- Signal and System Modeling, Representation and Estimation
- Multirate Signal Processing
- Sampling and Reconstruction
- Nonlinear Systems and Signal Processing
- Filter Design
- Adaptive Signal Processing
- Statistical Signal Processing
- Read more about TIME SAMPLES SELECTION IN SPIRAL ACQUISITION FOR SPARSE MAGNETIC RESONANCE SPECTROSCOPIC IMAGING
- Log in to post comments
- Categories:
- Read more about Phase Retrieval via Coordinate Descent
- Log in to post comments
Phase retrieval refers to recovery of a signal-of-interest given only the intensity measurement samples and has wide applicability including important areas of astronomy, computational biology, crystallography, digital communications, electron microscopy, neutron radiography and optical imaging. The classical problem formulation is to restore the time-domain signal from its power spectrum observations, although the Fourier transform can be generalized to any linear mappings.
- Categories:
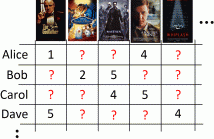
Matrix completion aims to find the missing entries from incomplete observations using the low-rank property. Conventional convex optimization based techniques minimize the nuclear norm subject to a constraint on the Frobenius norm of the residual. However, they are not robust to outliers and have a high computational complexity. Different from the existing schemes based on solving a minimization problem, we formulate matrix completion as a feasibility problem.
- Categories:
- Read more about PARTICLE PHD FILTER BASED MULTI-TARGET TRACKING USING DISCRIMINATIVE GROUP-STRUCTURED DICTIONARY LEARNING
- Log in to post comments
Structured sparse representation has been recently found to achieve better efficiency and robustness in exploiting the target appearance model in tracking systems with both holistic and local information. Therefore, to better simultaneously discriminate multi-targets from their background, we propose a novel video-based multi-target tracking system that combines the particle probability hypothesis density (PHD) filter with discriminative group-structured dictionary learning.
- Categories:
- Read more about An M-Channel Critically Sampled Graph Filter Bank
- Log in to post comments
We investigate an M-channel critically sampled filter bank for graph signals where each of the M filters is supported on a different subband of the graph Laplacian spectrum. We partition the graph vertices such that the mth set comprises a uniqueness set for signals supported on the mth subband. For analysis, the graph signal is filtered on each subband and downsampled on the corresponding set of vertices.
- Categories:
- Read more about Building Recurrent Networks by Unfolding Iterative Thresholding for Sequential Sparse Recovery
- Log in to post comments
Historically, sparse methods and neural networks, particularly modern deep learning methods, have been relatively disparate areas. Sparse methods are typically used for signal enhancement, compression,and recovery, usually in an unsupervised framework, while neural networks commonly rely on a supervised training set.
- Categories:
- Read more about NEW ASYMPTOTIC PROPERTIES FOR THE ROBUST ANMF
- Log in to post comments
- Categories:
- Read more about SUPERPIXEL-GUIDED CFAR DETECTION OF SHIPS AT SEA IN SAR IMAGERY
- Log in to post comments
- Categories:
- Read more about Online Empirical Mode Decomposition
- Log in to post comments
The success of Empirical Mode Decomposition (EMD) resides in its practical approach to dissect non-stationary data. EMD repetitively goes through the entire data span to iteratively extract Intrinsic Mode Functions (IMFs). This approach, however, is not suitable for data stream as the entire data set has to be reconsidered every time a new point is added. To overcome this, we propose Online EMD, an algorithm that extracts IMFs on the fly.
- Categories: