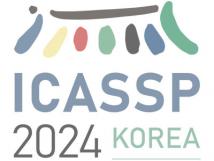
IEEE ICASSP 2024 - IEEE International Conference on Acoustics, Speech and Signal Processing (ICASSP) is the world’s largest and most comprehensive technical conference focused on signal processing and its applications. The IEEE ICASSP 2024 conference will feature world-class presentations by internationally renowned speakers, cutting-edge session topics and provide a fantastic opportunity to network with like-minded professionals from around the world. Visit the website.
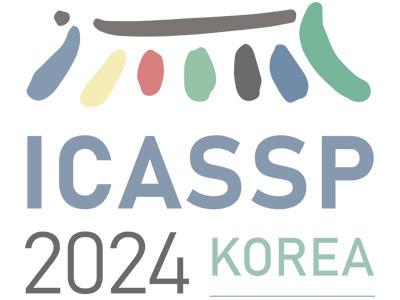
- Read more about END-TO-END SPEECH RECOGNITION CONTEXTUALIZATION WITH LARGE LANGUAGE MODELS
- Log in to post comments
In recent years, Large Language Models (LLMs) have garnered significant attention from the research community due to their exceptional performance and generalization capabilities. In this paper, we introduce a novel method for contextualizing speech recognition models incorporating LLMs. Our approach casts speech recognition as a mixed-modal language modeling task based on a pretrained LLM. We provide audio features, along with optional text tokens for context, to train the system to complete transcriptions in a decoderonly fashion.
- Categories:

- Read more about Presentation of Diffusion-based speech enhancement with a weighted generative-supervised learning loss
- 1 comment
- Log in to post comments
Diffusion-based generative models have recently gained attention in speech enhancement (SE), providing an alternative to conventional supervised methods. These models transform clean speech training samples into Gaussian noise, usually centered on noisy speech, and subsequently learn a parameterized
- Categories:
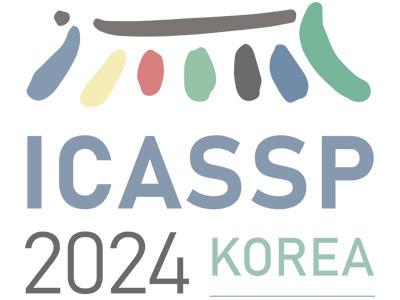
Inference of time-varying data over graphs is of importance in real-world applications such as urban water networks, economics, and brain recordings. It typically relies on identifying a computationally affordable joint spatiotemporal method that can leverage the patterns in the data. While this per se is a challenging task, it becomes even more so when the network comes with uncertainties, which, if not accounted for, can lead to unpredictable consequences.
- Categories:
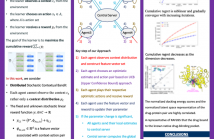
- Read more about DISTRIBUTED STOCHASTIC CONTEXTUAL BANDITS FOR PROTEIN DRUG INTERACTION
- 1 comment
- Log in to post comments
In recent work [1], we developed a distributed stochastic multi-arm contextual bandit algorithm to learn optimal actions when the contexts are unknown, and M agents work collaboratively under the coordination of a central server to minimize the total regret. In our model, the agents observe only the context distribution and the exact context is unknown to the agents. Such a situation arises, for instance, when the context itself is a noisy measurement or based on a prediction mechanism.
- Categories:
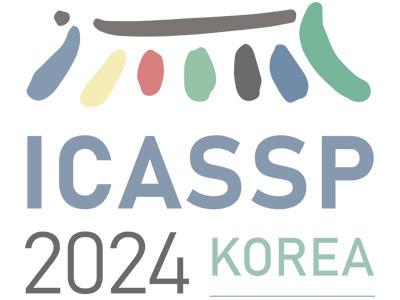
- Read more about Object Trajectory Estimation with Multi-Band Wi-Fi Neural Dynamic Fusion
- Log in to post comments
In contrast to existing multi-band Wi-Fi fusion in a frame-to-frame basis for simple classification, this paper considers asynchronous sequence-to-sequence fusion between sub-7GHz channel state information (CSI) and 60GHz beam SNR for more challenging downstream tasks such as continuous regression.
- Categories:
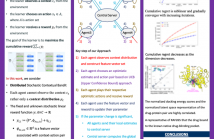
- Read more about DISTRIBUTED STOCHASTIC CONTEXTUAL BANDITS FOR PROTEIN DRUG INTERACTION
- Log in to post comments
In recent work [1], we developed a distributed stochastic multi-arm contextual bandit algorithm to learn optimal actions when the contexts are unknown, and M agents work collaboratively under the coordination of a central server to minimize the total regret. In our model, the agents observe only the context distribution and the exact context is unknown to the agents. Such a situation arises, for instance, when the context itself is a noisy measurement or based on a prediction mechanism.
- Categories:

- Read more about MaskMark: Robust Neural Watermarking for Real and Synthetic Speech (Slides)
- 1 comment
- Log in to post comments
High-quality speech synthesis models may be used to spread misinformation or impersonate voices. Audio watermarking can help combat such misuses by embedding a traceable signature in generated audio. However, existing audio watermarks are not designed for synthetic speech and typically demonstrate robustness to only a small set of transformations of the watermarked audio. To address this, we propose MaskMark, a neural network-based digital audio watermarking technique optimized for speech.
- Categories:
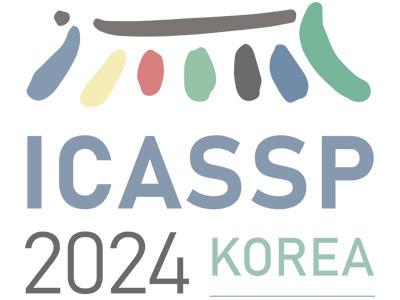
- Read more about [Poster] Selective Acoustic Feature Enhancement for Speech Emotion Recognition with Noisy Speech
- Log in to post comments
A speech emotion recognition (SER) system deployed on a real-world application can encounter speech contaminated with unconstrained background noise. To deal with this issue,
- Categories:
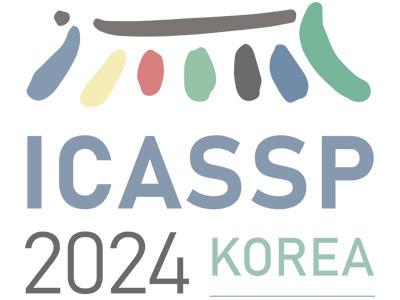
- Read more about A Lightweight Hybrid Multi-Channel Speech Extraction System with Directional Voice Activity Detection
- Log in to post comments
Although deep learning (DL) based end-to-end models have shown outstanding performance in multi-channel speech extraction, their practical applications on edge devices are restricted due to their high computational complexity. In this paper, we propose a hybrid system that can more effectively integrate the generalized sidelobe canceller (GSC) and a lightweight post-filtering model under the assistance of spatial speaker activity information provided by a directional voice activity detection (DVAD) module.
- Categories:
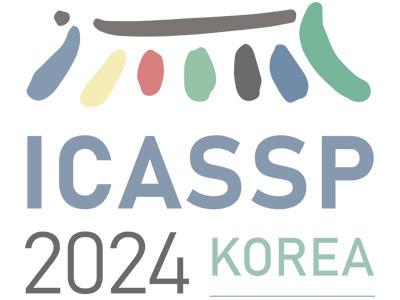
- Read more about Poster for ICASSP 2024 paper "Hot-Fixing Wake Work Recognition for End-to-End ASR via Neural Model Reprogramming"
- Log in to post comments
This paper proposes two novel variants of neural reprogramming to enhance wake word recognition in streaming end-to-end ASR models without updating model weights. The first, "trigger-frame reprogramming", prepends the input speech feature sequence with the learned trigger-frames of the target wake word to adjust ASR model’s hidden states for improved wake word recognition. The second, "predictor-state initialization", trains only the initial state vectors (cell and hidden states) of the LSTMs in the prediction network.
- Categories: