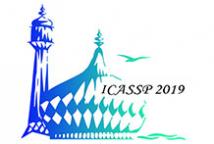
ICASSP is the world’s largest and most comprehensive technical conference focused on signal processing and its applications. The 2019 conference will feature world-class presentations by internationally renowned speakers, cutting-edge session topics and provide a fantastic opportunity to network with like-minded professionals from around the world. Visit website.
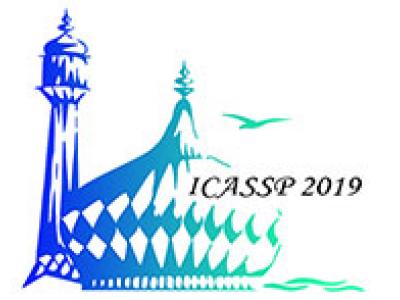
- Read more about RHFCN: Fully CNN-based Steganalysis of MP3 with Rich High-Pass Filtering
- Log in to post comments
Recent studies have shown that convolutional neural networks (CNNs) can boost the performance of audio steganalysis. In this paper, we propose a well-designed fully CNN architecture for MP3 steganalysis based on rich high-pass filtering (HPF). On the one hand, multi-type HPFs are employed for "residual" extraction to enlarge the traces of the signal in view of the truth that signal introduced by secret messages can be seen as high-pass frequency noise.
poster.pdf

- Categories:
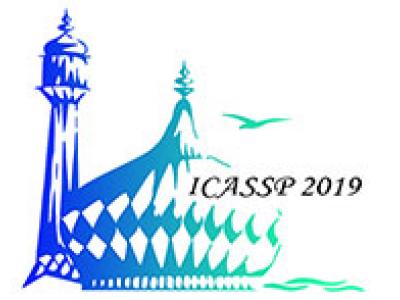
- Read more about ANALYSIS OF COPRIME ARRAYS ON MOVING PLATFORM
- Log in to post comments
Moving platforms enable sparse arrays to assume higher degrees of freedom and lead to increased number of lags. In essence, array motion can fill the holes in the spatial autocorrelation lags associated with a fixed platform and, therefore, increase the number of sources detectable by the same number of physical array sensors. In this paper, we consider coprime arrays, and assume quasi-stationarity of the environment, where the source locations and waveforms are considered invariant over array motion of half wavelength.
- Categories:
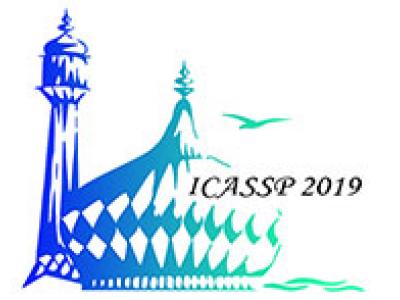
- Read more about Low-complexity Recurrent Neural Network-based Polar Decoder with Weight Quantization Mechanism
- Log in to post comments
Polar codes have drawn much attention and been adopted in 5G New Radio (NR) due to their capacity-achieving performance. Recently, as the emerging deep learning (DL) technique has breakthrough achievements in many fields, neural network decoder was proposed to obtain faster convergence and better performance than belief propagation (BP) decoding. However, neural networks are memory-intensive and hinder the deployment of DL in communication systems. In this work, a low-complexity recurrent neural network (RNN) polar decoder with codebook-based weight quantization is proposed.
- Categories:
- Read more about HOW VIDEO OBJECT TRACKING IS AFFECTED BY IN-CAPTURE DISTORTIONS?
- Log in to post comments
Video Object Tracking -VOT- in realistic scenarios is a difficult task. Image factors such as occlusion, clutter, confusion, object shape, and zooming, among others, have an impact on video tracker methods performance. While these conditions do affect trackers performance, there is not a clear distinction between the scene content challenges like occlusion and clutter, against challenges due to distortions generated by capture, compression, processing, and transmission of videos. This paper is concerned with the latter interpretation of quality as it affects VOT performance.
- Categories:
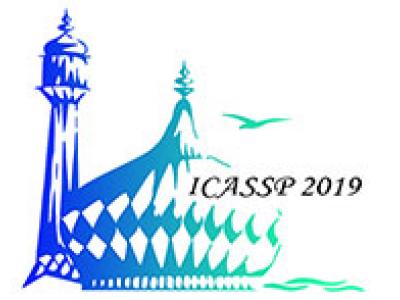
- Read more about SOUND SOURCE LOCALIZATION IN A REVERBERANT ROOM USING HARMONIC BASED MUSIC
- Log in to post comments
The localization of acoustic sound sources is beneficial to signal processing applications of speech enhancement, dereverberation, separation and tracking. Difficulties in position estimation arise in real world environments due to coherent reflections degrading performance of subspace localization techniques. This paper proposes a method of multiple signal classification (MUSIC) subspace localization, which is suitable for reverberant rooms. The method is based on the modal decomposition of a room's region-to-region transfer function, which is assumed to be known.
- Categories:
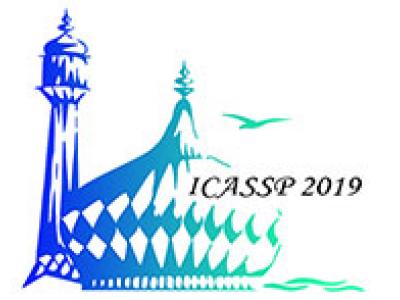
- Read more about Learning Shared Vector Representations of Lyrics and Chords in Music
- Log in to post comments
Music has a powerful influence on a listener's emotions. In this paper, we represent lyrics and chords in a shared vector space using a phrase-aligned chord-and-lyrics corpus. We show that models that use these shared representations predict a listener's emotion while hearing musical passages better than models that do not use these representations. Additionally, we conduct a visual analysis of these learnt shared vector representations and explain how they support existing theories in music.
- Categories:
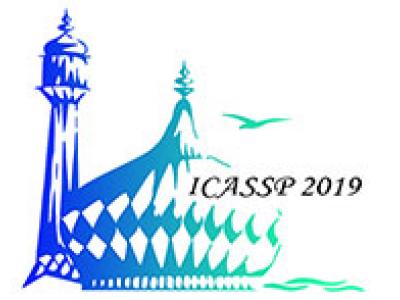
- Read more about Time domain Spherical harmonic analysis for adaptive noise cancellation over a spatial region
- Log in to post comments
Active Noise Cancellation (ANC) is a well researched topic for minimizing unwanted acoustic noise, and spatial ANC is a recently introduced concept that focuses on continuous spatial regions. Adaptive filter designing for spatial ANC is often based on frequency-domain spherical harmonic decomposition method, which has a major limitation due to the increased system latency. In this paper, we develop a time-domain spherical harmonic based signal decomposition method and use it to develop two time-space domain feed-forward adaptive filters for spatial ANC.
- Categories:
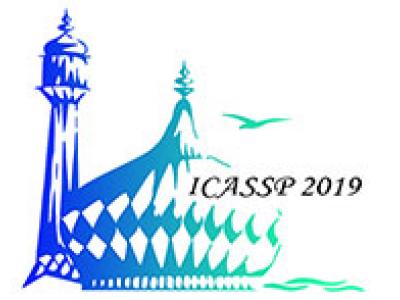
- Read more about A NEURAL NETWORK BASED RANKING FRAMEWORK TO IMPROVE ASR WITH NLU RELATED KNOWLEDGE DEPLOYED
- Log in to post comments
This work proposes a new neural network framework to simultaneously rank multiple hypotheses generated by one or more automatic speech recognition (ASR) engines for a speech utterance. Features fed in the framework not only include those calculated from the ASR information, but also involve natural language understanding (NLU) related features, such as trigger features capturing long-distance constraints between word/slot pairs and BLSTM features representing intent-sensitive sentence embedding.
- Categories:
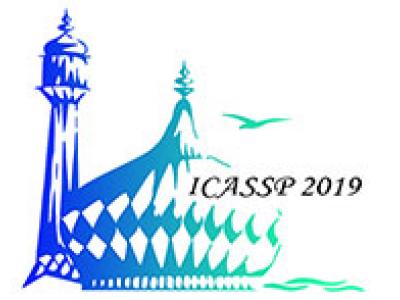
- Read more about Variational and Hierarchical Recurrent Autoencoder
- Log in to post comments
Despite a great success in learning representation for image data, it is challenging to learn the stochastic latent features from natural language based on variational inference. The difficulty in stochastic sequential learning is due to the posterior collapse caused by an autoregressive decoder which is prone to be too strong to learn sufficient latent information during optimization. To compensate this weakness in learning procedure, a sophisticated latent structure is required to assure good convergence so that random features are sufficiently captured for sequential decoding.
- Categories:
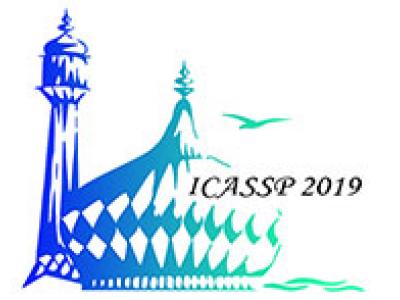
- Read more about COMPACT CONVOLUTIONAL RECURRENT NEURAL NETWORKS VIA BINARIZATION FOR SPEECH EMOTION RECOGNITION
- Log in to post comments
Despite the great advances, most of the recently developed automatic speech recognition systems focus on working in a server-client manner, and thus often require a high computational cost, such as the storage size and memory accesses. This, however, does not satisfy the increasing demand for a succinct model that can run smoothly in embedded devices like smartphones.
- Categories: