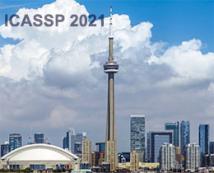
ICASSP 2021 - IEEE International Conference on Acoustics, Speech and Signal Processing is the world’s largest and most comprehensive technical conference focused on signal processing and its applications. The ICASSP 2021 conference will feature world-class presentations by internationally renowned speakers, cutting-edge session topics and provide a fantastic opportunity to network with like-minded professionals from around the world. Visit website.
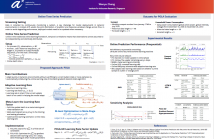
- Read more about POLA: Online Time Series Prediction by Adaptive Learning Rates
- Log in to post comments
Online prediction for streaming time series data has practical use for many real-world applications where downstream decisions depend on accurate forecasts for the future. Deployment in dynamic environments requires models to adapt quickly to changing data distributions without overfitting. We propose POLA (Predicting Online by Learning rate Adaptation) to automatically regulate the learning rate of recurrent neural network models to adapt to changing time series patterns across time.
POLA_slides.pdf

POLA_poster.pdf

- Categories:
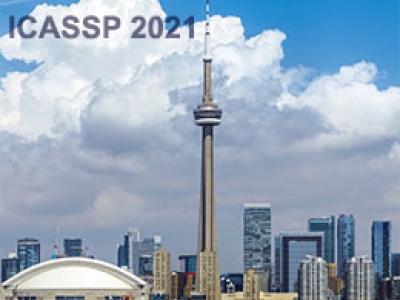
- Read more about FragmentVC: Any-to-Any Voice Conversion by End-to-End Extracting and Fusing Fine-Grained Voice Fragments With Attention
- Log in to post comments
Any-to-any voice conversion aims to convert the voice from and to any speakers even unseen during training, which is much more challenging compared to one-to-one or many-to-many tasks, but much more attractive in real-world scenarios. In this paper we proposed FragmentVC, in which the latent phonetic structure of the utterance from the source speaker is obtained from Wav2Vec 2.0, while the spectral features of the utterance(s) from the target speaker are obtained from log mel-spectrograms.
ICASSP_FragmentVC.pdf

FragmentVC.pdf

- Categories:
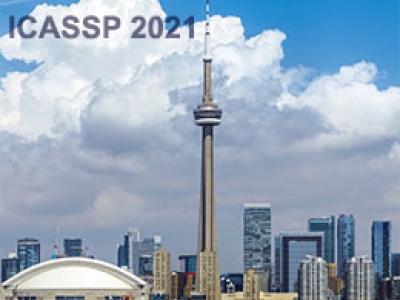
- Read more about Investigating on Incorporating Pretrained and Learnable Speaker Representations for Multi-Speaker Multi-Style Text-to-Speech
- Log in to post comments
The few-shot multi-speaker multi-style voice cloning task is to synthesize utterances with voice and speaking style similar to a reference speaker given only a few reference samples. In this work, we investigate different speaker representations and proposed to integrate pretrained and learnable speaker representations. Among different types of embeddings, the embedding pretrained by voice conversion achieves the best performance.
ICASSP_M2VoC.pdf

- Categories:
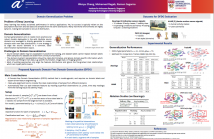
- Read more about Robust Domain-Free Domain Generalization with Class-Aware Alignment
- Log in to post comments
While deep neural networks demonstrate state-of-the-art performance on a variety of learning tasks, their performance relies on the assumption that train and test distributions are the same, which may not hold in real-world applications. Domain generalization addresses this issue by employing multiple source domains to build robust models that can generalize to unseen target domains subject to shifts in data distribution.
DFDG_slides.pdf

DFDG_poster.pdf

- Categories:
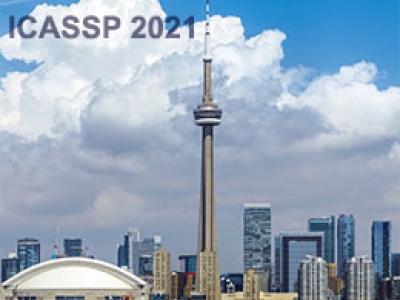
- Read more about Intermediate Loss Regularization for CTC-based Speech Recognition
- Log in to post comments
- Categories:
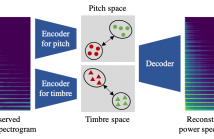
- Read more about Pitch-Timbre Disentanglement of Musical Instrument Sounds Based on VAE-Based Metric Learning
- Log in to post comments
This paper describes a representation learning method for disentangling an arbitrary musical instrument sound into latent pitch and timbre representations. Although such pitch-timbre disentanglement has been achieved with a variational autoencoder (VAE), especially for a predefined set of musical instruments, the latent pitch and timbre representations are outspread, making them hard to interpret.
- Categories:
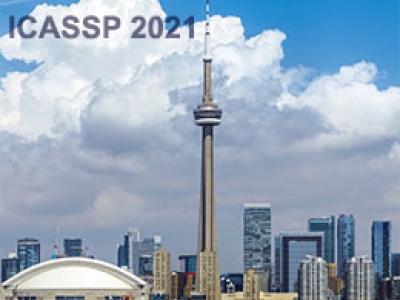
- Read more about Sparse time-frequency representation via atomic norm minimization
- Log in to post comments
- Categories:
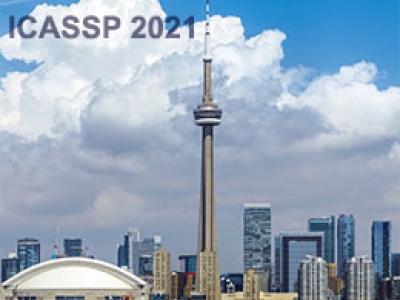
- Read more about MORE:A Metric-learning based Framework for Open-domain Relation Extraction
- Log in to post comments
Open relation extraction (OpenRE) is the task of extracting relation schemes from open-domain corpora. Most existing OpenRE methods either do not fully benefit from high-quality labeled corpora or can not learn semantic representation directly, affecting downstream clustering efficiency. To address these problems, in this work, we propose a novel learning framework named MORE (Metric learning-based Open Relation Extraction.
- Categories:
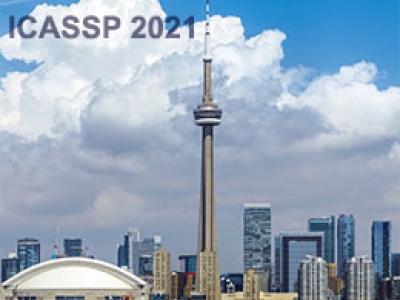
- Read more about Bridging Unpaired Facial Photos and Sketches by Line-drawings
- Log in to post comments
- Categories: