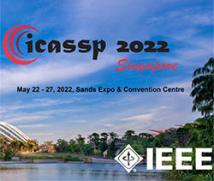
ICASSP 2022 - IEEE International Conference on Acoustics, Speech and Signal Processing is the world’s largest and most comprehensive technical conference focused on signal processing and its applications. The ICASSP 2022 conference will feature world-class presentations by internationally renowned speakers, cutting-edge session topics and provide a fantastic opportunity to network with like-minded professionals from around the world. Visit the website.
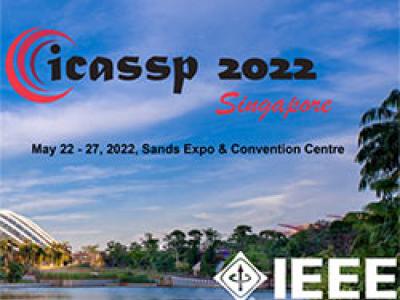
- Read more about TED TALK TEASER GENERATION WITH PRE-TRAINED MODELS
- Log in to post comments
While we have seen significant advances in automatic summarization for text, research on speech summarization is still limited. In this work, we address the challenge of automatically generating teasers for TED talks. In the first step, we create a corpus for automatic summarization of TED and TEDx talks consisting of the talks' recording, their transcripts and their descriptions. The corpus is used to build a speech summarization system for the task. We adapt and combine pre-trained models for automatic speech recognition (ASR) and text summarization using the collected data.
- Categories:
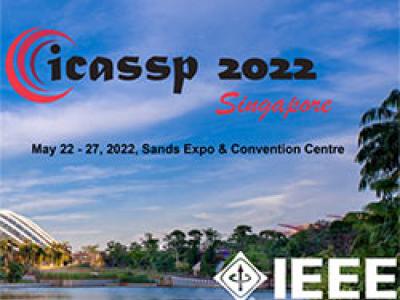
- Read more about LEARNING MONOCULAR 3D HUMAN POSE ESTIMATION WITH SKELETAL INTERPOLATION
- Log in to post comments
poster.pdf

- Categories:
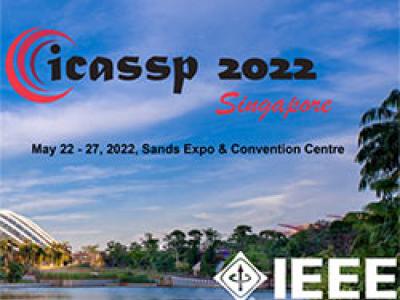
- Read more about 4D Convolutional Neural Networks for Multi Spectral and Multi Temporal Remote Sensing Data Classification
- Log in to post comments
Multi-temporal remotely sensed observations acquired by multi-spectral sensors contain a wealth of information related to the Earth’s state. Deep learning methods have demonstrated a great potential in analyzing such observations. Traditional 2D and 3D approaches are unable to effectively extract valuable information encoded across all available dimensions.
- Categories:
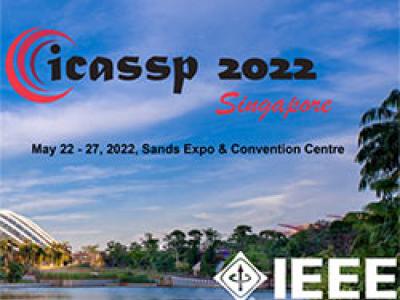
- Read more about A Novel Sequential Monte Carlo Framework For Predicting Ambiguous Emotion State (poster)
- Log in to post comments
- Categories:
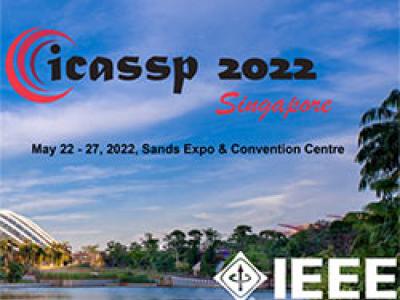
- Read more about A Novel Sequential Monte Carlo Framework For Predicting Ambiguous Emotion State (presentation slides)
- Log in to post comments
- Categories:
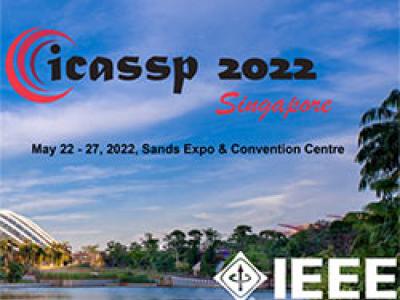
The recently proposed conformer architecture has been successfully used for end-to-end automatic speech recognition (ASR) architectures achieving state-of-the-art performance on different datasets. To our best knowledge, the impact of using conformer acoustic model for hybrid ASR is not investigated. In this paper, we present and evaluate a competitive conformer-based hybrid model training recipe. We study different training aspects and methods to improve worderror-rate as well as to increase training speed.
- Categories:
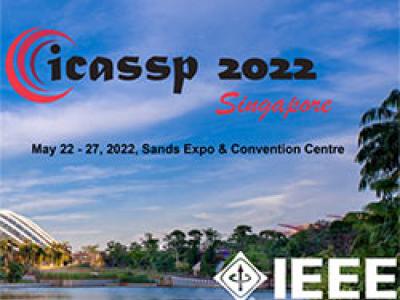
- Read more about Towards Transferable Speech Emotion Representation: On Loss Functions For Cross-Lingual Latent Representations
- Log in to post comments
In recent years, speech emotion recognition (SER) has been used in wide ranging applications, from healthcare to the commercial sector. In addition to signal processing approaches, methods for SER now also use deep learning techniques which provide transfer learning possibilities. However, generalizing over languages, corpora and recording conditions is still an open challenge. In this work we address this gap by exploring loss functions that aid in transferability, specifically to non-tonal languages.
- Categories:
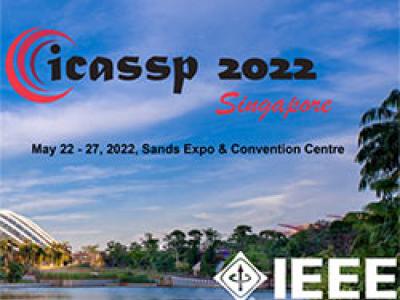
- Read more about AN ERROR CORRECTION SCHEME FOR IMPROVED AIR-TISSUE BOUNDARY IN REAL-TIME MRI VIDEO FOR SPEECH PRODUCTION
- Log in to post comments
The best performance in Air-tissue boundary (ATB) segmentation of real-time Magnetic Resonance Imaging (rtMRI) videos in speech production is known to be achieved by a 3-dimensional convolutional neural network (3D-CNN) model. However, the evaluation of this model, as well as other ATB segmentation techniques reported in the literature, is done using Dynamic Time Warping (DTW) distance between the entire original and predicted contours. Such an evaluation measure may not capture local errors in the predicted contour.
- Categories:
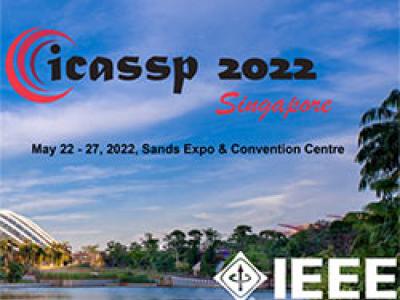
- Read more about End-to-End Neural Speech Coding for Real-Time Communications
- Log in to post comments
- Categories: