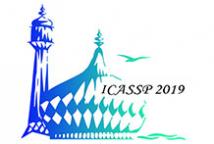
ICASSP is the world’s largest and most comprehensive technical conference focused on signal processing and its applications. The 2019 conference will feature world-class presentations by internationally renowned speakers, cutting-edge session topics and provide a fantastic opportunity to network with like-minded professionals from around the world. Visit website.
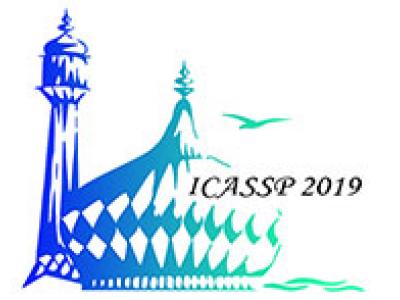
- Read more about Dimensional Analysis of Laughter in Female Conversational Speech
- Log in to post comments
How do people hear laughter in expressive, unprompted speech? What is the range of expressivity and function of laughter in this speech, and how can laughter inform the recognition of higher-level expressive dimensions in a corpus? This paper presents a scalable method for collecting natural human description of laughter, transforming the description to a vector of quantifiable laughter dimensions, and deriving baseline classifiers for the different dimensions of expressive laughter.
- Categories:
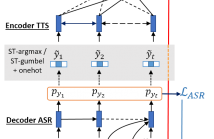
- Read more about END-TO-END FEEDBACK LOSS IN SPEECH CHAIN FRAMEWORK VIA STRAIGHT-THROUGH ESTIMATOR
- Log in to post comments
The speech chain mechanism integrates automatic speech recognition (ASR) and text-to-speech synthesis (TTS) modules into a single cycle during training. In our previous work, we applied a speech chain mechanism as a semi-supervised learning. It provides the ability for ASR and TTS to assist each other when they receive unpaired data and let them infer the missing pair and optimize the model with reconstruction loss.
- Categories:
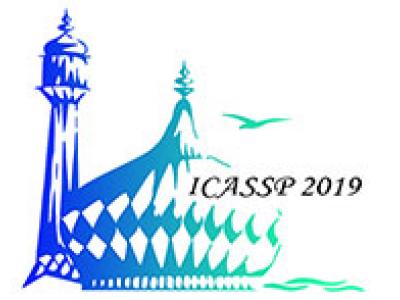
- Read more about BLHUC: BAYESIAN LEARNING OF HIDDEN UNIT CONTRIBUTIONS FOR DEEP NEURAL NETWORK SPEAKER ADAPTATION
- Log in to post comments
- Categories:
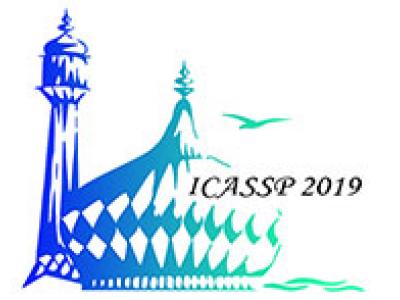
- Read more about Joint Separation and Dereverberation of Reverberant Mixture with Multichannel Variational Autoencoder
- Log in to post comments
AASP_L4_2.pdf

- Categories:
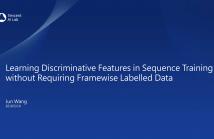
- Read more about Learning Discriminative Features in Sequence Training without Requiring Framewise Labelled Data
- Log in to post comments
Slides.pdf

- Categories:
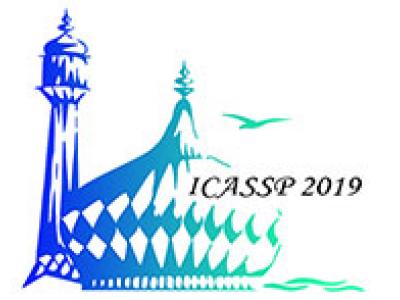
- Read more about INDUCTIVE CONFORMAL PREDICTOR FOR SPARSE CODING CLASSIFIERS: APPLICATIONS TO IMAGE CLASSIFICATION
- Log in to post comments
Conformal prediction uses the degree of strangeness (nonconformity) of new data instances to determine the confidence values of new predictions. We propose an inductive conformal predictor for sparse coding classifiers, referred to as ICP-SCC. Our contribution is twofold: first, we present two nonconformitymeasures that produce reliable confidence values; second, we propose a batchmode active learning algorithm within the conformal prediction framework to improve classification performance by selecting training instances based on two criteria, informativeness and diversity.
- Categories:
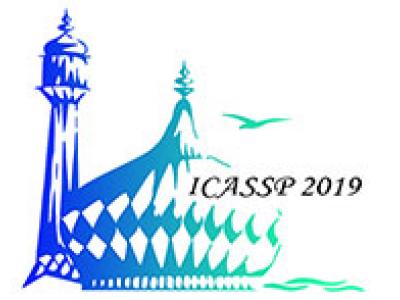
- Read more about Phoneme Level Language Models for Sequence Based Low Resource ASR
- Log in to post comments
Building multilingual and crosslingual models help bring different languages together in a language universal space. It allows models to share parameters and transfer knowledge across languages, enabling faster and better adaptation to a new language. These approaches are particularly useful for low resource languages. In this paper, we propose a phoneme-level language model that can be used multilingually and for crosslingual adaptation to a target language.
- Categories:
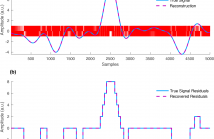
- Read more about One-Bit Unlimited Sampling
- Log in to post comments
Conventional analog–to–digital converters (ADCs) are limited in dynamic range. If a signal exceeds some prefixed threshold, the ADC saturates and the resulting signal is clipped, thus becoming prone to aliasing artifacts. Recent developments in ADC design allow to overcome this limitation: using modulo operation, the so called self-reset ADCs fold amplitudes which exceed the dynamic range. A new (unlimited) sampling theory is currently being developed in the context of this novel class of ADCs.
- Categories:
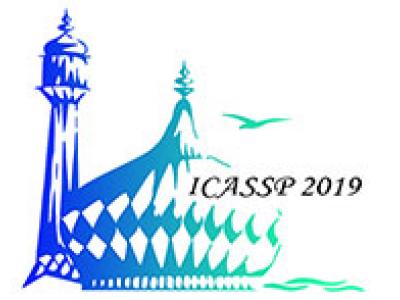
- Read more about Subword Regularization and Beam Search Decoding for End-to-End Automatic Speech Recognition
- Log in to post comments
In this paper, we experiment with the recently introduced subword regularization technique \cite{kudo2018subword} in the context of end-to-end automatic speech recognition (ASR). We present results from both attention-based and CTC-based ASR systems on two common benchmark datasets, the 80 hour Wall Street Journal corpus and 1,000 hour Librispeech corpus. We also introduce a novel subword beam search decoding algorithm that significantly improves the final performance of the CTC-based systems.
- Categories: