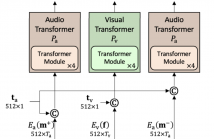
- Read more about ModEFormer: Modality-preserving embedding for audio-video synchronization using transformers
- Log in to post comments
Lack of audio-video synchronization is a common problem during television broadcasts and video conferencing, leading to an unsatisfactory viewing experience. A widely accepted paradigm is to create an error detection mechanism that identifies the cases when audio is leading or lagging. We propose ModEFormer, which independently extracts audio and video embeddings using modality-specific transformers.
- Categories:
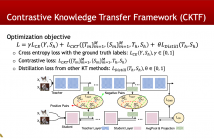
- Read more about A Contrastive Knowledge Transfer Framework for Model Compression and Transfer Learning
- 1 comment
- Log in to post comments
Knowledge Transfer (KT) achieves competitive performance and is widely used for image classification tasks in model compression and transfer learning. Existing KT works transfer the information from a large model ("teacher") to train a small model ("student") by minimizing the difference of their conditionally independent output distributions.
- Categories:
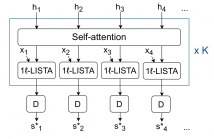
- Read more about Designing Transformer networks for sparse recovery of sequential data using deep unfolding
- Log in to post comments
Deep unfolding models are designed by unrolling an optimization algorithm into a deep learning network. These models have shown faster convergence and higher performance compared to the original optimization algorithms. Additionally, by incorporating domain knowledge from the optimization algorithm, they need much less training data to learn efficient representations. Current deep unfolding networks for sequential sparse recovery consist of recurrent neural networks (RNNs), which leverage the similarity between consecutive signals.
- Categories:
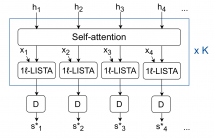
- Read more about Designing Transformer networks for sparse recovery of sequential data using deep unfolding: Presentation
- Log in to post comments
Deep unfolding models are designed by unrolling an optimization algorithm into a deep learning network. These models have shown faster convergence and higher performance compared to the original optimization algorithms. Additionally, by incorporating domain knowledge from the optimization algorithm, they need much less training data to learn efficient representations. Current deep unfolding networks for sequential sparse recovery consist of recurrent neural networks (RNNs), which leverage the similarity between consecutive signals.
- Categories:
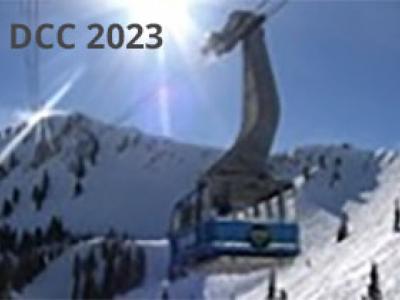
- Read more about Applicability limitations of differentiable full-reference image-quality metrics
- Log in to post comments
Subjective image-quality measurement plays a critical role in the development of image- processing applications. The purpose of a visual-quality metric is to approximate the results of subjective assessment. In this regard, more and more metrics are under development, but little research has considered their limitations. This paper addresses that deficiency: we show how image preprocessing before compression can artificially increase the quality scores provided by the popular metrics DISTS, LPIPS, HaarPSI, and VIF as well as how these scores are inconsistent with subjective-quality scores.
DCC_pptx.pptx

- Categories:
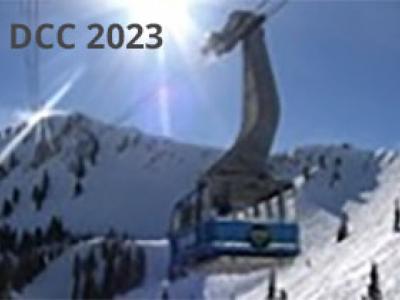
Deep variational autoencoders for image and video compression have gained significant attraction
in the recent years, due to their potential to offer competitive or better compression
rates compared to the decades long traditional codecs such as AVC, HEVC or VVC. However,
because of complexity and energy consumption, these approaches are still far away
from practical usage in industry. More recently, implicit neural representation (INR) based
codecs have emerged, and have lower complexity and energy usage to classical approaches at
- Categories:
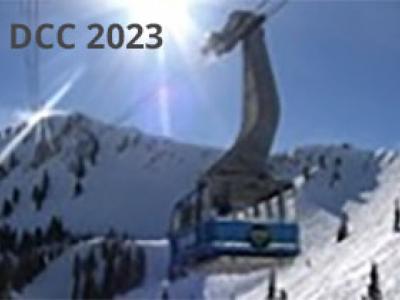
- Read more about Learned Disentangled Latent Representations for Scalable Image Coding for Humans and Machines
- Log in to post comments
As an increasing amount of image and video content will be analyzed by machines, there is demand for a new codec paradigm that is capable of compressing visual input primarily for the purpose of computer vision inference, while secondarily supporting input reconstruction. In this work, we propose a learned compression architecture that can be used to build such a codec. We introduce a novel variational formulation that explicitly takes feature data relevant to the desired inference task as input at the encoder side.
- Categories:
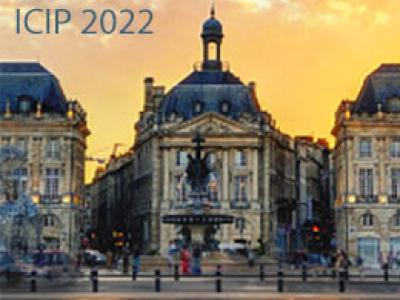
- Read more about Automatic Defect Segmentation by Unsupervised Anomaly Learning
- Log in to post comments
This paper addresses the problem of defect segmentation in semiconductor manufacturing. The input of our segmentation is a scanning-electron-microscopy (SEM) image of the candidate defect region. We train a U-net shape network to segment defects using a dataset of clean background images. The samples of the training phase are produced automatically such that no manual labeling is required. To enrich the dataset of clean background samples, we apply defect implant augmentation. To that end, we apply a copy-and-paste of a random image patch in the clean specimen.
- Categories:
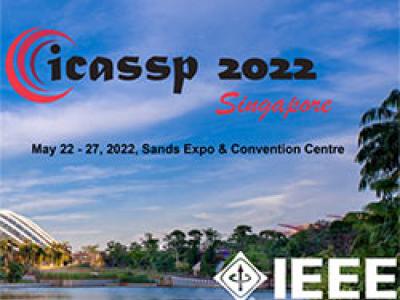
- Read more about TRIBYOL: TRIPLET BYOL FOR SELF-SUPERVISED REPRESENTATION LEARNING
- Log in to post comments
1917-1.pdf

- Categories:
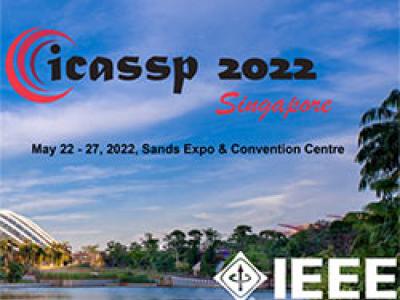
- Read more about 4D Convolutional Neural Networks for Multi Spectral and Multi Temporal Remote Sensing Data Classification
- Log in to post comments
Multi-temporal remotely sensed observations acquired by multi-spectral sensors contain a wealth of information related to the Earth’s state. Deep learning methods have demonstrated a great potential in analyzing such observations. Traditional 2D and 3D approaches are unable to effectively extract valuable information encoded across all available dimensions.
- Categories: