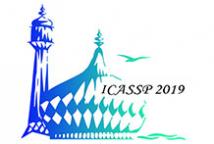
ICASSP is the world’s largest and most comprehensive technical conference focused on signal processing and its applications. The 2019 conference will feature world-class presentations by internationally renowned speakers, cutting-edge session topics and provide a fantastic opportunity to network with like-minded professionals from around the world. Visit website.
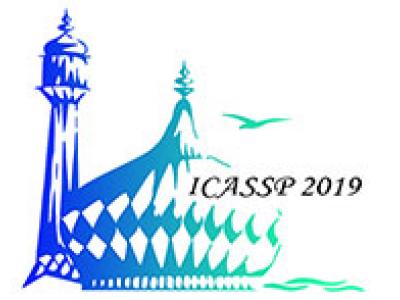
- Read more about POSTER OF PAPER 3809 (SLP-P20)
- Log in to post comments
Poster presented at the poster session "Speech Synthesis II" of ICASSP 2019 of the paper "ENHANCED VIRTUAL SINGERS GENERATION BY INCORPORATING SINGING DYNAMICS TO PERSONALIZED TEXT-to-SPEECH-to-SINGING"
- Categories:
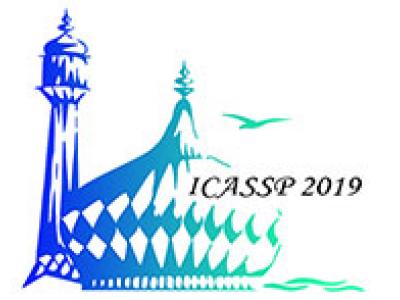
- Read more about Toward Robust Interpretable Human Movement Pattern Analysis in a Workplace Setting
- Log in to post comments
Gaining a better understanding of how people move about and interact with their environment is an important piece of understanding human behavior. Careful analysis of individuals’ deviations or variations in movement over time can provide an awareness about changes to their physical or mental state and may be helpful in tracking performance and well-being especially in workplace settings. We propose a technique for clustering and discovering patterns in human movement data by extracting motifs from the time series of durations where participants linger at different locations.
- Categories:
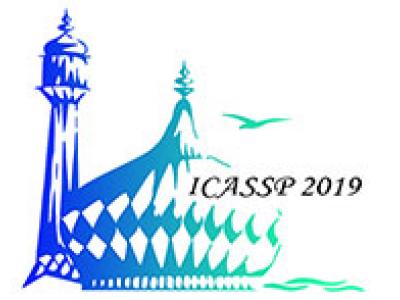
While neural networks have achieved vastly enhanced performance over traditional iterative methods in many cases, they are generally empirically designed and the underlying structures are difficult to interpret. The algorithm unrolling approach has helped connect iterative algorithms to neural network architectures. However, such connections have not been made yet for blind image deblurring. In this paper, we propose a neural network architecture that advances this idea.
- Categories:
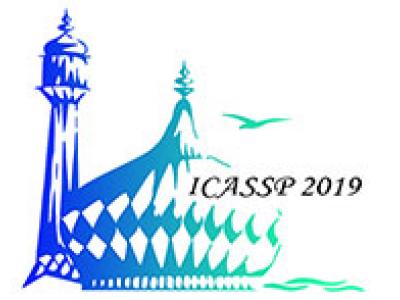
- Read more about Solving Complex Quadratic Equations with Full-rank Random Gaussian Matrices
- Log in to post comments
We tackle the problem of recovering a complex signal $\mathbf{x}\in\mathbb{C}^n$ from quadratic measurements of the form $y_i=\mathbf{x}^*\mathbf{A}_i\mathbf{x}$, where $\{\mathbf{A}_i\}_{i=1}^m$ is a set of complex iid standard Gaussian matrices. This non-convex problem is related to the well understood phase retrieval problem where $\mathbf{A}_i$ is a rank-1 positive semidefinite matrix.
- Categories:
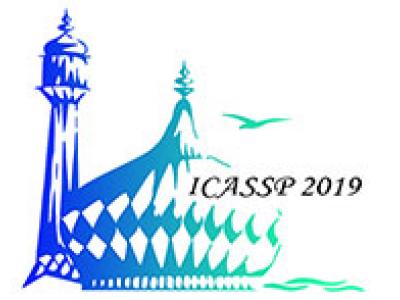
- Read more about TIME SERIES PREDICTION FOR KERNEL-BASED ADAPTIVE FILTERS USING VARIABLE BANDWIDTH, ADAPTIVE LEARNING-RATE, AND DIMENSIONALITY REDUCTION
- Log in to post comments
Kernel-based adaptive filters are sequential learning algorithms, operating on reproducing kernel Hilbert spaces. Their learning performance is susceptible to the selection of appropriate values for kernel bandwidth and learning-rate parameters. Additionally, as these algorithms train the model using a sequence of input vectors, their computation scales with the number of samples. We propose a framework that addresses the previous open challenges of kernel-based adaptive filters.
- Categories:
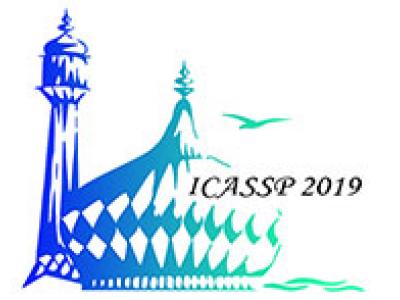
- Read more about ENHANCING BEAMFORMED FINGERPRINT OUTDOOR POSITIONING WITH HIERARCHICAL CONVOLUTIONAL NEURAL NETWORKS
- Log in to post comments
poster.pdf

- Categories:
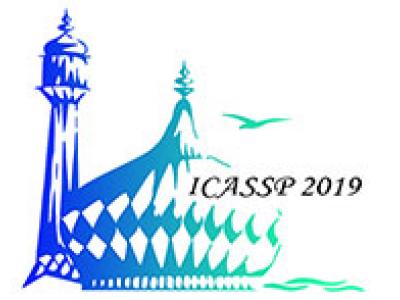
- Read more about Point Cloud Segmentation using Hierarchical Tree for Architectural Models.
- Log in to post comments
Over the past few years, gathering massive volume of 3D data has become straightforward due to the proliferation of laser scanners and acquisition devices. Segmentation of such large data into meaningful segments, however, remains a challenge. Raw scans usually have missing data and varying density. In this work, we present a simple yet effective method to semantically decompose and reconstruct 3D models from point clouds. Using a hierarchical tree approach, we segment and reconstruct planar as well as non-planar scenes in an outdoor environment.
- Categories:
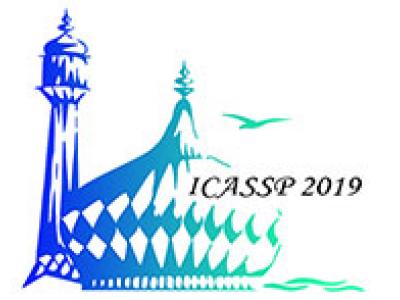
- Read more about ENLLVM: Ensemble based Nonlinear Bayesian Filtering using Linear Latent Variable Models
- Log in to post comments
Real-time nonlinear Bayesian filtering algorithms are overwhelmed by data volume, velocity and increasing complexity of computational models. In this paper, we propose a novel ensemble based nonlinear Bayesian filtering approach which only requires a small number of simulations and can be applied to high-dimensional systems in the presence of intractable likelihood functions.
- Categories:
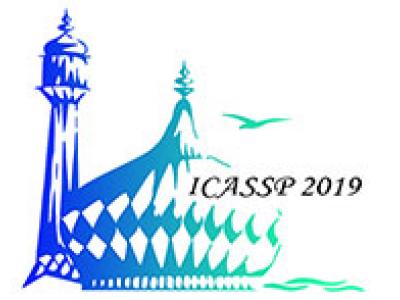
- Read more about Improving Human-Computer Interaction in Low-Resource Settings with Text-to-Phonetic Data Augmentation
- Log in to post comments
Off-the-shelf speech recognizers are error-prone in specialized domains; we aim to mitigate the impact of these errors for downstream classification tasks without in-domain speech training data, by augmenting available typewritten text training data with inferred phonetic information. We apply our method to mitigate the effects of the lack of speech training data when converting a typed chatbot to a spoken language interface.
Paper available here: https://ieeexplore.ieee.org/document/8682550
- Categories:
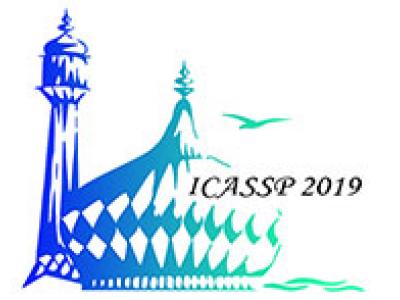
- Read more about Deep Speaker Embedding Learning with Multi-Level Pooling for Text-Independent Speaker Verification
- Log in to post comments
This paper aims to improve the widely used deep speaker embedding x-vector model. We propose the following improvements: (1) a hybrid neural network structure using both time delay neural network (TDNN) and long short-term memory neural networks (LSTM) to generate complementary speaker information at different levels; (2) a multi-level pooling strategy to collect speaker information from both TDNN and LSTM layers; (3) a regularization scheme on the speaker embedding extraction layer to make the extracted embeddings suitable for the following fusion step.
- Categories: