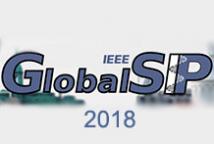
The 6th IEEE Global Conference on Signal and Information Processing (GlobalSIP) focuses on signal and information processing with an emphasis on up-and-coming signal processing themes. The conference features world-class plenary speeches, distinguished symposium talks, tutorials, exhibits, oral and poster sessions, and panels. GlobalSIP is comprised of co-located General Symposium and symposia selected based on responses to the call-for-symposia proposals.
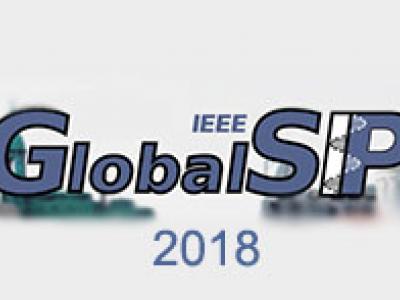
- Categories:
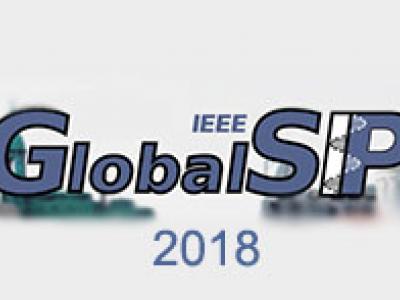
- Read more about RANDOMIZED METHOD FOR ESTIMATING THE VON NEUMANN ENTROPY OF LARGE-SCALE DENSITY MATRICES
- Log in to post comments
- Categories:
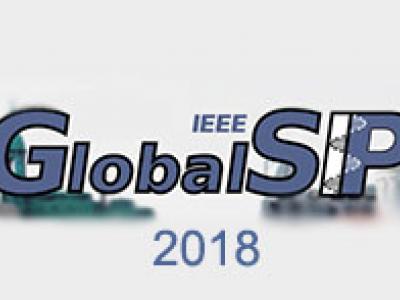
- Read more about SPARSE ERROR CORRECTION FOR PMU DATA UNDER GPS SPOOFING ATTACKS
- Log in to post comments
Time-synchronized phasor measurements from phasor measurement units (PMUs) are valuable for real time monitoring and control. However, their reliance on civilian GPS signals makes them vulnerable to GPS signal spoofing attacks which can be launched by an adversary to falsify PMU data entries.
- Categories:
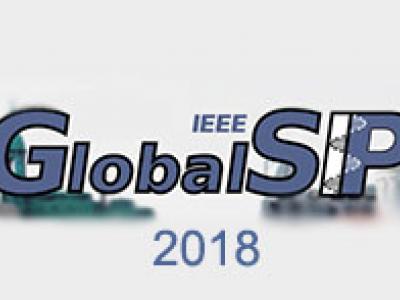
- Read more about RANDOM ENSEMBLE OF LOCALLY OPTIMUM DETECTORS FOR DETECTION OF ADVERSARIAL EXAMPLES
- Log in to post comments
- Categories:
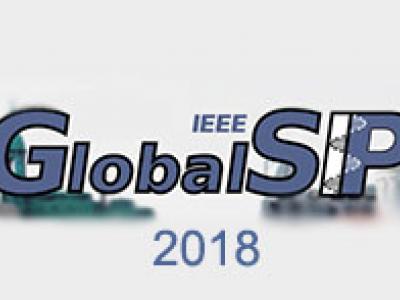
- Read more about Coincident Peak Prediction Using a Feed-Forward Neural Network
- Log in to post comments
A significant portion of a business' annual electrical payments can be made up of coincident peak charges: a transmission surcharge for power consumed when the entire system is at peak demand. This charge occurs only a few times annually, but with per-MW prices orders of magnitudes higher than non-peak times. A business is incentivized to reduce its power consumption, but accurately predicting the timing of peak demand charges is nontrivial. In this paper we present a decision framework based on predicting the day-ahead likelihood of peak demand charges.
- Categories:
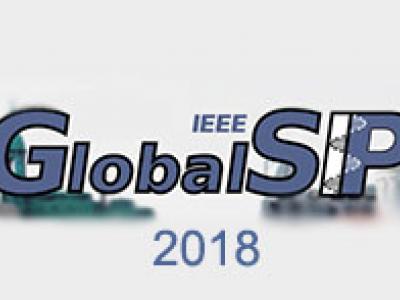
- Read more about A MACHINE LEARNING APPROACH FOR THE CLASSIFICATION OF INDOOR ENVIRONMENTS USING RF SIGNATURES
- Log in to post comments
Efficient deployment of Internet of Things (IoT) sensors primarily depends on allowing the adjustment of sensor power consumption according to the radio frequency (RF) propagation channel which is dictated by the type of the surrounding indoor environment. This paper develops a machine learning approach for indoor environment classification by exploiting support vector machine (SVM) based on RF signatures computed from real-time measurements.
- Categories:
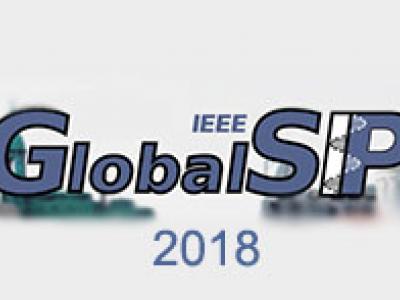
- Read more about BAYESIAN QUICKEST CHANGE POINT DETECTION WITH MULTIPLE CANDIDATES OF POST-CHANGE MODELS
- Log in to post comments
We study the quickest change point detection for systems with multiple possible post-change models. A change point is the time instant at which the distribution of a random process changes. We consider the case that the post-change model is from a finite set of possible models. Under the Bayesian setting, the objective is to minimize the average detection delay (ADD), subject to upper bounds on the probability of false alarm (PFA). The proposed algorithm is a threshold-based sequential test.
- Categories:
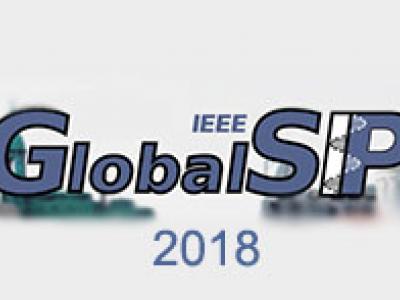
- Read more about BANDWIDTH AND ROUND-TRIP TIME DETECTION BASED CONGESTION CONTROL FOR MULTIPATH TCP OVER HIGHLY LOSSY SATELLITE NETWORKS
- Log in to post comments
Satellite systems might become an important supplement to 5G systems. In addition, compared with regular TCP, multipath TCP (MPTCP) provides a robust solution. However, due to the high latency and high loss of satellite networks, most of the existing multipath TCP congestion control schemes do not perform well. Therefore, we proposed a bandwidth and round-trip time detection-based congestion control (BWRD) for multipath TCP over highly lossy satellite networks. Simulations are running to evaluate the proposed BWRD algorithm.
- Categories:
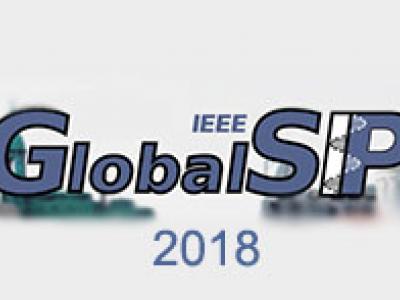
- Read more about Defending DNN Adversarial Attacks with Pruning and Logits Augmentation
- Log in to post comments
Deep neural networks (DNNs) have been shown to be powerful models and perform extremely well on many complicated artificial intelligent tasks. However, recent research found that these powerful models are vulnerable to adversarial attacks, i.e., intentionally added imperceptible perturbations to DNN inputs can easily mislead the DNNs with extremely high confidence. In this work, we enhance the robustness of DNNs under adversarial attacks by using pruning method and logits augmentation, we achieve both effective defense against adversarial examples and DNN model compression.
- Categories:
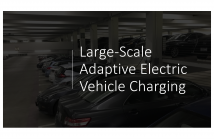
- Read more about LARGE-SCALE ADAPTIVE ELECTRIC VEHICLE CHARGING
- Log in to post comments
Large-scale charging infrastructure will play an important role in supporting the adoption of electric vehicles. In this presentation, we describe a unique physical testbed for large-scale, high-density EV charging research which we call the Adaptive Charging Network (ACN). We describe the architecture of the ACN including its hardware and software components. We also present a practical framework for online scheduling, which is based on model predictive control and convex optimization.
- Categories: