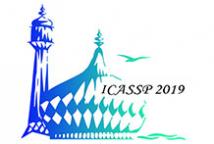
ICASSP is the world’s largest and most comprehensive technical conference focused on signal processing and its applications. The 2019 conference will feature world-class presentations by internationally renowned speakers, cutting-edge session topics and provide a fantastic opportunity to network with like-minded professionals from around the world. Visit website.
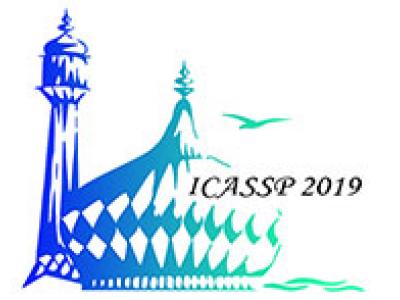
- Read more about A Non-Convex Approach to Non-negative Super-Resolution: Theory and Algorithm
- Log in to post comments
QIAO_HENG.pdf

- Categories:
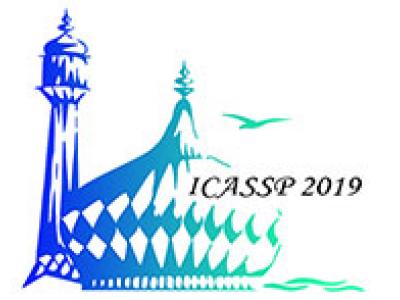
- Read more about Unsuper vised Deep Clustering for Source Separation: Direct Learning from Mixtures Using Spatial Information Slides
- Log in to post comments
We present a monophonic source separation system that is trained by only observing mixtures with no ground truth separation information. We use a deep clustering approach which trains on multi-channel mixtures and learns to project spectrogram bins to source clusters that correlate with various spatial features. We show that using such a training process we can obtain separation performance that is as good as making use of ground truth separation information.
- Categories:
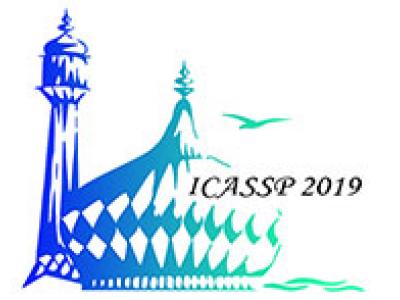
- Read more about Word Characters and Phone Pronunciation Embedding for ASR Confidence Classifier
- Log in to post comments
Confidences are integral to ASR systems, and applied to data selection, adaptation, ranking hypotheses, arbitration etc.Hybrid ASR system is inherently a match between pronunciations and AM+LM evidence but current confidence features lack pronunciation information. We develop pronunciation embeddings to represent and factorize acoustic score in relevant bases, and demonstrate 8-10% relative reduction in false alarm (FA) on large scale tasks. We generalize to standard NLP embeddings like Glove, and show 16% relative reduction in FA in combination with Glove.
- Categories:
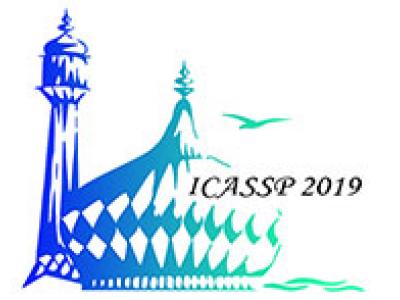
- Read more about CODING TREE EARLY TERMINATION FOR FAST HEVC TRANSRATING BASED ON RANDOM FORESTS
- Log in to post comments
Video transrating has become an essential task in streaming service providers that need to transmit and deliver different versions of the same content for a multitude of users that operate under different network conditions. As the transrating operation is comprised of a decoding and an encoding step in sequence, a huge computational cost is required in such large-scale services, especially when considering the use of complex state-of-the-art codecs, such as the High Efficiency Video Coding (HEVC).
- Categories:
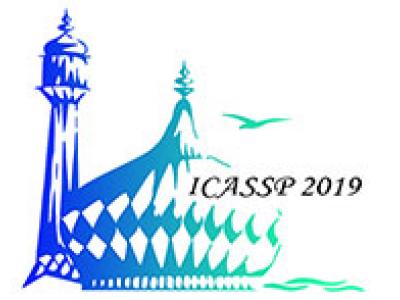
- Read more about Immersive Audio Coding for Virtual Reality Using a Metadata-Assisted Extension of the 3GPP EVS Codec
- Log in to post comments
Virtual Reality (VR) audio scenes may be composed of a very large number of audio elements, including dynamic audio objects, fixed audio channels and scene-based audio elements such as Higher Order Ambisonics (HOA).
VRStream.pdf

- Categories:

- Read more about Introducing the Orthogonal Periodic Sequences for the Identification of Functional Link Polynomial Filters
- Log in to post comments
The paper introduces a novel family of deterministic signals, the orthogonal periodic sequences (OPSs), for the identification of functional link polynomial (FLiP) filters. The novel sequences share many of the characteristics of the perfect periodic sequences (PPSs). As the PPSs, they allow the perfect identification of a FLiP filter on a finite time interval with the cross-correlation method. In contrast to the PPSs, OPSs can identify also non-orthogonal FLiP filters, as the Volterra filters.
poster2.pdf

- Categories:
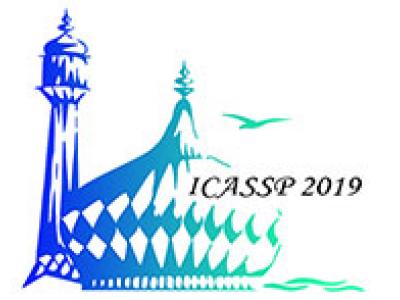
- Read more about Inter- and Intra- Patient ECG Heartbeat Classification For Arrhythmia Detection: a Sequence to Sequence Deep Learning Approach
- Log in to post comments
Electrocardiogram (ECG) signal is a common and powerful tool to study heart function and diagnose several abnormal arrhythmias. While there have been remarkable improvements in cardiac arrhythmia classification methods, they still cannot offer acceptable performance in detecting different heart conditions, especially when dealing with imbalanced datasets. In this paper, we propose a solution to address this limitation of current classification approaches by developing an automatic heartbeat classification method using deep convolutional neural networks and sequence to sequence models.
- Categories:
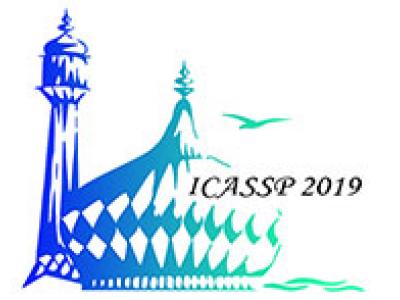
- Read more about Bluetooth based Indoor Localization using Triplet Embeddings
- Log in to post comments
- Categories:
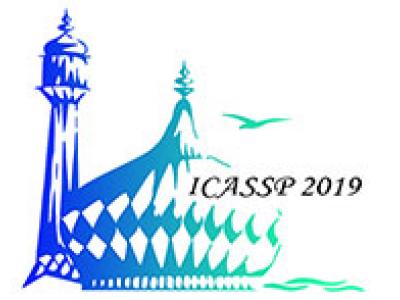
- Read more about Denoising Gravitational Waves with Enhanced Deep Recurrent Denoising Auto-Encoders
- Log in to post comments
- Categories:
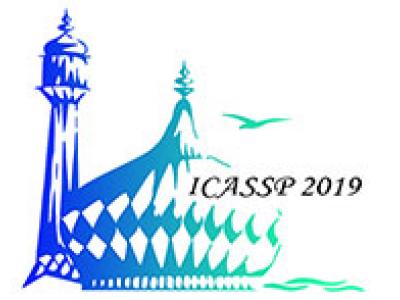
- Read more about End-to-End Anchored Speech Recognition
- Log in to post comments
Voice-controlled house-hold devices, like Amazon Echo or Google Home, face the problem of performing speech recognition of device- directed speech in the presence of interfering background speech, i.e., background noise and interfering speech from another person or media device in proximity need to be ignored. We propose two end-to-end models to tackle this problem with information extracted from the “anchored segment”.
- Categories: