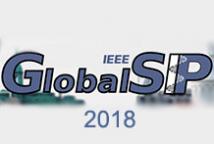
The 6th IEEE Global Conference on Signal and Information Processing (GlobalSIP) focuses on signal and information processing with an emphasis on up-and-coming signal processing themes. The conference features world-class plenary speeches, distinguished symposium talks, tutorials, exhibits, oral and poster sessions, and panels. GlobalSIP is comprised of co-located General Symposium and symposia selected based on responses to the call-for-symposia proposals.
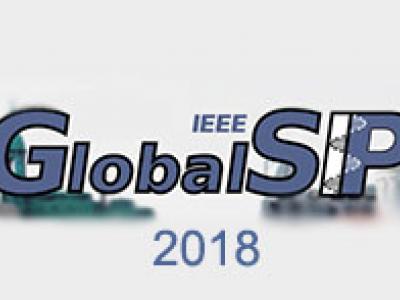
- Read more about A Novel Approach to Joint User Selection and Precoding for Multiuser MISO Downlink Channels
- Log in to post comments
The downlink capacity of a unicast network with a large number of users than the base station transmit antennas depends on user selection and interference among the selected users. Various suboptimal selection schemes in combination with suboptimal or optimal precoding have been proposed in the literature, and some of these techniques asymptotically achieve the sum capacity of DPC, as the number of users goes to infinity. In the previous works, the joint design problem is addressed as a decoupled problem of selection and precoding either at the design level or the solution level.
- Categories:
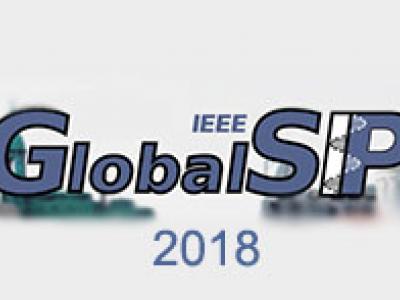
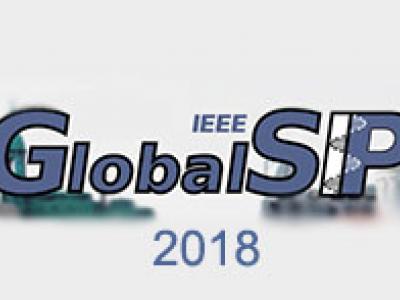
- Read more about Contact Surface Area: A Novel Signal for Heart Rate Estimation in Smartphone Videos
- Log in to post comments
Smartphone video-based measurement of heart rate typically uses photoplethysmography (PPG). Prior accuracy studies report low mean absolute errors for apps based on contact PPG on a fingertip, but substantial errors on a troubling percentage of measurements. In this study, we aimed to reduce the rate of substantial heart rate estimation errors by introducing a novel signal present in fingertip videos: fingertip contact surface area.
- Categories:
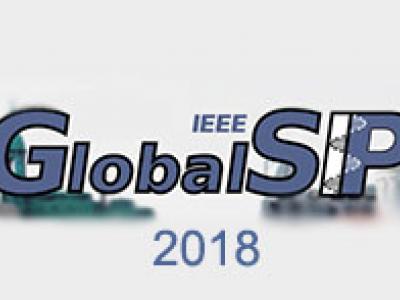
- Read more about PHYSICAL LAYER ABSTRACTION FOR PERFORMANCE EVALUATION OF LEO SATELLITE SYSTEM FOR IOT USING TIME-FREQUENCY ALOHA SCHEME
- Log in to post comments
One of the main issues in using a Low Earth Orbit (LEO) satellite constellation to extend a Low-Powered Wide Area Network is the frequency synchronization. Using a link based on random access solves this concern, but also prevents delivery guarantees,
and implies less predictable performance. This paper concerns the estimation of Bit Error Rate (BER) and Packet Error Rate (PER) using physical layer abstractions under a time and frequency random scheme, namely Time and Frequency Aloha. We first derive a BER
- Categories:
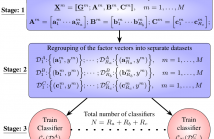
- Read more about Tensor Ensemble Learning
- Log in to post comments
In big data applications, classical ensemble learning is typically infeasible on the raw input data and dimensionality reduction techniques are necessary. To this end, novel framework that generalises classic flat-view ensemble learning to multidimensional tensor- valued data is introduced. This is achieved by virtue of tensor decompositions, whereby the proposed method, referred to as tensor ensemble learning (TEL), decomposes every input data sample into multiple factors which allows for a flexibility in the choice of multiple learning algorithms in order to improve test performance.
- Categories:
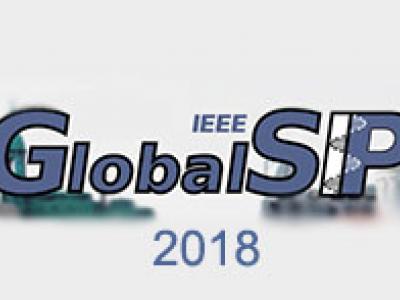
- Read more about Is Ordered Weighed L1 Regularized Regression Robust to Adversarial Perturbation ? A Case Study on OSCAR
- Log in to post comments
Many state-of-the-art machine learning models such as deep neural networks have recently shown to be vulnerable to adversarial perturbations, especially in classification tasks. Motivated by adversarial machine learning, in this paper we investigate the robustness of sparse regression models with strongly correlated covariates to adversarially designed measurement noises. Specifically, we consider the family of ordered weighted L1 (OWL) regularized regression methods and study the case of OSCAR (octagonal shrinkage clustering algorithm for regression) in the adversarial setting.
- Categories:
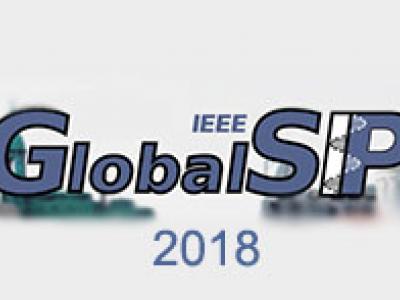
- Read more about Optimal Local Thresholds for Distributed Detection in Energy Harvesting Wireless Sensor Networks
- Log in to post comments
- Categories:
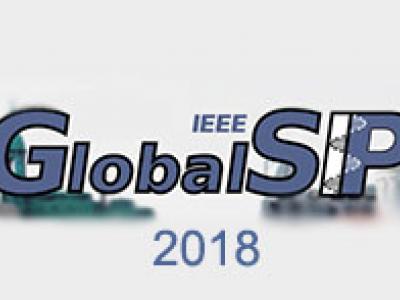
- Read more about ON THE BEHAVIOR OF THE EXPECTATION-MAXIMIZATION ALGORITHM FOR MIXTURE MODELS
- Log in to post comments
Globalsip.pdf

- Categories:
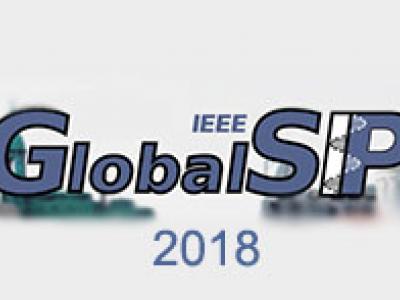
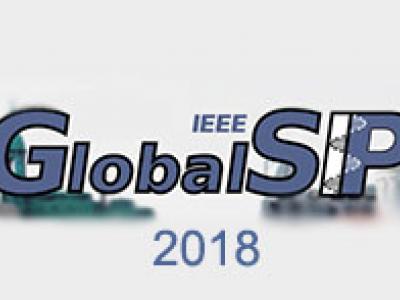
- Read more about Presentation slides for GlobalSIP 2018
- Log in to post comments
In this paper, we investigate the impact of multiple-antenna
deployment at access points (APs) and users on the performance
of cell-free massive multiple-input multiple-output
(MIMO). The transmission is done via time-division duplex
(TDD) protocol. With this protocol, the channels are first
estimated at each AP based on the received pilot signals
in the training phase. Then these channel information will
be used to decode the symbols before sending to all users.
The simple and distributed conjugate beamforming technique
- Categories: