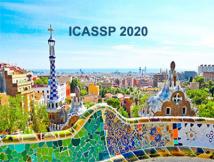
ICASSP is the world’s largest and most comprehensive technical conference focused on signal processing and its applications. The ICASSP 2020 conference will feature world-class presentations by internationally renowned speakers, cutting-edge session topics and provide a fantastic opportunity to network with like-minded professionals from around the world. Visit website.
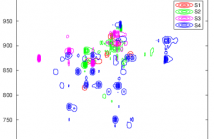
- Read more about β-NMF and sparsity promoting regularizations for complex mixture unmixing: Application to 2D HSQC NMR
- Log in to post comments
In Nuclear Magnetic Resonance (NMR) spectroscopy, an efficient analysis and a relevant extraction of different molecule properties from a given chemical mixture are important tasks, especially when processing bidimensional NMR data. To that end, using a blind source separation approach based on a variational formulation seems to be a good strategy. However, the poor resolution of NMR spectra and their large dimension require a new and modern blind source separation method.
- Categories:
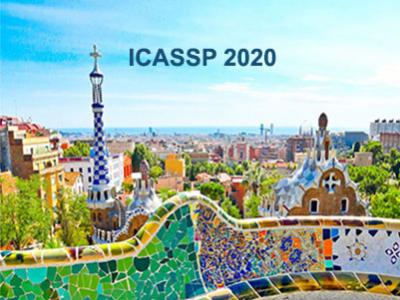
- Read more about BUT System for the Second DIHARD Speech Diarization Challenge
- Log in to post comments
This paper describes the winning systems developed by the BUT team for the four tracks of the Second DIHARD Speech Diarization Challenge. For tracks 1 and 2 the systems were mainly based on performing agglomerative hierarchical clustering (AHC) of x-vectors, followed by another x-vector clustering based on Bayes hidden Markov model and variational Bayes inference. We provide a comparison of the improvement given by each step and share the implementation of the core of the system.
- Categories:
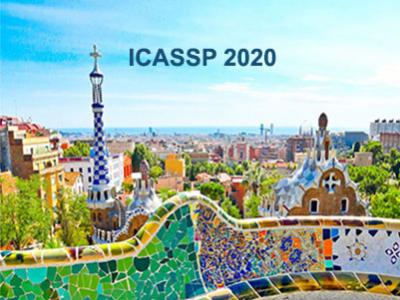
- Read more about FAST BLOCK-SPARSE ESTIMATION FOR VECTOR NETWORKS
- Log in to post comments
While there is now a significant literature on sparse inverse covariance estimation, all that literature, with only a couple of exceptions, has dealt only with univariate (or scalar) net- works where each node carries a univariate signal. However in many, perhaps most, applications, each node may carry multivariate signals representing multi-attribute data, possibly of different dimensions. Modelling such multivariate (or vector) networks requires fitting block-sparse inverse covariance matrices. Here we achieve maximal block sparsity by maximizing a block-l0-sparse penalized likelihood.
blockSpGGM.pdf

- Categories:
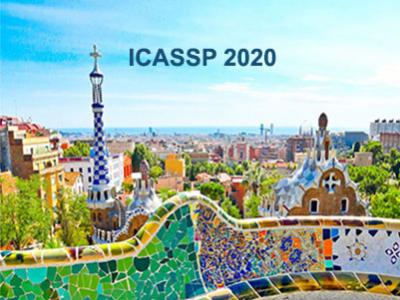
- Read more about LARGE-SCALE TIME SERIES CLUSTERING WITH k-ARs
- Log in to post comments
Time-series clustering involves grouping homogeneous time series together based on certain similarity measures. The mixture AR model (MxAR) has already been developed for time series clustering, as has an associated EM algorithm. How- ever, this EM clustering algorithm fails to perform satisfactorily in large-scale applications due to its high computational complexity. This paper proposes a new algorithm, k-ARs, which is a limiting version of the existing EM algorithm.
- Categories:
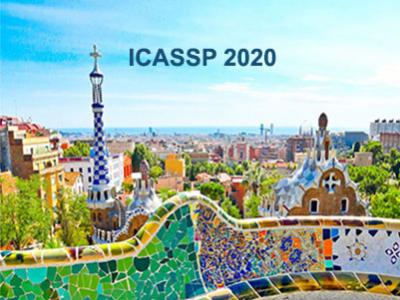
- Read more about DESIGN OF A CONVERGENCE-AWARE BASED EXPECTATION PROPAGATION ALGORITHM FOR UPLINK MIMO SCMA SYSTEMS
- Log in to post comments
Sparse code multiple access (SCMA) uses multi-dimensional sparse codewords to transmit user data. The expectation propagation algorithm (EPA) exploiting the sparse property shows linear complexity growth and thus is preferred for multi-user detection. To further reduce the complexity, a convergence-aware based EPA for uplink MIMO SCMA systems is proposed. Techniques including user termination, antenna termination, and codebook reduction are adopted. The user termination must be combined with the iteration constraint to avoid misjudgement.
- Categories:
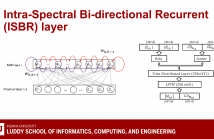
- Read more about Monaural Speech Enhancement Using Intra-Spectral Recurrent Layers In The Magnitude And Phase Responses
- Log in to post comments
Speech enhancement has greatly benefited from deep learning. Currently, the best performing deep architectures use long short-term memory (LSTM) recurrent neural networks (RNNs) to model short and long temporal dependencies. These approaches, however, underutilize or ignore spectral-level dependencies within the magnitude and phase responses, respectively. In this paper, we propose a deep learning architecture that leverages both temporal and spectral dependencies within the magnitude and phase responses.
- Categories:
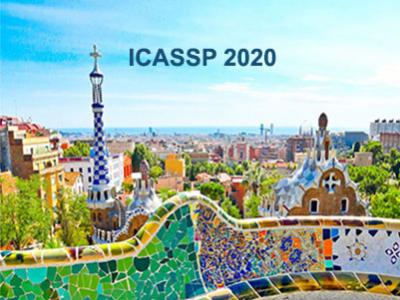
- Read more about TASK-AWARE MEAN TEACHER METHOD FOR LARGE SCALE WEAKLY LABELED SEMI-SUPERVISED SOUND EVENT DETECTION
- Log in to post comments
- Categories:
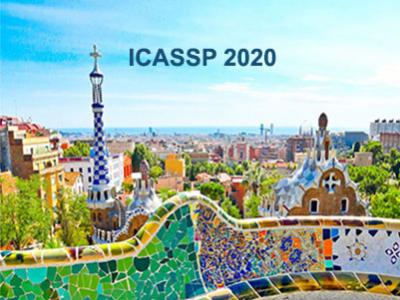
- Read more about Augmentation Data Synthesis via GANs: Boosting Latent Fingerprint Reconstruction
- Log in to post comments
Latent fingerprint reconstruction is a vital preprocessing step for its identification. This task is very challenging due to not only existing complicated degradation patterns but also its scarcity of paired training data. To address these challenges, we propose a novel generative adversarial network (GAN) based data augmentation scheme to improve such reconstruction.
ICASSP1263.pdf

- Categories:
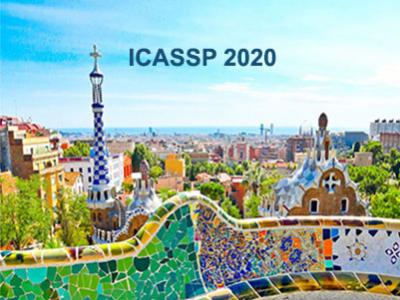
- Read more about Combining Acoustics, Content and Interaction Features to Find Hot Spots in Meetings
- Log in to post comments
Involvement hot spots have been proposed as a useful concept for meeting analysis and studied off and on for over 15 years. These are regions of meetings that are marked by high participant involvement, as judged by human annotators. However, prior work was either not conducted in a formal machine learning setting, or focused on only a subset of possible meeting features or downstream applications (such as summarization). In this paper we investigate to what extent various acoustic, linguistic and pragmatic aspects of the meetings, both in isolation and jointly, can help detect hot spots.
- Categories:
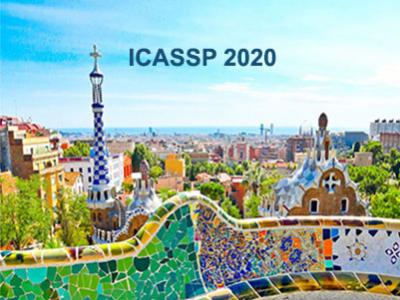
- Read more about Robust TDOA Indoor Tracking Using Constrained Measurement Filtering and Grid-Based Filtering
- Log in to post comments
- Categories: