform the state of the art on the TIMIT and AMI datasets.
- Categories:
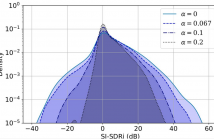
- Read more about (Poster) Unified Gradient Reweighting for Model Biasing with Applications to Source Separation
- Log in to post comments
Recent deep learning approaches have shown great improvement in audio source separation tasks. However, the vast majority of such work is focused on improving average separation performance, often neglecting to examine or control the distribution of the results. In this paper, we propose a simple, unified gradient reweighting scheme, with a lightweight modification to bias the learning process of a model and steer it towards a certain distribution of results. More specifically, we reweight the gradient updates of each batch, using a user-specified probability distribution.
- Categories:
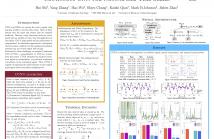
- Read more about Continuous CNN for Nonuniform Time Series
- Log in to post comments
CNN for time series data implicitly assumes that the data are uniformly sampled, whereas many event-based and multi-modal data are nonuniform or have heterogeneous sampling rates. Directly applying regular CNN to nonuniform time series is ungrounded, because it is unable to recognize and extract common patterns from the nonuniform input signals. In this paper, we propose the Continuous CNN (\myname), which estimates the inherent continuous inputs by interpolation, and performs continuous convolution on the continuous input.
- Categories:
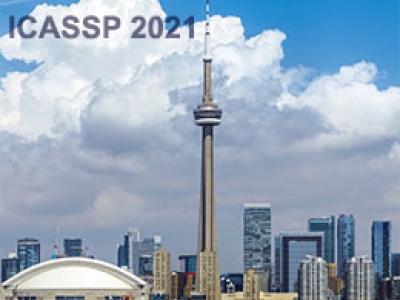
- Read more about ADA-SISE: ADAPTIVE SEMANTIC INPUT SAMPLING FOR EFFICIENT EXPLANATION OF CONVOLUTIONAL NEURAL NETWORKS
- Log in to post comments
Explainable AI (XAI) is an active research area to interpret a neural network’s decision by ensuring transparency and trust in the task-specified learned models.Recently,perturbation-based model analysis has shown better interpretation, but back-propagation techniques are still prevailing because of their computational efficiency. In this work, we combine both approaches as a hybrid visual explanation algorithm and propose an efficient interpretation method for convolutional neural networks.
- Categories:
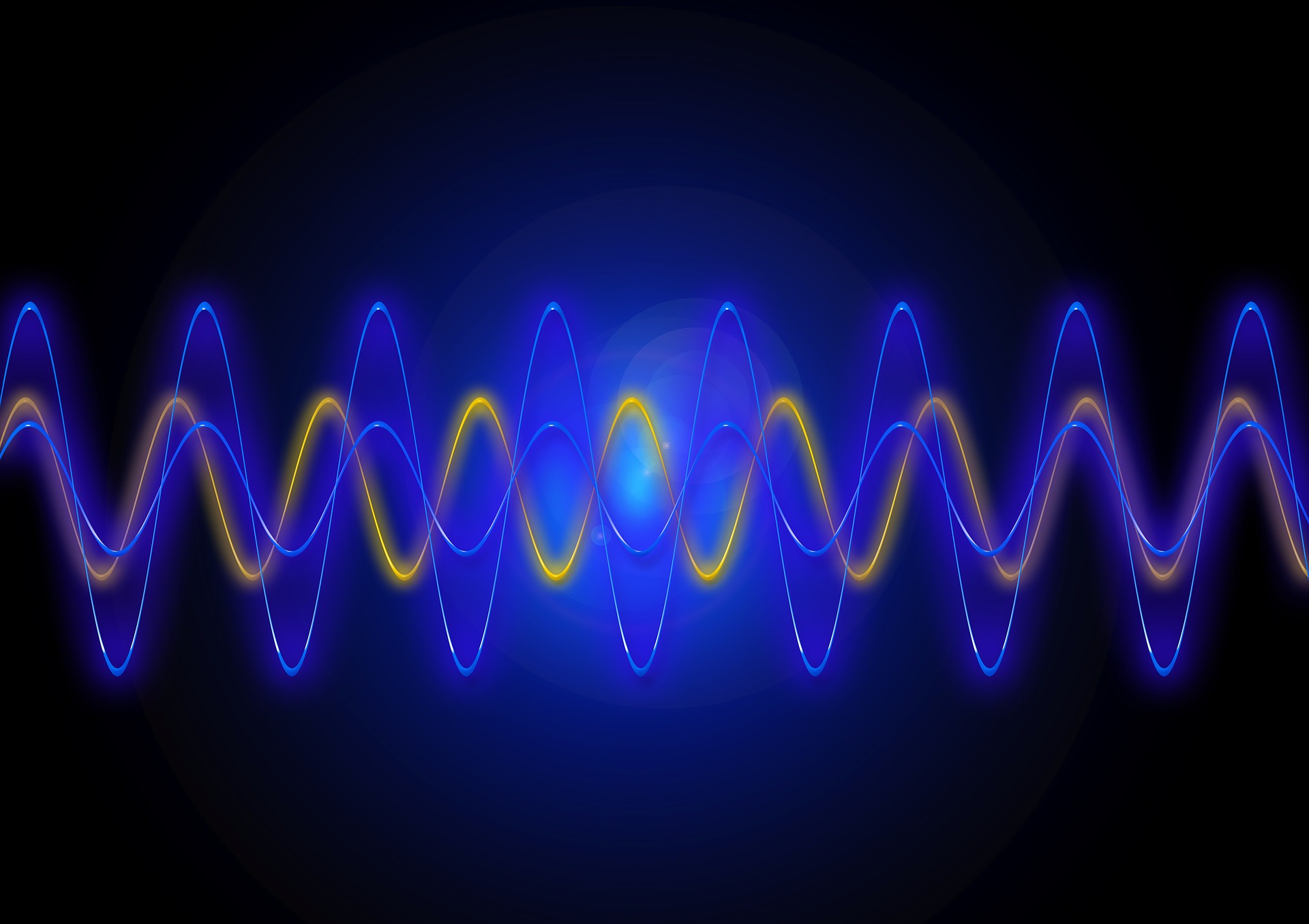
- Read more about Fusion Neural Network for Vehicle Trajectory Prediction in Autonomous Driving
- Log in to post comments
- Categories:
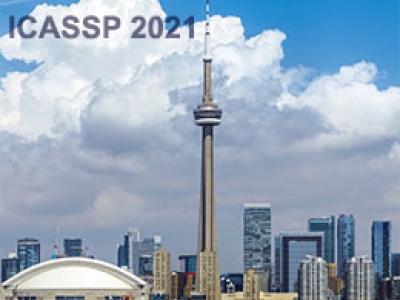
- Read more about CHANNEL-WISE MIX-FUSION DEEP NEURAL NETWORKS FOR ZERO-SHOT LEARNING
- Log in to post comments
- Categories:
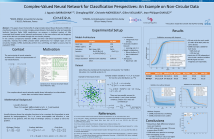
- Read more about Complex-Valued Vs. Real-Valued Neural Networks for Classification Perspectives: An Example on Non-Circular Data
- Log in to post comments
This paper shows the benefits of using Complex-Valued Neural Network (CVNN) on classification tasks for non-circular complex-valued datasets. Motivated by radar and especially Synthetic Aperture Radar (SAR) applications, we propose a statistical analysis of fully connected feed-forward neural networks performance in the cases where real and imaginary parts of the data are correlated through the non-circular property.
- Categories: