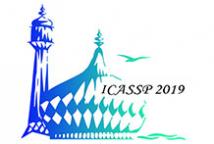
ICASSP is the world’s largest and most comprehensive technical conference focused on signal processing and its applications. The 2019 conference will feature world-class presentations by internationally renowned speakers, cutting-edge session topics and provide a fantastic opportunity to network with like-minded professionals from around the world. Visit website.
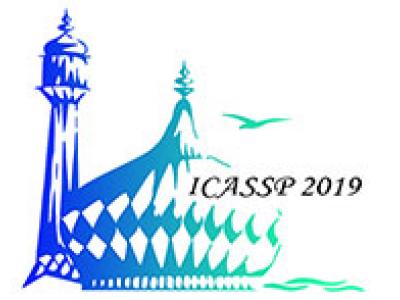
- Read more about A Pipeline for Lung Tumor Detection and Segmentation from CT Scans using Dilated Convolutional Neural Networks
- Log in to post comments
Lung cancer is the most prevalent cancer worldwide with about 230,000 new cases every year. Most cases go undiagnosed until it’s too late, especially in developing countries and remote areas. Early detection is key to beating cancer. Towards this end, the work presented here proposes an automated pipeline for lung tumor detection and segmentation from 3D lung CT scans from the NSCLC Radiomics Dataset. It also presents a new dilated hybrid-3D convolutional neural network architecture for tumor segmentation. First, a binary classifier chooses CT scan slices that may contain parts of a tumor.
- Categories:
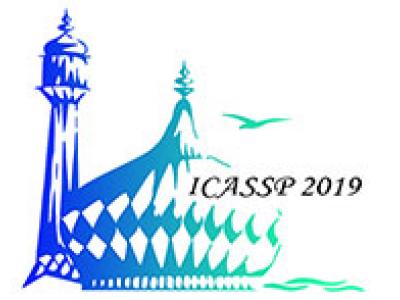
- Read more about HIERARCHY-AWARE LOSS FUNCTION ON A TREE STRUCTURED LABEL SPACE FOR AUDIO EVENT DETECTION
- Log in to post comments
- Categories:
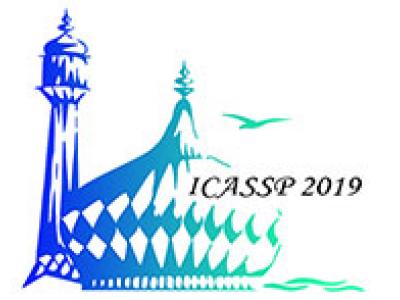
- Read more about The discrete cosine transform on triangles
- Log in to post comments
- Categories:
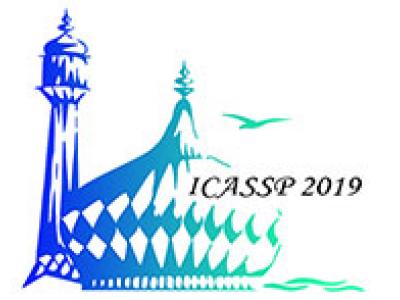
- Read more about DEEP SPEAKER REPRESENTATION USING ORTHOGONAL DECOMPOSITION AND RECOMBINATION FOR SPEAKER VERIFICATION
- Log in to post comments
Speech signal contains intrinsic and extrinsic variations such as accent, emotion, dialect, phoneme, speaking manner, noise, music, and reverberation. Some of these variations are unnecessary and are unspecified factors of variation. These factors lead to increased variability in speaker representation. In this paper, we assume that unspecified factors of variation exist in speaker representations, and we attempt to minimize variability in speaker representation.
- Categories:
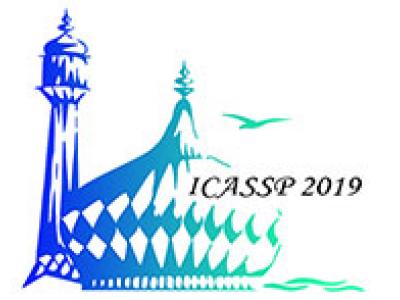
- Read more about Adversarial Speaker Adaptation
- Log in to post comments
We propose a novel adversarial speaker adaptation (ASA) scheme, in which adversarial learning is applied to regularize the distribution of deep hidden features in a speaker-dependent (SD) deep neural network (DNN) acoustic model to be close to that of a fixed speaker-independent (SI) DNN acoustic model during adaptation. An additional discriminator network is introduced to distinguish the deep features generated by the SD model from those produced by the SI model.
- Categories:
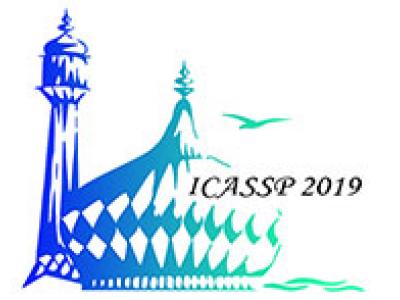
- Read more about Attentive Adversarial Learning for Domain-Invariant Training
- Log in to post comments
Adversarial domain-invariant training (ADIT) proves to be effective in suppressing the effects of domain variability in acoustic modeling and has led to improved performance in automatic speech recognition (ASR). In ADIT, an auxiliary domain classifier takes in equally-weighted deep features from a deep neural network (DNN) acoustic model and is trained to improve their domain-invariance by optimizing an adversarial loss function.
- Categories:
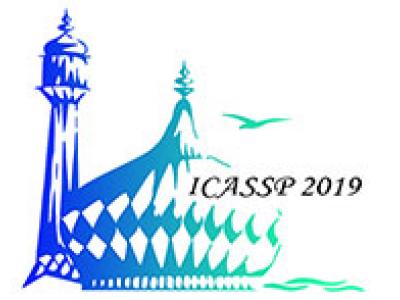
- Read more about Adversarial Speaker Verification
- Log in to post comments
The use of deep networks to extract embeddings for speaker recognition has proven successfully. However, such embeddings are susceptible to performance degradation due to the mismatches among the training, enrollment, and test conditions. In this work, we propose an adversarial speaker verification (ASV) scheme to learn the condition-invariant deep embedding via adversarial multi-task training. In ASV, a speaker classification network and a condition identification network are jointly optimized to minimize the speaker classification loss and simultaneously mini-maximize the condition loss.
- Categories:
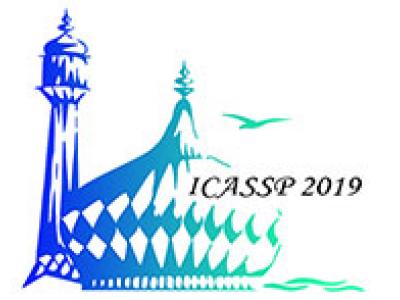
- Read more about Conditional Teacher-Student Learning
- Log in to post comments
The teacher-student (T/S) learning has been shown to be effective for a variety of problems such as domain adaptation and model compression. One shortcoming of the T/S learning is that a teacher model, not always perfect, sporadically produces wrong guidance in form of posterior probabilities that misleads the student model towards a suboptimal performance.
- Categories:
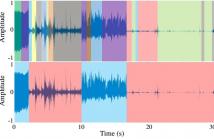
- Read more about QUALITY CONTROL OF VOICE RECORDINGS IN REMOTE PARKINSON'S DISEASE MONITORING USING THE INFINITE HIDDEN MARKOV MODEL
- Log in to post comments
The performance of voice-based systems for remote monitoring of Parkinson’s disease is highly dependent on the degree of adherence of the recordings to the test protocols, which probe for specific symptoms. Identifying segments of the signal that adhere to the protocol assumptions is typically performed manually by experts. This process is costly, time consuming, and often infeasible for large-scale data sets. In this paper, we propose a method to automatically identify the segments of signals that violate the test protocol with a high accuracy.
- Categories:
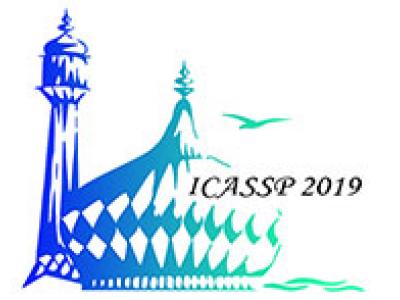
- Read more about ADVERSARIAL INPAINTING OF MEDICAL IMAGE MODALITIES
- Log in to post comments
- Categories: