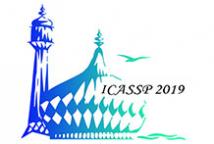
ICASSP is the world’s largest and most comprehensive technical conference focused on signal processing and its applications. The 2019 conference will feature world-class presentations by internationally renowned speakers, cutting-edge session topics and provide a fantastic opportunity to network with like-minded professionals from around the world. Visit website.
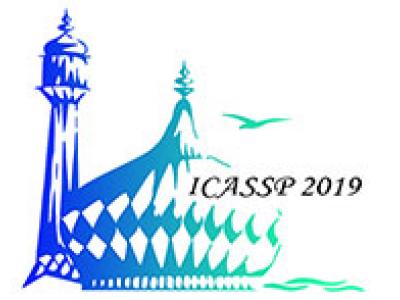
- Read more about DEEP POLYPHONIC ADSR PIANO NOTE TRANSCRIPTION
- Log in to post comments
- Categories:
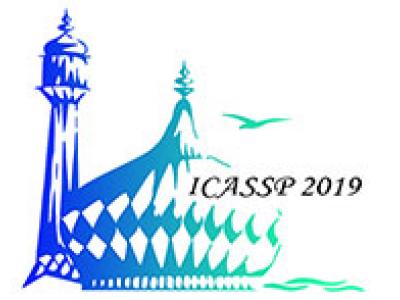
- Read more about Speech Emotion Recognition Using Deep Neural Network Considering Verbal and Nonverbal Speech Sounds
- Log in to post comments
Speech emotion recognition is becoming increasingly important for many applications. In real-life communication, non-verbal sounds within an utterance also play an important role for people to recognize emotion. In current studies, only few emotion recognition systems considered nonverbal sounds, such as laughter, cries or other emotion interjection, which naturally exists in our daily conversation. In this work, both verbal and nonverbal sounds within an utterance were thus considered for emotion recognition of real-life conversations.
- Categories:
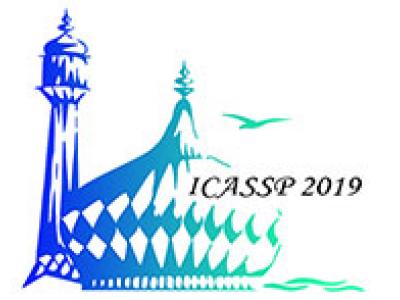
- Read more about Deep learning for Minimal Context Classification of Block-types through Side-Channel Analysis
- Log in to post comments
It is well known that electromagnetic and power side-channel attacks allow extraction of unintended information from a computer processor. However, little work has been done to quantify how small a sample is needed in order to glean meaningful information about a program's execution. This paper quantifies this minimum context by training a deep-learning model to track and classify program block types given small windows of side-channel data. We show that a window containing approximately four clock cycles suffices to predict block type with our experimental setup.
- Categories:
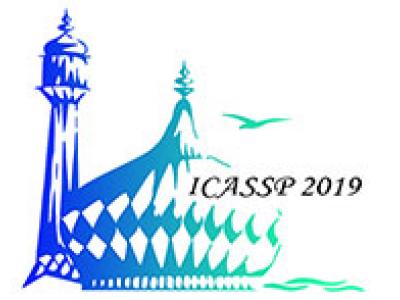
- Read more about AUTOMATIC RADAR-BASED GESTURE DETECTION VIA REGION-BASED DCNN
- Log in to post comments
In this paper, a region-based deep convolutional neural network
(R-DCNN) is proposed to detect and classify gestures
measured by a frequency-modulated continuous wave radar
system. Micro-Doppler (μD) signatures of gestures are exploited,
and the resulting spectrograms are fed into a neural
network. We are the first to use the R-DCNN for radar-based
gesture recognition, such that multiple gestures could be automatically
detected and classified without manually clipping
the data streams according to each hand movement in advance.
- Categories:
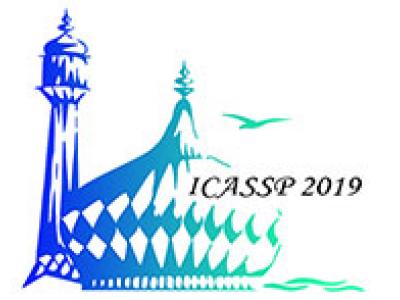
Methods based on sparse representation have found great use in the recovery of audio signals degraded by clipping. The state of the art in declipping within the sparsity-based approaches has been achieved by the SPADE algorithm by Kitić et. al. (LVA/ICA’15). Our recent study (LVA/ICA’18) has shown that although the original S-SPADE can be improved such that it converges faster than the A-SPADE, the restoration quality is significantly worse. In the present paper, we propose a new version of S-SPADE.
- Categories:
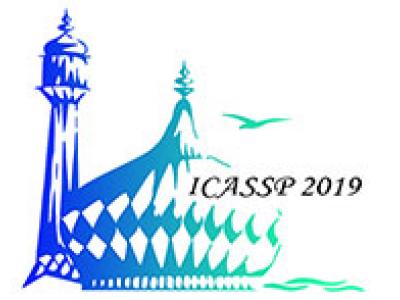
- Read more about EVALUATION OF NON-INTRUSIVE LOAD MONITORING ALGORITHMS FOR APPLIANCE-LEVEL ANOMALY DETECTION
- Log in to post comments
- Categories:
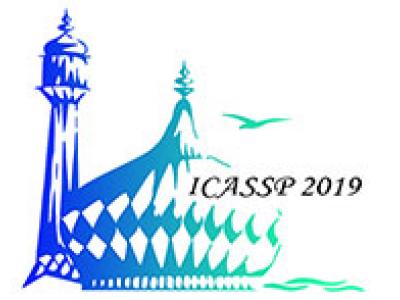
- Read more about Image Reflection Removal Using The Wasserstein Generative Adversarial Network
- Log in to post comments
Imaging through a semi-transparent material such as glass often suffers from the reflection problem, which degrades the image quality. Reflection removal is a challenging task since it is severely ill-posed. Traditional methods, while all require long computation time on minimizing different objective functions with huge matrices, do not necessarily give satisfactory performance. In this paper, we propose a novel deep-learning based method to allow fast removal of reflection.
- Categories:
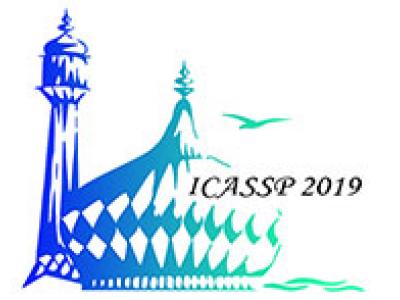
- Read more about 3D reconstruction using single-photon Lidar data: exploiting the widths of the returns
- Log in to post comments
Single-photon light detection and ranging (Lidar) data can be used to capture depth and intensity profiles of a 3D scene. In a general setting, the scenes can have an unknown number of surfaces per pixel (semi-transparent surfaces or outdoor measurements), high background noise (strong ambient illumination), can be acquired by systems with a broad instrumental response (non-parallel laser beam with respect to the target surface) and with possibly high attenuating media (underwater conditions).
ICASSP19.pptx

- Categories:
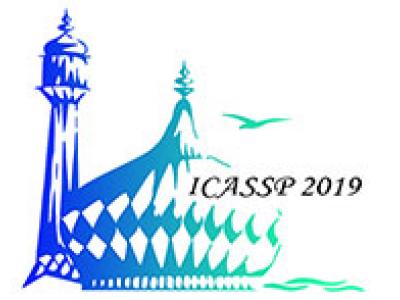
- Read more about A Subject-to-Subject Transfer Learning Framework based on Jensen-Shannon divergence for Improving Brain-computer Interface
- Log in to post comments
One of the major limitations of current electroencephalogram (EEG)-based brain-computer interfaces (BCIs) is the long calibration time. Due to a high level of noise and non-stationarity inherent in EEG signals, a calibration model trained using the limited number of train data may not yield an accurate BCI model. To address this problem, this paper proposes a novel subject-to-subject transfer learning framework that improves the classification accuracy using limited training data.
- Categories:
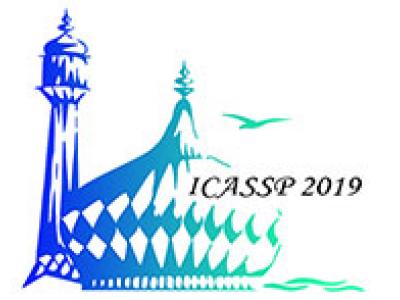
- Read more about Subband Temporal Envelope Features and Data Augmentation for End-to-end Recognition of Distant Conversational Speech
- Log in to post comments
This paper investigates the use of subband temporal envelope (STE) features and speed perturbation based data augmentation in end-to-end recognition of distant conversational speech in everyday home environments. STE features track energy peaks in perceptual frequency bands which reflect the resonant properties of the vocal tract. Data augmentation is performed by adding more training data obtained after modifying the speed of the original training data.
- Categories: