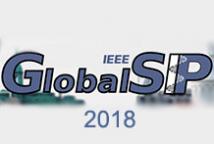
The 6th IEEE Global Conference on Signal and Information Processing (GlobalSIP) focuses on signal and information processing with an emphasis on up-and-coming signal processing themes. The conference features world-class plenary speeches, distinguished symposium talks, tutorials, exhibits, oral and poster sessions, and panels. GlobalSIP is comprised of co-located General Symposium and symposia selected based on responses to the call-for-symposia proposals.
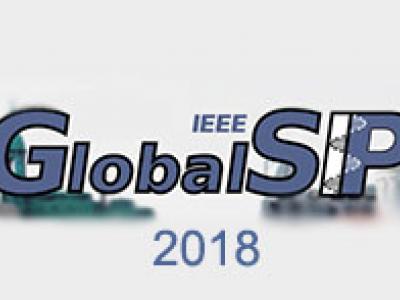
- Read more about On The Utility of Conditional Generation Based Mutual Information For Characterizing Adversarial Subspaces
- Log in to post comments
- Categories:
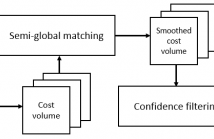
- Read more about Disparity Map Estimation from Cross-modal Stereo
- Log in to post comments
Mono-modal stereo matching problem has been studied for decades. The introduction of cross-modal stereo systems in industrial scene increases the interest in cross-modal stereo matching. The existing algorithms mostly consider mono-modal setting so they do not translate well in cross-modal setting. Recent development for cross-modal stereo considers small local matching and focus mainly on joint enhancement. Therefore, we propose a guided filter-based stereo matching algorithm. It works by integrating guided filter equation in a basic cost function for cost volume generation.
- Categories:
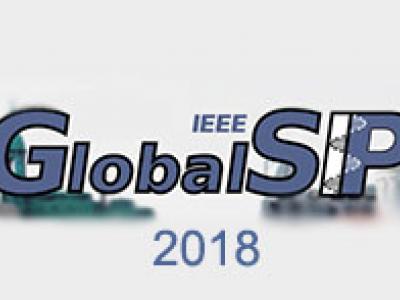
- Read more about Non-Asymptotic Rates for Communication Efficient Distributed Zeroth Order Strongly Convex Optimization
- Log in to post comments
This paper focuses on the problem of communication efficient distributed zeroth order minimization of a sum of strongly convex loss functions. Specifically, we develop distributed stochastic optimization methods for zeroth order strongly convex optimization that are based on an adaptive probabilistic sparsifying communications protocol.
- Categories:
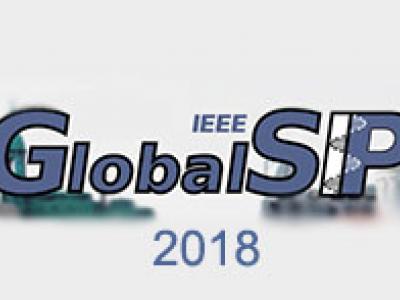
- Read more about Persistent Hyperspectral Observations of the Urban Lightscape
- Log in to post comments
- Categories:
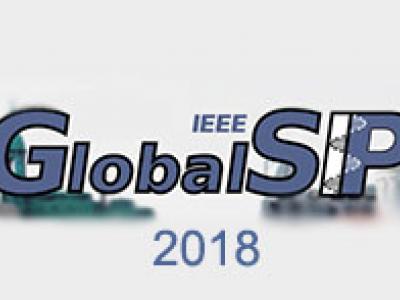
- Read more about Wideband Massive MIMO Channel Estimation via Sequential Atomic Norm Minimization
- Log in to post comments
- Categories:
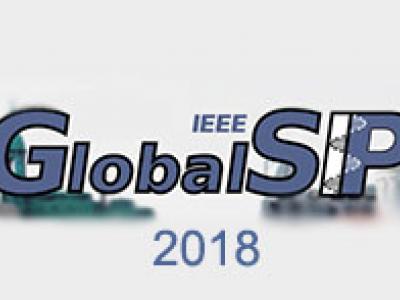
- Read more about The Greedy Dirichlet Process Filter - An Online Clustering Multi-Target Tracker
- Log in to post comments
Reliable collision avoidance is one of the main requirements for autonomous driving.
Hence, it is important to correctly estimate the states of an unknown number of static and dynamic objects in real-time.
Here, data association is a major challenge for every multi-target tracker.
We propose a novel multi-target tracker called Greedy Dirichlet Process Filter (GDPF) based on the non-parametric Bayesian model called Dirichlet Processes and the fast posterior computation algorithm Sequential Updating and Greedy Search (SUGS).
- Categories:
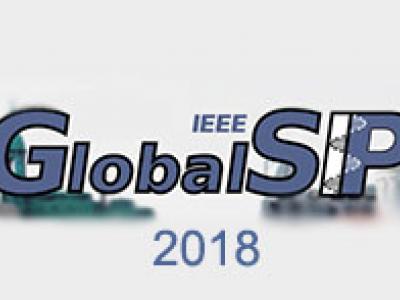
- Read more about OPPORTUNISTIC SCHEDULING IN UNDERLAY COGNITIVE RADIO BASED SYSTEMS: USER SELECTION PROBABILITY ANALYSIS
- Log in to post comments
GlobalSIP.pdf

- Categories:
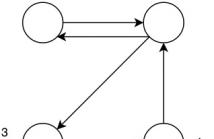
In this paper, we discuss the problem of modeling a graph signal on a directed graph when observing only partially the graph signal. The graph signal is recovered using a learned graph filter. The novelty is to use the random walk operator associated to an ergodic random walk on the graph, so as to define and learn a graph filter, expressed as a polynomial of this operator. Through the study of different cases, we show the efficiency of the signal modeling using the random walk operator compared to existing methods using the adjacency matrix or ignoring the directions in the graph.
- Categories:
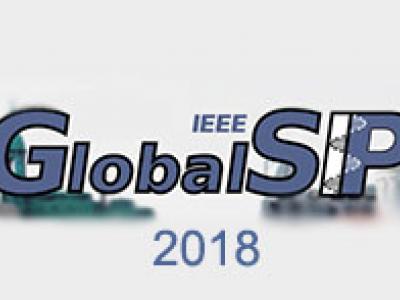
- Read more about Polar Feature Based Deep Architectures for Automatic Modulation Classification Considering Channel Fading
- Log in to post comments
To develop intelligent receivers, automatic modulation classification (AMC) plays an important role for better spectrum utilization. The emerging deep learning (DL) technique has received much attention in AMC due to its superior performance in classifying data with deep structure. In this work, a novel polar-based deep learning architecture with channel compensation network (CCN) is proposed. Our test results show that learning features from polar domain (r-θ) can improve recognition accuracy by 5% and reduce training overhead by 48%.
- Categories: