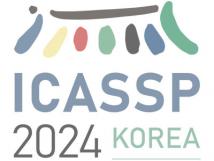
IEEE ICASSP 2024 - IEEE International Conference on Acoustics, Speech and Signal Processing (ICASSP) is the world’s largest and most comprehensive technical conference focused on signal processing and its applications. The IEEE ICASSP 2024 conference will feature world-class presentations by internationally renowned speakers, cutting-edge session topics and provide a fantastic opportunity to network with like-minded professionals from around the world. Visit the website.
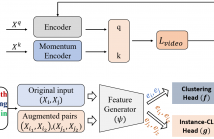
- Read more about ICASSP2024-Paper ID 3371-IVMSP-L2.4: VT-REID: LEARNING DISCRIMINATIVE VISUAL-TEXT REPRESENTATION FOR POLYP RE-IDENTIFICATION
- Log in to post comments
Presention Slides in ICASSP2024 for IVMSP-L2.4: VT-REID: LEARNING DISCRIMINATIVE VISUAL-TEXT REPRESENTATION FOR POLYP RE-IDENTIFICATION
- Categories:
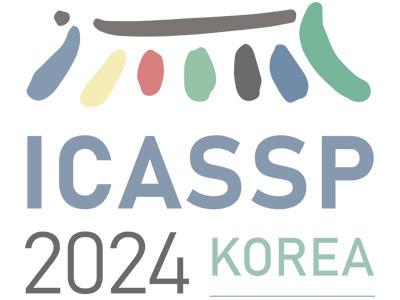
- Read more about Poster for IMAGE ATTRIBUTION BY GENERATING IMAGES
- Log in to post comments
We introduce GPNN-CAM, a novel method for CNN explanation, that bridges two distinct areas of computer vision:
Image Attribution, which aims to explain a predictor by highlighting image regions it finds important, and Single
Image Generation (SIG), that focuses on learning how to generate variations of a single sample. GPNN-CAM leverages samples generated by Generative
- Categories:
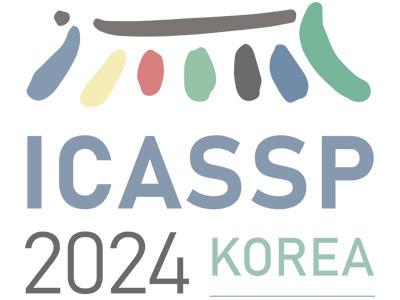
- Read more about Partially observable model-based learning for ISAC resource allocation
- Log in to post comments
This paper considers resource allocation problems for integrated sensing and communications (ISAC) systems operating in dynamic shared spectrum scenarios. Specifically, the paper proposes a new Model-Based Online Learning (MBOL) method that accounts for partial observability caused by noisy observations. First, the approach converts the partially observable Markov decision process (POMDP) to the equivalent belief state Markov decision process (MDP). Then, the state prediction model is learned from the sensor observations.
- Categories:
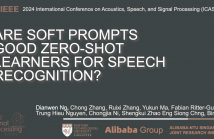
- Read more about Are Soft Prompts Good Zero-shot Learners for Speech Recognition?
- Log in to post comments
Large self-supervised pre-trained speech models require computationally expensive fine-tuning for downstream tasks. Soft prompt tuning offers a simple parameter-efficient alternative by utilizing minimal soft prompt guidance, enhancing portability while also maintaining competitive performance. However, not many people understand how and why this is so. In this study, we aim to deepen our understanding of this emerging method by investigating the role of soft prompts in automatic speech recognition (ASR).
- Categories:
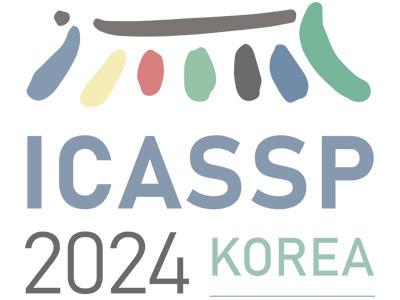
- Read more about Speech Emotion Recognition with Distilled Prosodic and Linguistic Affect Representations
- Log in to post comments
We propose EmoDistill, a novel speech emotion recognition (SER) framework that leverages cross-modal knowledge distillation during training to learn strong linguistic and prosodic representations of emotion from speech. During inference, our method only uses a stream of speech signals to perform unimodal SER thus reducing computation overhead and avoiding run-time transcription and prosodic feature extraction errors. During training, our method distills information at both embedding and logit levels from a pair of pre-trained Prosodic and Linguistic teachers that are fine-tuned for SER.
- Categories:
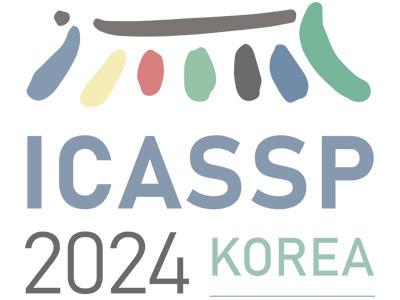
- Read more about A UNIFIED DNN-BASED SYSTEM FOR INDUSTRIAL PIPELINE SEGMENTATION
- Log in to post comments
This paper presents a unified system tailored for autonomous pipe segmentation within an industrial setting. To this end, it is designed to analyze RGB images captured by Unmanned Aerial Vehicle (UAV)-mounted cameras to predict binary pipe segmentation maps.
- Categories:
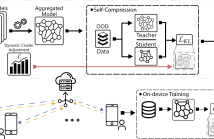
- Read more about Communication-Efficient Federated Learning through Adaptive Weight Clustering and Server-Side Distillation
- Log in to post comments
Federated Learning (FL) is a promising technique for the collaborative training of deep neural networks across multiple devices while preserving data privacy. Despite its potential benefits, FL is hindered by excessive communication costs due to repeated server-client communication during training.
2401.14211.pdf

- Categories:
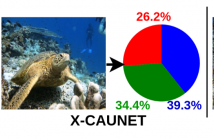
- Read more about X-CAUNET: CROSS-COLOR CHANNEL ATTENTION WITH UNDERWATER IMAGE-ENHANCING TRANSFORMER
- Log in to post comments
Underwater image enhancement is essential to mitigate the environment-centric noise in images, such as haziness, color degradation, etc. With most existing works focused on processing an RGB image as a whole, the explicit context that can be mined from each color channel separately goes unaccounted for, ignoring the effects produced by the wavelength of light in underwater conditions. In this work, we propose a framework called X-CAUNET that addresses this
- Categories:
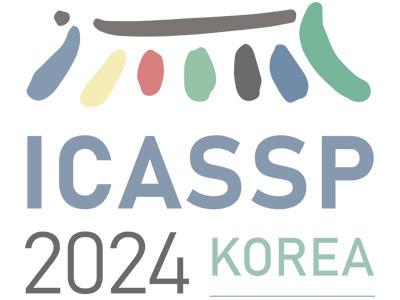
- Read more about ROTOR NOISE-AWARE NOISE COVARIANCE MATRIX ESTIMATION FOR UNMANNED AERIAL VEHICLE AUDITION
- Log in to post comments
A noise covariance matrix (NCM) estimation method for unmanned aerial vehicle (UAV) audition is proposed with rotor noise reduction as its primary focus. The proposed NCM estimation method could be incorporated into audio processing algorithms using UAV-mounted microphone array systems.
- Categories:
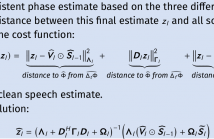
- Read more about PHASE RECONSTRUCTION IN SINGLE CHANNEL SPEECH ENHANCEMENT BASED ON PHASE GRADIENTS AND ESTIMATED CLEAN-SPEECH AMPLITUDES
- Log in to post comments
Phase gradients can help enforce phase consistency across time and frequency, further improving the output of speech enhancement approaches. Recently, neural networks were used to estimate the phase gradients from the short-term amplitude spectra of clean speech. These were then used to synthesise phase to reconstruct a plausible time-domain signal. However, using purely synthetic phase in speech enhancement yields unnatural-sounding output. Therefore we derive a closed-form phase estimate that combines the synthetic phase with that of the enhanced speech, yielding more natural output.
- Categories: