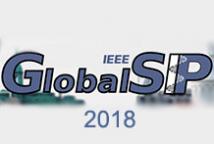
The 6th IEEE Global Conference on Signal and Information Processing (GlobalSIP) focuses on signal and information processing with an emphasis on up-and-coming signal processing themes. The conference features world-class plenary speeches, distinguished symposium talks, tutorials, exhibits, oral and poster sessions, and panels. GlobalSIP is comprised of co-located General Symposium and symposia selected based on responses to the call-for-symposia proposals.
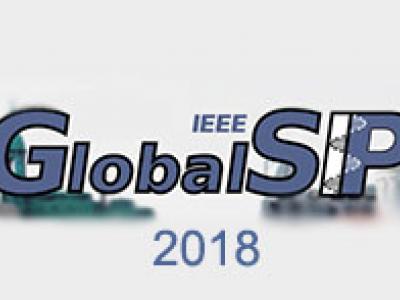
- Read more about NEURAL LATTICE DECODERS
- Log in to post comments
Lattice decoders constructed with neural networks are presented.
Firstly, we show how the fundamental parallelotope
is used as a compact set for the approximation by a neural lattice
decoder. Secondly, we introduce the notion of Voronoi reduced
lattice basis. As a consequence, a first optimal neural
lattice decoder is built from Boolean equations and the facets
of the Voronoi cell. This decoder needs no learning. Finally,
we present two neural decoders with learning. It is shown
- Categories:
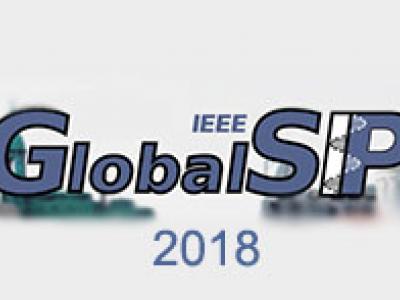
- Read more about A Novel Approach to Joint User Selection and Precoding for Multiuser MISO Downlink Channels
- Log in to post comments
The downlink capacity of a unicast network with a large number of users than the base station transmit antennas depends on user selection and interference among the selected users. Various suboptimal selection schemes in combination with suboptimal or optimal precoding have been proposed in the literature, and some of these techniques asymptotically achieve the sum capacity of DPC, as the number of users goes to infinity. In the previous works, the joint design problem is addressed as a decoupled problem of selection and precoding either at the design level or the solution level.
- Categories:
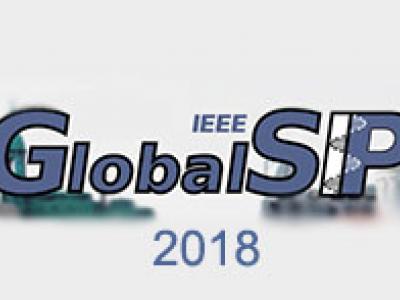
- Read more about A Performance Analysis on the Optimal Number of Measurements for Coded Compressive Imaging
- Log in to post comments
In this paper, we consider two practical coded compressive imaging techniques. We investigate the optimal number of measurements under quadratic signal-to-noise-ratio (SNR) decrease. We focus on imaging scenarios in both real and complex vector spaces. In real vector spaces, we consider focal plane array (FPA) based super-resolution imaging with a constant measurement time constraint. Our model is comprised of a spatial light modulator and a low resolution FPA for modulating and sampling the incoming light intensity, respectively.
- Categories:
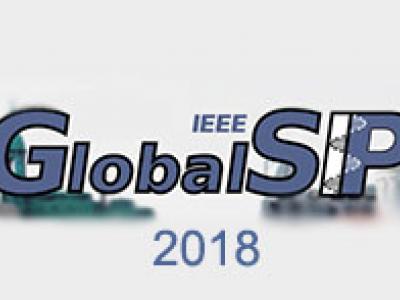
- Read more about SOURCE SEPARATION IN THE PRESENCE OF SIDE INFORMATION: NECESSARY AND SUFFICIENT CONDITIONS FOR RELIABLE DE-MIXING
- Log in to post comments
- Categories:
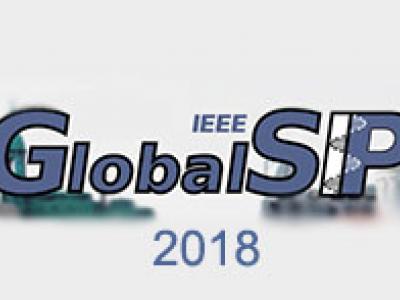
- Read more about Delayed Weight Update for Faster Convergence in Data-parallel Deep Learning
- Log in to post comments
- Categories:
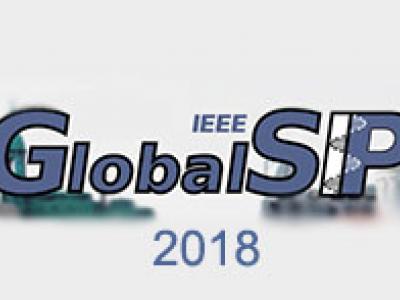
- Read more about DEPLOYING JOINT BEAM HOPPING AND PRECODING IN MULTIBEAM SATELLITE NETWORKS WITH TIME VARIANT TRAFFIC
- Log in to post comments
This paper studies the application of Beam Hopping (BH) as a key enabler to provide high level of flexibility to manage scarce on-board resources, particularly power, based on the irregular and time variant traffic requests/demands distributed within the coverage of a satellite multibeam system. However, while high throughput full frequency reuse pattern is employed among beams, the performance of BH is significantly degraded due to the generated inter-beam interference, and applying precoding is essential. In this context, we propose Joint Precoding and BH (J-PBH) in a multibeam system.
- Categories:
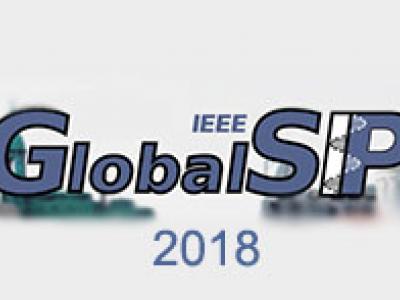
- Read more about Using Linear Prediction to Mitigate End Effects in Empirical Mode Decomposition
- Log in to post comments
It is well known that empirical mode decomposition can suffer from computational instabilities at the signal boundaries. These ``end effects'' cause two problems: 1) sifting termination issues, i.e.~convergence and 2) estimation error, i.e.~accuracy. In this paper, we propose to use linear prediction in conjunction with a previous method to address end effects, to further mitigate these problems.
- Categories:
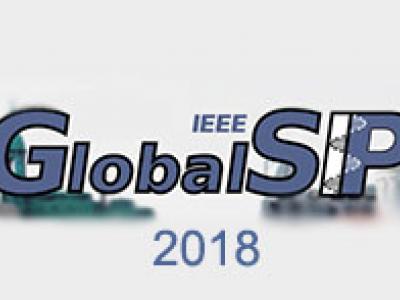
- Read more about Dominant Component Tracking for Empirical Mode Decomposition using a Hidden Markov Model
- Log in to post comments
It is well known that the empirical mode decomposition algorithm does not always return an appropriate decomposition due to problems like mode mixing. In this paper, we consider the problem of a component being split across several intrinsic mode functions (IMFs). We propose the use of a hidden Markov model (HMM) to track the dominant component across the set of IMFs returned by EMD.
- Categories:
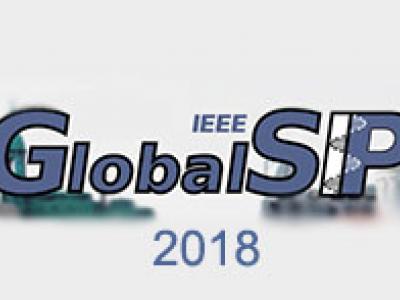
- Read more about Single image super-resolution with limited number of filters
- Log in to post comments
In this paper, we propose a single image super-resolution with limited number of filters based on RAISR. RAISR is well known as rapid and accurate super-resolution method which utilizes 864 filters for upscaling. This super-resolution idea utilizes the filter learned with sufficient training set. To get low cost of calculation and comparable image quality with other highly accurate super-resolution methods, the patch of input image is classified into classes by simple hash calculation.
- Categories:
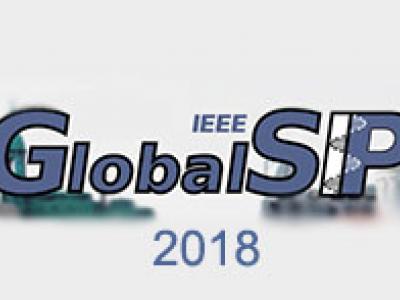
- Categories: