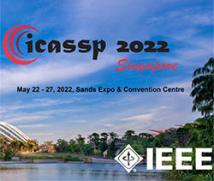
ICASSP 2022 - IEEE International Conference on Acoustics, Speech and Signal Processing is the world’s largest and most comprehensive technical conference focused on signal processing and its applications. The ICASSP 2022 conference will feature world-class presentations by internationally renowned speakers, cutting-edge session topics and provide a fantastic opportunity to network with like-minded professionals from around the world. Visit the website.
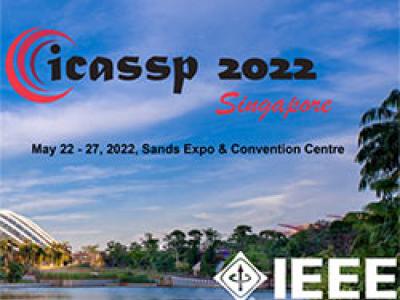
- Read more about Poster: ICASSP2022-2228: Cramer-Rao Bound for the Time-Varying Poisson
- Log in to post comments
Point processes are finding increasing applications in neuroscience, genomics, and social media. But basic modelling properties are little studied. Here we consider a periodic time-varying Poisson model and develop the asymptotic Cramer-Rao bound. We also develop, for the first time, a maximum likelihood algorithm for parameter estimation.
- Categories:
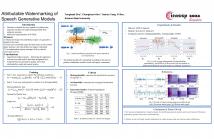
Generative models are now capable of synthesizing images, speeches, and videos that are hardly distinguishable from authentic contents. Such capabilities cause concerns such as malicious impersonation and IP theft. This paper investigates a solution for model attribution, i.e., the classification of synthetic contents by their source models via watermarks embedded in the contents.
- Categories:
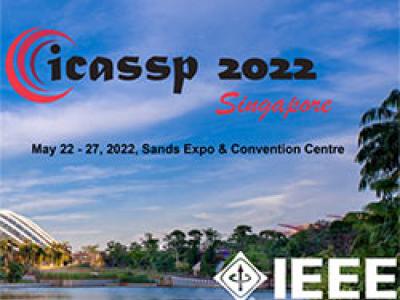
- Read more about SEGNET-BASED DEEP REPRESENTATION LEARNING FOR DYSPHAGIA CLASSIFICATION
- Log in to post comments
- Categories:
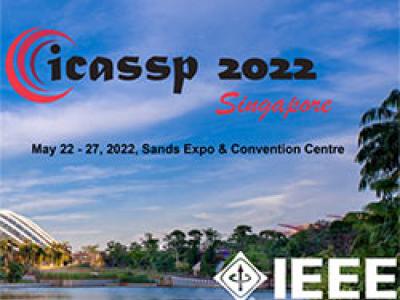
- Read more about MULTIMODAL EMOTION RECOGNITION WITH SURGICAL AND FABRIC MASKS
- Log in to post comments
In this study, we investigate how different types of masks affect automatic emotion classification in different channels of audio, visual, and multimodal. We train emotion classification models for each modality with the original data without mask and the re-generated data with mask respectively, and investigate how muffled speech and occluded facial expressions change the prediction of emotions.
- Categories:
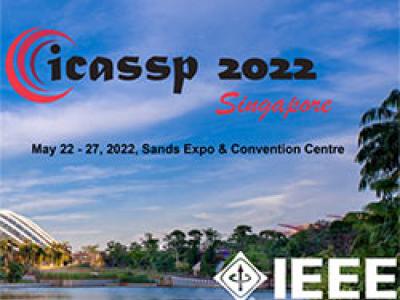
- Read more about ICASSP - Sequential MCMC methods for audio signal enhancement
- 1 comment
- Log in to post comments
With the aim of addressing audio signal restoration as a sequential inference problem, we build upon Gabor regression to propose a state-space model for audio time series. Exploiting the structure of our model, we devise a sequential Markov chain Monte Carlo algorithm to explore the sequence of filtering distributions of the synthesis coefficients. The algorithm is then tested on a series of denoising examples.
- Categories:
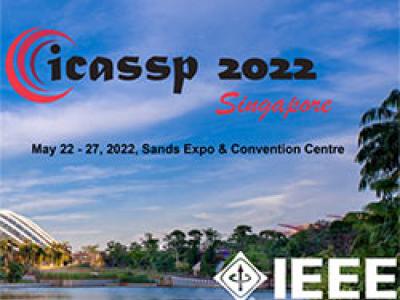
- Read more about SELF-KNOWLEDGE DISTILLATION BASED SELF-SUPERVISED LEARNING FOR COVID-19 DETECTION FROM CHEST X-RAY IMAGES
- Log in to post comments
1206-3.pdf

- Categories:
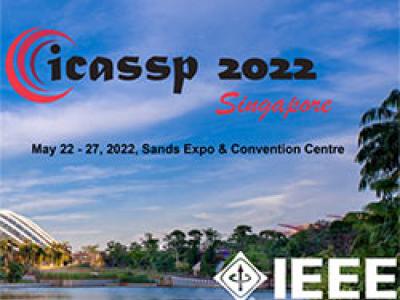
- Read more about TRIBYOL: TRIPLET BYOL FOR SELF-SUPERVISED REPRESENTATION LEARNING
- Log in to post comments
1917-1.pdf

- Categories:
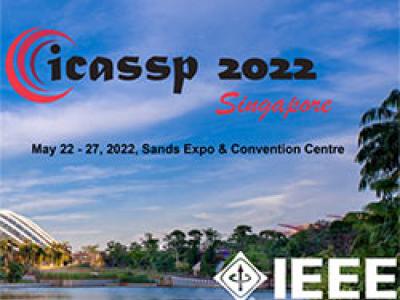
- Read more about FRAUG: A FRAME RATE BASED DATA AUGMENTATION METHOD FOR DEPRESSION DETECTION FROM SPEECH SIGNALS
- Log in to post comments
- Categories:
- Read more about Investigating the Potential of Auxiliary-Classifier GANs for Image Classification in Low Data Regimes
- Log in to post comments
Generative Adversarial Networks (GANs) have shown promise in augmenting datasets and boosting convolutional neural networks' (CNN) performance on image classification tasks. But they introduce more hyperparameters to tune as well as the need for additional time and computational power to train supplementary to the CNN. In this work, we examine the potential for Auxiliary-Classifier GANs (AC-GANs) as a 'one-stop-shop' architecture for image classification, particularly in low data regimes.
- Categories: