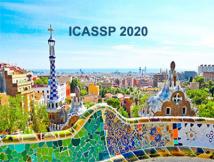
ICASSP is the world’s largest and most comprehensive technical conference focused on signal processing and its applications. The ICASSP 2020 conference will feature world-class presentations by internationally renowned speakers, cutting-edge session topics and provide a fantastic opportunity to network with like-minded professionals from around the world. Visit website.
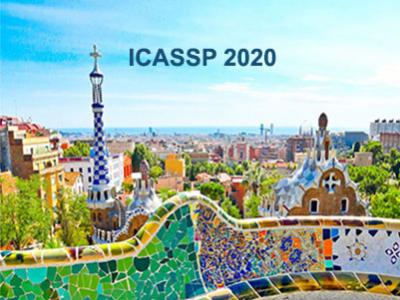
- Read more about Blind Multi-Spectral Image Pan-Sharpening
- Log in to post comments
We address the problem of sharpening low spatial-resolution multi-spectral (MS) images with their associated misaligned high spatial-resolution panchromatic (PAN) image, based on priors on the spatial blur kernel and on the cross-channel relationship. In particular, we formulate the blind pan-sharpening problem within a multi-convex optimization framework using total generalized variation for the blur kernel and local Laplacian prior for the cross-channel relationship.
- Categories:
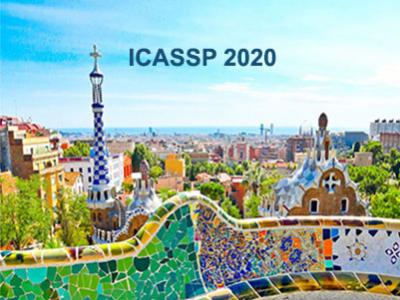
- Read more about Confidence Estimation for Black Box Automatic Speech Recognition Systems Using Lattice Recurrent Neural Networks
- Log in to post comments
Recently, there has been growth in providers of speech transcription services enabling others to leverage technology they would not normally be able to use. As a result, speech-enabled solutions have become commonplace. Their success critically relies on the quality, accuracy, and reliability of the underlying speech transcription systems. Those black box systems, however, offer limited means for quality control as only word sequences are typically available.
- Categories:
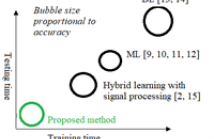
- Read more about INSTANT ADAPTIVE LEARNING: AN ADAPTIVE FILTER BASED FAST LEARNING MODEL CONSTRUCTION FOR SENSOR SIGNAL TIME SERIES CLASSIFICATION ON EDGE DEVICES
- Log in to post comments
Construction of learning model under computational and energy constraints, particularly in highly limited training time requirement is a critical as well as unique necessity of many practical IoT applications that use time series sensor signal analytics for edge devices. Yet, majority of the state-of-the-art algorithms and solutions attempt to achieve high performance objective (like test accuracy) irrespective of the computational constraints of real-life applications.
- Categories:
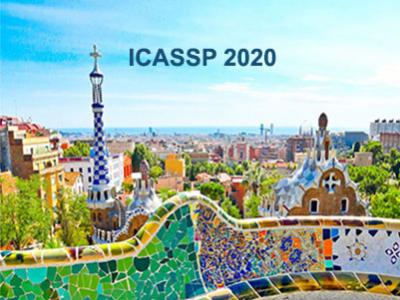
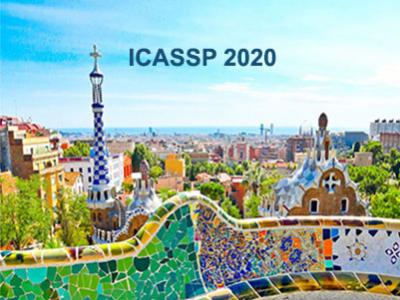
- Read more about A Time-Based Sampling Framework for Finite-Rate-of-Innovation Signals
- Log in to post comments
Time-based sampling of continuous-time signals is an alternate sampling paradigm in which the signal is encoded using a sequence of non-uniform instants time. The standard methods for reconstructing bandlimited and shift-invariant signals from their time-encoded measurements employ alternating projections type methods. In this paper, we consider the problem of sampling and perfect reconstruction of periodic finite-rate-of-innovation (FRI) signals using crossing time-encoding machine (C-TEM) and integrate-and-fire TEM (IF-TEM).
- Categories:
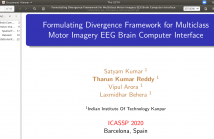
- Read more about FORMULATING DIVERGENCE FRAMEWORK FOR MULTICLASS MOTOR IMAGERY EEG BRAIN COMPUTER INTERFACE
- Log in to post comments
The ubiquitous presence of non-stationarities in the EEG signals significantly perturb the feature distribution thus deteriorating the performance of Brain Computer Interface. In this work, a novel method is proposed based on Joint Approximate Diagonalization (JAD) to optimize stationarity for multiclass motor imagery Brain Computer Interface (BCI) in an information theoretic framework. Specifically, in the proposed method, we estimate the subspace which optimizes the discriminability between the classes and simultaneously preserve stationarity within the motor imagery classes.
- Categories:
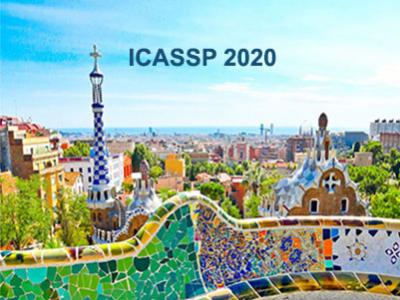
- Read more about Computing Hilbert Transform and Spectral Factorization for Signal Spaces of Smooth Functions
- Log in to post comments
Although the Hilbert transform and the spectral factorization are of central importance in signal processing,
- Categories:
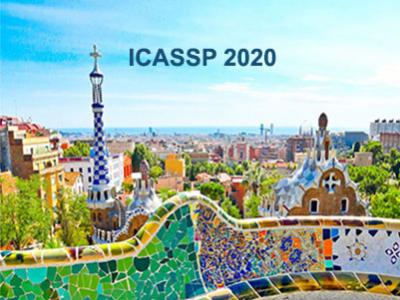
- Read more about FAST CLUSTERING WITH CO-CLUSTERING VIA DISCRETE NON-NEGATIVE MATRIX FACTORIZATION FOR IMAGE IDENTIFICATION
- Log in to post comments
- Categories:
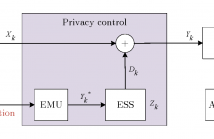
- Read more about On design of optimal smart meter privacy control strategy against adversarial MAP detection
- Log in to post comments
We study the optimal control problem of the maximum a posteriori (MAP) state sequence detection of an adversary using smart meter data. The privacy leakage is measured using the Bayesian risk and the privacy-enhancing control is achieved in real-time using an energy storage system. The control strategy is designed to minimize the expected performance of a non-causal adversary at each time instant. With a discrete-state Markov model, we study two detection problems: when the adversary is unaware or aware of the control.
- Categories:
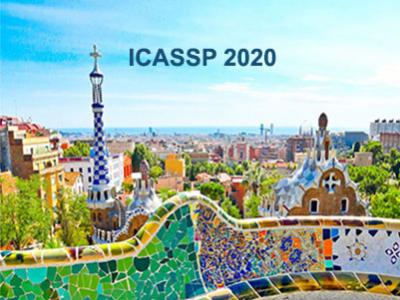
- Read more about An LSTM Based Architecture to Relate Speech Stimulus to EEG
- 1 comment
- Log in to post comments
icassp2020.pdf

- Categories: