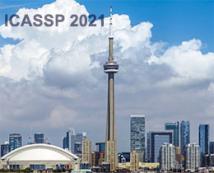
ICASSP 2021 - IEEE International Conference on Acoustics, Speech and Signal Processing is the world’s largest and most comprehensive technical conference focused on signal processing and its applications. The ICASSP 2021 conference will feature world-class presentations by internationally renowned speakers, cutting-edge session topics and provide a fantastic opportunity to network with like-minded professionals from around the world. Visit website.
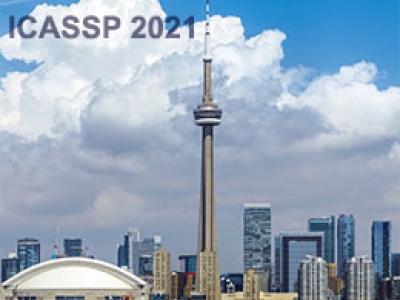
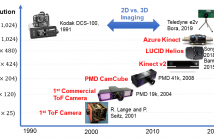
- Read more about Raw Data Processing for Practical Time-of-Flight Super-Resolution
- Log in to post comments
The relatively low resolution of Time-of-Flight (ToF) cameras, together with high power consumption and motion artifacts due to long exposure times, have kept ToF sensors away from classical lidar application fields, such as mobile robotics and autonomous driving. In this paper we note that while attempting to address the last two issues, e. g., via burst mode, the lateral resolution can be effectively increased. Differently from prior approaches, we propose a stripped-down modular super-resolution framework that operates in the raw data domain.
- Categories:
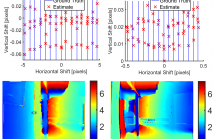
- Read more about Raw Data Processing for Practical Time-of-Flight Super-Resolution
- Log in to post comments
The relatively low resolution of Time-of-Flight (ToF) cameras, together with high power consumption and motion artifacts due to long exposure times, have kept ToF sensors away from classical lidar application fields, such as mobile robotics and autonomous driving. In this paper we note that while attempting to address the last two issues, e. g., via burst mode, the lateral resolution can be effectively increased. Differently from prior approaches, we propose a stripped-down modular super-resolution framework that operates in the raw data domain.
- Categories:
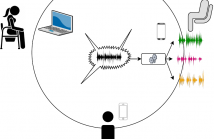
- Read more about DISTRIBUTED SPEECH SEPARATION IN SPATIALLY UNCONSTRAINED MICROPHONE ARRAYS
- Log in to post comments
- Categories:
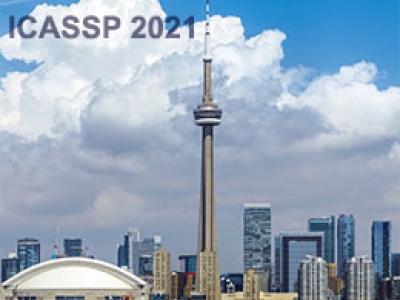
- Read more about CONTRASTIVE SEPARATIVE CODING FOR SELF-SUPERVISED REPRESENTATION LEARNING
- Log in to post comments
To extract robust deep representations from long sequential modeling of speech data, we propose a self-supervised learning approach, namely Contrastive Separative Coding (CSC). Our key finding is to learn such representations by separating the target signal from contrastive interfering signals.
- Categories:
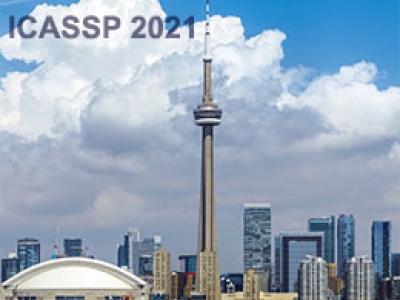
- Read more about ZERO-SHOT AUDIO CLASSIFICATION WITH FACTORED LINEAR AND NONLINEAR ACOUSTIC-SEMANTIC PROJECTIONS
- Log in to post comments
In this paper, we study zero-shot learning in audio classification through factored linear and nonlinear acoustic-semantic projections between audio instances and sound classes. Zero-shot learning in audio classification refers to classification problems that aim at recognizing audio instances of sound classes, which have no available training data but only semantic side information. In this paper, we address zero-shot learning by employing factored linear and nonlinear acoustic-semantic projections.
- Categories:
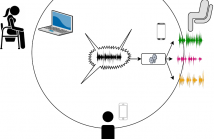
- Read more about DISTRIBUTED SPEECH SEPARATION IN SPATIALLY UNCONSTRAINED MICROPHONE ARRAYS
- 1 comment
- Log in to post comments
- Categories:
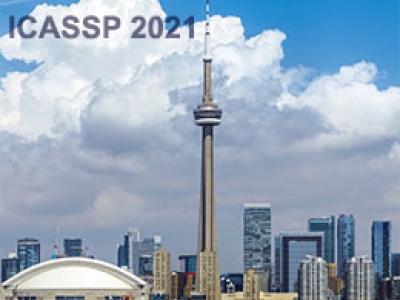
- Read more about Radar Clutter Classification Using Expectation-Maximization Method
- Log in to post comments
In this paper, the problem of classifying radar clutter returns into statistically homogeneous subsets is addressed. To this end, latent variables, which represent the classes to which the tested range cells belong, in conjunction with the expectation-maximization method are jointly exploited to devise the classification architecture. Moreover, two different models for the structure of the clutter covariance matrix are considered.
- Categories:
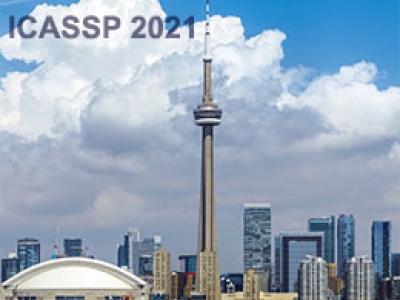
- Read more about FPGA HARDWARE DESIGN FOR PLENOPTIC 3D IMAGE PROCESSING ALGORITHM TARGETING A MOBILE APPLICATION
- Log in to post comments
- Categories:
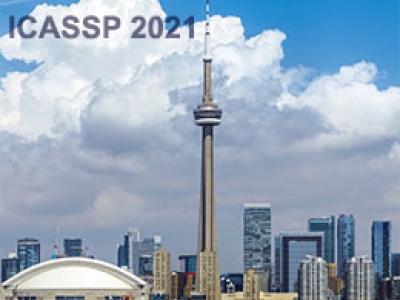
- Read more about FPGA HARDWARE DESIGN FOR PLENOPTIC 3D IMAGE PROCESSING ALGORITHM TARGETING A MOBILE APPLICATION
- Log in to post comments
- Categories: