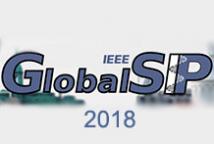
The 6th IEEE Global Conference on Signal and Information Processing (GlobalSIP) focuses on signal and information processing with an emphasis on up-and-coming signal processing themes. The conference features world-class plenary speeches, distinguished symposium talks, tutorials, exhibits, oral and poster sessions, and panels. GlobalSIP is comprised of co-located General Symposium and symposia selected based on responses to the call-for-symposia proposals.
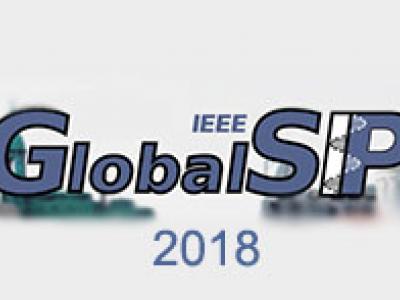
- Read more about PERFORMANCE EVALUATION OF OBJECTIVE QUALITY METRICS ON HLG-BASED HDR IMAGE CODING
- Log in to post comments
We evaluate the performance of objective quality metrics for high dynamic range (HDR) image coding that uses the transfer function (TF) of the Hybrid Log-Gamma (HLG) method. Previous evaluations of objective metrics for HDR image coding have studied which of them are reliable predictors of perceived quality; however, in those tests, all the non-linear transforms used both for encoding and by the best-performing metrics are essentially very similar and based on visual perception data of detection thresholds for lightness variations.
- Categories:
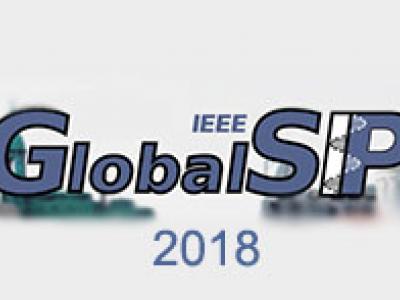
- Read more about USING DEEP CROSS MODAL HASHING AND ERROR CORRECTING CODES FOR IMPROVING THE EFFICIENCY OF ATTRIBUTE GUIDED FACIAL IMAGE RETRIEVAL
- Log in to post comments
With benefits of fast query speed and low storage cost,hashing-based image retrieval approaches have garnered considerable attention from the research community. In this pa-per, we propose a novel Error-Corrected Deep Cross Modal Hashing (CMH-ECC) method which uses a bitmap specifying the presence of certain facial attributes as an input query to retrieve relevant face images from the database.
- Categories:
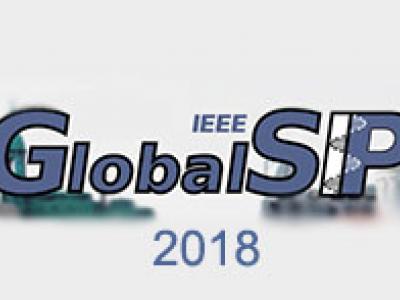
- Read more about A New Look at Secrecy Capacity of MIMOME Using Artificial Noise from Alice and Bob without Knowledge of Eve’s CSI
- Log in to post comments
This study investigates a secure wireless communication scheme which combines two of the most effective strategies to combat (passive) eavesdropping, namely mixing information with artificial noise at the transmitter and jamming from a full-duplex receiver. All nodes are assumed to possess multiple antennas, which is known as a MIMOME network. The channel state information (CSI) of Eve is known to Eve but not to Alice and Bob. While such setup has been investigated in related works, new and important insights are revealed in this work.
- Categories:
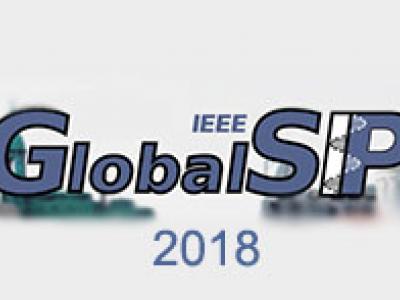
- Read more about 60-GHZ MILLIMETER-WAVE PATHLOSS MEASUREMENTS IN BOISE AIRPORT
- Log in to post comments
This paper presents a large-scale fading channel model at the 60 GHz band. This model is based on the measurement campaign that our team conducted at Boise Airport and Boise State University. The close-in reference path loss and floating-intercept path loss models with both statistical and stochastic approaches are investigated for these environments. The measurements were collected at several different locations in line-of-sight (LOS) and non-line-of-sight (NLOS) scenarios using a high gain directional antenna.
- Categories:
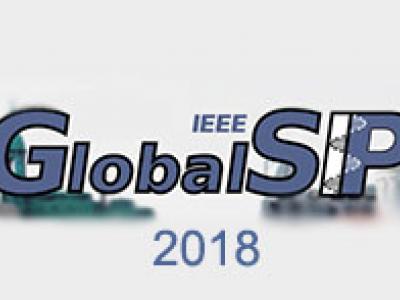
- Read more about POLARITY INVARIANT TRANSFORMATION FOR EEG MICROSTATES ANALYSIS
- Log in to post comments
Electroencephalography (EEG) has been widely used in human brain research. Several techniques in EEG relies on analyzing the topographical distribution of the data. One of the most common analysis is EEG microstate (EEG-ms). EEG-ms reflects the stable topographical representation of EEG signal lasting a few dozen milliseconds. EEG-ms were associated with resting state fMRI networks and were associated with mental processes and abnormalities.
- Categories:
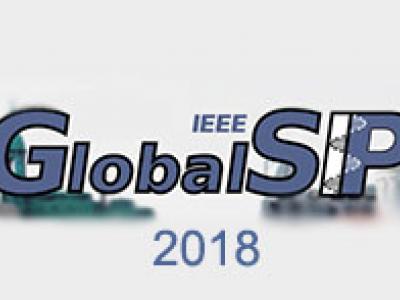
- Read more about Gradient-Based Solution of Maximum Likelihood Angle Estimation for Virtual Array Measurements
- Log in to post comments
Precise measurement and characterization of millimeter wave channels requires antennas capable of high angular resolution to resolve closely spaced multipath sources. To achieve angular resolution on the order of a few degrees these antennas must be electrically large which is impractical for phased array architectures at millimeter wave frequencies. An alternative approach is to synthesize a virtual aperture in space by using an accurate mechanical positioner to move a receive antenna to points along a sampling grid.
- Categories:
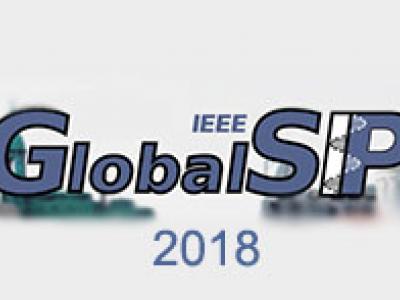
- Read more about On Optimal Sensing and Capacity Trade-off in Cognitive Radio Systems with Directional Antennas
- Log in to post comments
We consider a cognitive radio system, in which the secondary users (SUs) and primary users (PUs) coexist. The SUs are equipped with steerable directional antennas. In our system, the secondary transmitter (SUtx) first senses the spectrum (with errors) for a duration of τ, and, then transmits data to the secondary receiver (SUrx) if spectrum is sensed idle. The sensing time as well as the orientation of SUtx's antenna affect the accuracy of spectrum sensing and yield a trade-off between spectrum sensing and capacity of the secondary network.
- Categories:
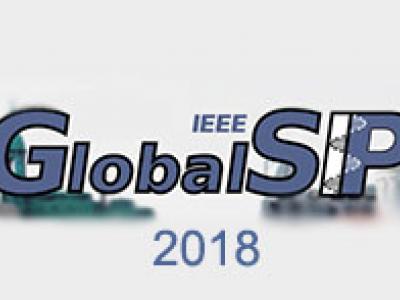
- Read more about Reinforced Adversarial Attacks on Deep Neural Networks Using ADMM
- Log in to post comments
- Categories:
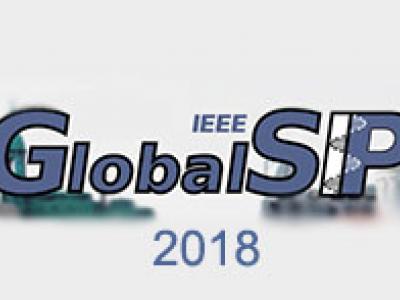
Multi-view frame reconstruction is an important problem particularly when multiple frames are missing and past and future frames within the camera are far apart from the missing ones. Realistic coherent frames can still be reconstructed using corresponding frames from other overlapping cameras. We propose an adversarial approach to learn the
spatio-temporal representation of the missing frame using conditional Generative Adversarial Network (cGAN). The conditional input to each cGAN is the preceding or following
GlobalSIP.pdf

- Categories: