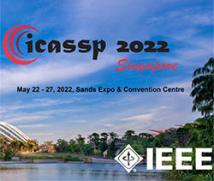
ICASSP 2022 - IEEE International Conference on Acoustics, Speech and Signal Processing is the world’s largest and most comprehensive technical conference focused on signal processing and its applications. The ICASSP 2022 conference will feature world-class presentations by internationally renowned speakers, cutting-edge session topics and provide a fantastic opportunity to network with like-minded professionals from around the world. Visit the website.
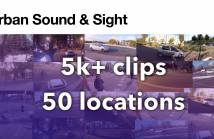
- Categories:
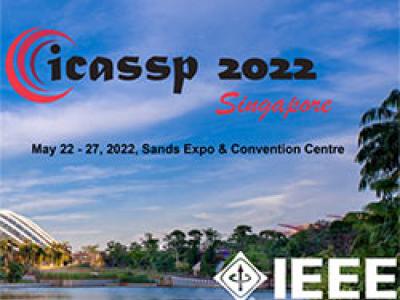
- Read more about One TTS Alignment To Rule Them All
- Log in to post comments
Speech-to-text alignment is a critical component of neural text-to-speech (TTS) models. Autoregressive TTS models typically use an attention mechanism to learn these alignments on-line. However, these alignments tend to be brittle and often fail to generalize to long utterances and out-of-domain text, leading to missing or repeating words. Most non-autoregressive end-to-end TTS models rely on durations extracted from external sources. In this paper we leverage the alignment mechanism proposed in RAD-TTS and demonstrate its applicability to wide variety of neural TTS models.
- Categories:
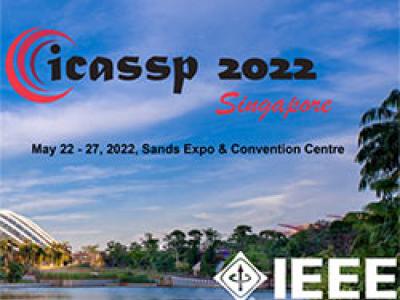
- Read more about Hand Gesture Recognition Using Temporal Convolutions and Attention Mechanism
- Log in to post comments
- Categories:
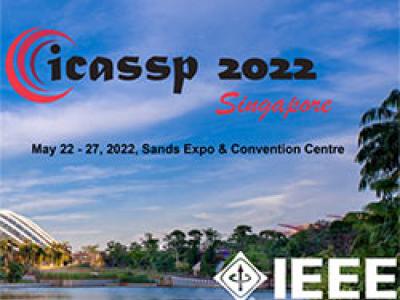
- Read more about SPATIO-TEMPORAL GRAPH CONVOLUTIONAL NETWORKS FOR CONTINUOUS SIGN LANGUAGE RECOGNITION
- Log in to post comments
ICASSP_poster_4541.pdf

- Categories:
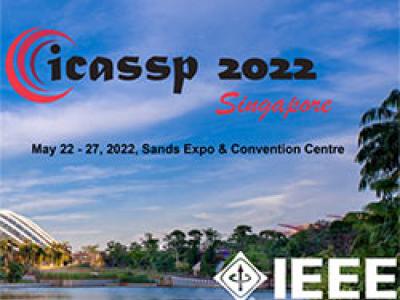
- Read more about Multiview Long-Short Spatial Contrastive Learning for 3D Medical Image Analysis
- Log in to post comments
The success of supervised deep learning heavily depends on large labeled datasets whose construction is often challenging in medical image analysis. Contrastive learning, a variant of self-supervised learning, is a potential solution to alleviate the strong demand for data annotation. In this work, we extend the contrastive learning framework to 3D volumetric medical imaging.
- Categories:
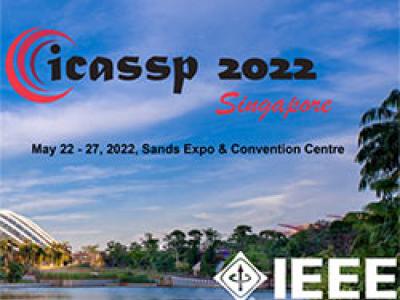
- Read more about Optimizing The Consumption Of Spiking Neural Networks With Activity Regularization
- Log in to post comments
- Categories:
- Read more about Optimizing The Consumption Of Spiking Neural Networks With Activity Regularization
- Log in to post comments
- Categories:
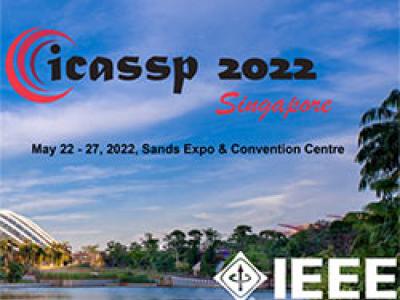
- Read more about PRIOR-BERT AND MULTI-TASK LEARNING FOR TARGET-ASPECT-SENTIMENT JOINT DETECTION
- Log in to post comments
Aspect-Based Sentiment Analysis (ABSA) is a fine-grained sentiment analysis task and has become a significant task with real-world scenario value. The challenge of this task is how to generate an effective text representation and construct an end-to-end model that can simultaneously detect (target, aspect, sentiment) triples from a sentence. Besides, the existing models do not take the heavily unbalanced distribution of labels into account and also do not give enough consideration to long-distance dependence of targets and aspect-sentiment pairs.
poster-new.pdf

- Categories:
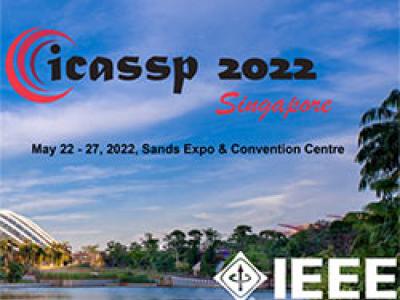
- Read more about PRIOR-BERT AND MULTITASK LEARNING FOR TARGET-ASPECT-SENTIMENT JOINT DETECTION
- Log in to post comments
Aspect-Based Sentiment Analysis (ABSA) is a fine-grained sentiment analysis task and has become a significant task with real-world scenario value. The challenge of this task is how to generate an effective text representation and construct an end-to-end model that can simultaneously detect (target, aspect, sentiment) triples from a sentence. Besides, the existing models do not take the heavily unbalanced distribution of labels into account and also do not give enough consideration to long-distance dependence of targets and aspect-sentiment pairs.
poster-new.pdf

- Categories:
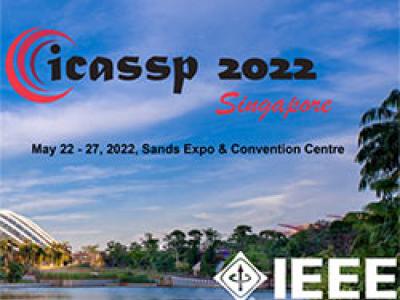
- Read more about NOVEL INSTANCE MINING WITH PSEUDO-MARGIN EVALUATION FOR FEW-SHOT OBJECT DETECTION
- Log in to post comments
Few-shot object detection (FSOD) enables the detector to recognize novel objects only using limited training samples, which could greatly alleviate model’s dependency on data. Most existing methods include two training stages, namely base training and fine-tuning. However, the unlabeled novel instances in the base set were untouched in previous works, which can be re-used to enhance the FSOD performance. Thus, a new instance mining model is proposed in this paper to excavate the novel samples from the base set. The detector is thus fine-tuned again by these additional free novel instances.
poster.pdf

- Categories: