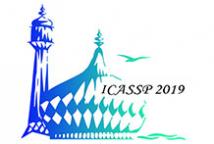
ICASSP is the world’s largest and most comprehensive technical conference focused on signal processing and its applications. The 2019 conference will feature world-class presentations by internationally renowned speakers, cutting-edge session topics and provide a fantastic opportunity to network with like-minded professionals from around the world. Visit website.
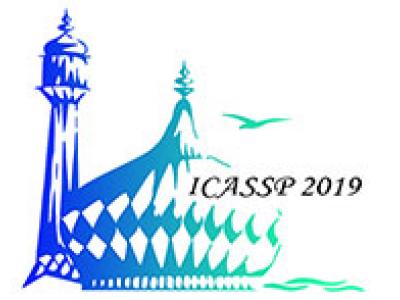
- Read more about PRUNING SIFT & SURF FOR EFFICIENT CLUSTERING OF NEAR-DUPLICATE IMAGES
- Log in to post comments
Clustering and categorization of similar images using SIFT and SURF require a high computational cost. In this paper, a simple approach to reduce the cardinality of keypoint set and prune the dimension of SIFT and SURF feature descriptors for efficient image clustering is proposed. For this purpose, sparsely spaced (uniformly distributed) important keypoints are chosen. In addition, multiple reduced dimensional variants of SIFT and SURF descriptors are presented.
- Categories:
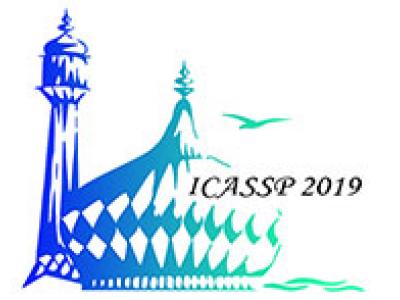
- Read more about Connectionist Temporal Localization for Sound Event Detection with Sequential Labeling
- Log in to post comments
Research on sound event detection (SED) with weak labeling has mostly focused on presence/absence labeling, which provides no temporal information at all about the event occurrences. In this paper, we consider SED with sequential labeling, which specifies the temporal order of the event boundaries. The conventional connectionist temporal classification (CTC) framework, when applied to SED with sequential labeling, does not localize long events well due to a "peak clustering" problem.
Poster.pdf

- Categories:
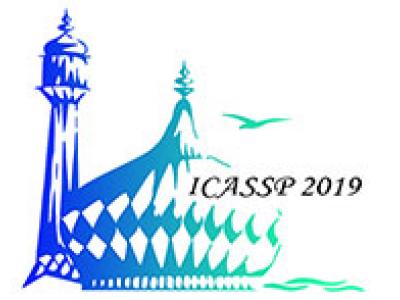
- Read more about Video-Based, Occlusion-Robust Multi-View Stereo Using Inner-Boundary Depths of Textureless Areas
- Log in to post comments
Occlusions and poor textures are two main problems in multi-view stereo reconstruction. This paper presents a video-based solution to address both challenges in depth estimation. We focus on reconstructing accurate inner boundaries of visible textureless areas, particularly for occluded background, by leveraging the reliable depths of object edges. This is done by efficiently respecting two local cues with complementary advantages, i.e. smoothness and density of recovered surfaces.
ICASSP2019.pdf

- Categories:
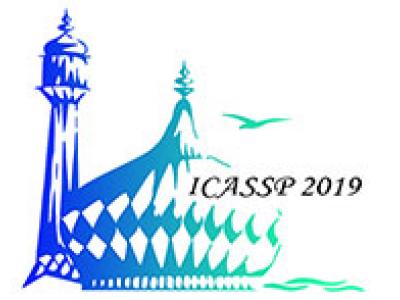
- Read more about EMBEDDING PHYSICAL AUGMENTATION AND WAVELET SCATTERING TRANSFORM TO GENERATIVE ADVERSARIAL NETWORKS FOR AUDIO CLASSIFICATION WITH LIMITED TRAINING RESOURCES
- Log in to post comments
This paper addresses audio classification with limited training resources. We first investigate different types of data augmentation including physical modeling, wavelet scattering transform and Generative Adversarial Networks (GAN). We than propose a novel GAN which allows embedding of physical augmentation and wavelet scattering transform in processing. The experimental results on Google Speech Command show significant improvements of the proposed method when training with limited resources.
- Categories:
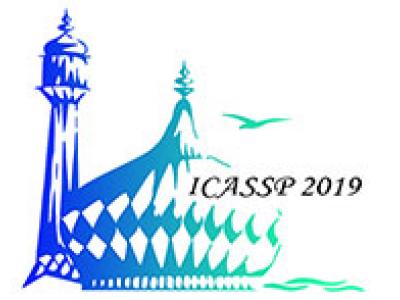
- Read more about MULTI-BAND PIT AND MODEL INTEGRATION FOR IMPROVED MULTI-CHANNEL SPEECH SEPARATION
- Log in to post comments
- Categories:
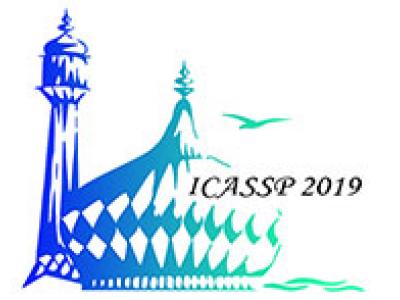
- Read more about Knowledge Distillation Using Output Errors for Self-Attention ASR Models
- Log in to post comments
Most automatic speech recognition (ASR) neural network models are not suitable for mobile devices due to their large model sizes. Therefore, it is required to reduce the model size to meet the limited hardware resources. In this study, we investigate sequence-level knowledge distillation techniques of self-attention ASR models for model compression.
- Categories:
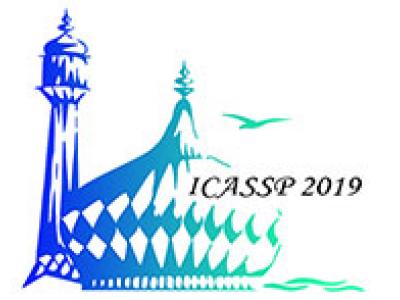
- Read more about ADVERSARIAL MULTI-TASK DEEP FEATURES AND UNSUPERVISED BACK-END ADAPTATION FOR LANGUAGE RECOGNITION
- Log in to post comments
- Categories:
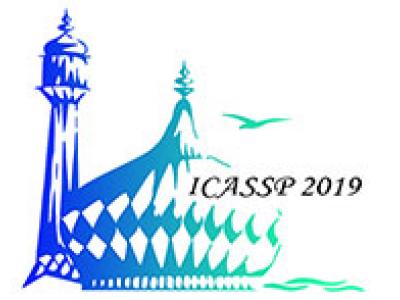
- Read more about Transform Domain based Medical Image Super-Resolution via Deep Multi-scale Network
- Log in to post comments
This paper proposes a new medical image super-resolution (SR) network, namely deep multi-scale network (DMSN), in the uniform discrete curvelet transform (UDCT) domain. DMSN is made up of a set of cascaded multi-scale fushion (MSF) blocks. In each MSF block, we use convolution kernels of different sizes to adaptively detect the local multiscale feature, and then local residual learning (LRL) is used to learn effective feature from preceding MSF block and current multi-scale features.
poster.pdf

- Categories:
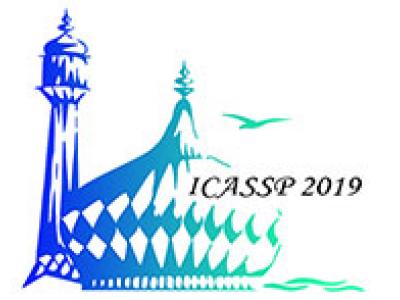
- Read more about Signals and Systems:Casting it as an Action-Adventure rather than a Horror Genre
- Log in to post comments
Simple but effective strategies for an undergraduate introductory course in signals and systems are described in this paper. These include peer facilitated tutorials, optional class tests, in-class only lab assessment and use of interactive animations. Peer facilitated tutorials were designed to support students to help other students. The optional class tests removed the stress and anxiety students face. With in-class only lab assessment the time students spent writing lab reports was replaced with time devoted to preparing and doing the lab together as a group.
- Categories:
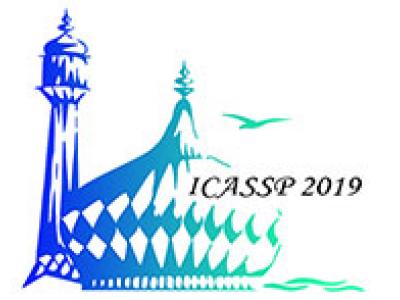
- Read more about Graph Filtering with Multiple Shift Matrices
- Log in to post comments
- Categories: