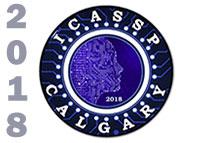
ICASSP is the world’s largest and most comprehensive technical conference focused on signal processing and its applications. The 2019 conference will feature world-class presentations by internationally renowned speakers, cutting-edge session topics and provide a fantastic opportunity to network with like-minded professionals from around the world. Visit website.
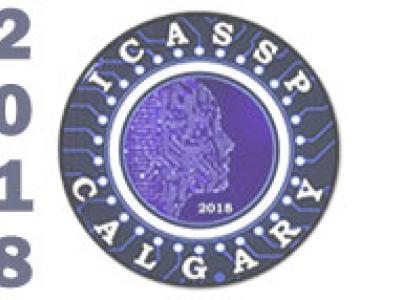
- Read more about MULTI-KERNEL, DEEP NEURAL NETWORK AND HYBRID MODELS FOR PRIVACY PRESERVING MACHINE LEARNING
- Log in to post comments
The rapid rise of IoT and Big Data can facilitate the use of data to enhance our quality of life. However, the omnipresent and sensitive nature of data can simultaneously generate privacy concerns. Hence, there is a strong need to develop techniques that ensure the data serve the intended purposes, but not for prying into one’s sensitive information. We address this challenge via utility maximizing lossy compression of data.
Poster.pdf

- Categories:
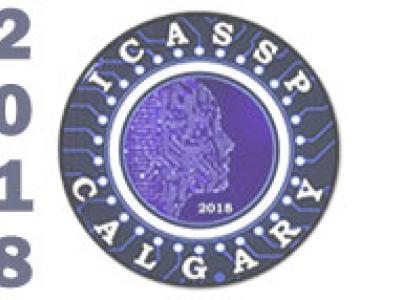
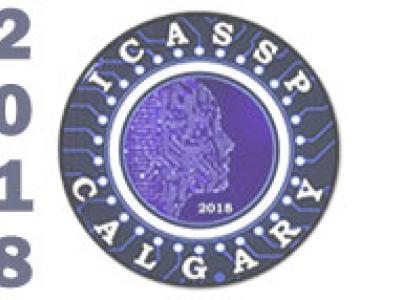
- Read more about OUTLIER REMOVAL FOR ENHANCING KERNEL-BASED CLASSIFIER VIA THE DISCRIMINANT INFORMATION
- Log in to post comments
Pattern recognition on big data can be challenging for kernel machines as the complexity grows with the squared number of training samples. In this work, we overcome this hurdle via the outlying data sample removal pre-processing step. This approach removes less informative data samples and trains the kernel machines only with the remaining data, and hence, directly reduces the complexity by reducing the number of training samples. To enhance the classification performance, the outlier removal process is done such that the discriminant information of the data is mostly intact.
- Categories:
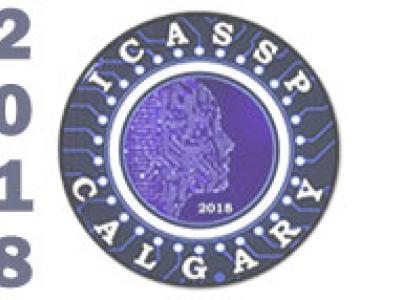
- Categories:
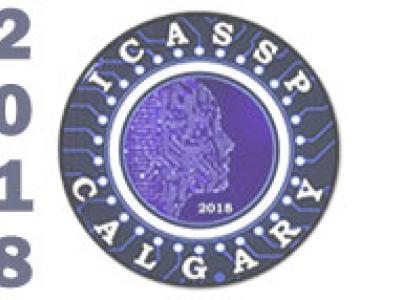
- Read more about DEEP MULTIMODAL LEARNING FOR EMOTION RECOGNITION IN SPOKEN LANGUAGE
- Log in to post comments
In this paper, we present a novel deep multimodal framework to predict human emotions based on sentence-level spoken language. Our architecture has two distinctive characteristics. First, it extracts the high-level features from both text and audio via a hybrid deep multimodal structure, which considers the spatial information from text, temporal information from audio, and high-level associations from low-level handcrafted features.
- Categories:
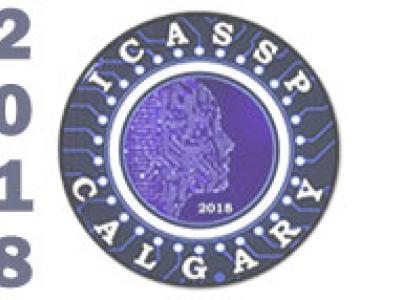
- Read more about TWO-STAGE IDENTIFICATION OF LOCALLY STATIONARY AUTOREGRESSIVE PROCESSES AND ITS APPLICATION TO THE PARAMETRIC SPECTRUM ESTIMATION
- Log in to post comments
The problem of identification of a nonstationary autoregressive process
with unknown, and possibly time-varying, rate of parameter
changes, is considered and solved using the parallel estimation approach.
The proposed two-stage estimation scheme, which combines
the local estimation approach with the basis function one, offers
both quantitative and qualitative improvements compared with
the currently used single-stage methods.
- Categories:
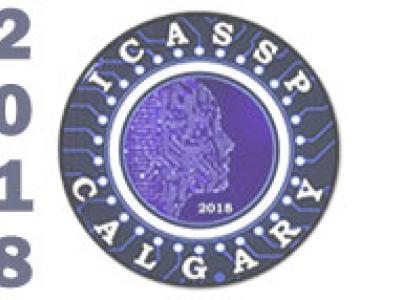
- Read more about Particle flow particle filter for Gaussian mixture noise models
- Log in to post comments
Particle filters has become a standard tool for state estimation in nonlinear systems. However, their performance usually deteriorates if the dimension of state space is high or the measurements are highly informative. A major challenge is to construct a proposal density that is well matched to the posterior distribution. Particle flow methods are a promising option for addressing this task. In this paper, we develop a particle flow particle filter algorithm to address the case where both the process noise and the measurement noise are distributed as mixtures of Gaussians.
- Categories:
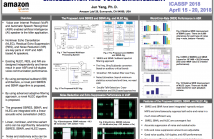
- Read more about MULTILAYER ADAPTATION BASED COMPLEX ECHO CANCELLATION AND VOICE ENHANCEMENT
- Log in to post comments
The paper proposes an efficient signal processing system mainly consisting of an adaptation-based nonlinear echo cancellation (NLEC) layer and a joint perceptual subband residual echo suppression (SBRES) layer and noise reduction (SBNR) layer. The theoretical analyses, subjective and objective test results show that the proposed signal processing system can offer a significant improvement for automatic speech recognition and full-duplex voice communication performance in emerging artificial intelligence speakers.
- Categories:
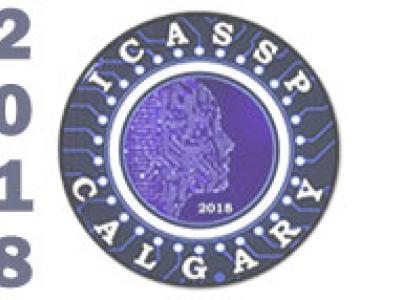
- Read more about FAST 3D-HEVC DEPTH MAPS INTRA-FRAME PREDICTION USING DATA MINING
- Log in to post comments
This paper presents a fast 3D-High Efficiency Video Coding (3D-HEVC) depth maps intra-frame prediction based on static Coding Unit (CU) splitting decisions trees. This coding approach uses data mining to extract the correlation among the encoder context attributes and to define a split decision tree for each CU level of the depth maps encoding. The decision trees were trained using the information extracted from 3D-HEVC Test Model (3D-HTM) and using the Common Test Conditions (CTC).
- Categories:
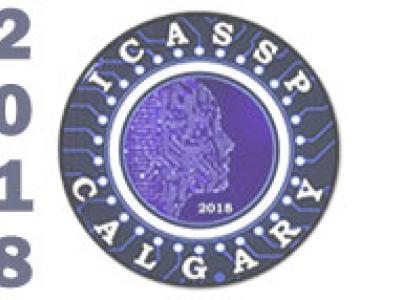
- Categories: